Denoiser-guided image deconvolution with arbitrary boundaries and incomplete observations
SIGNAL PROCESSING(2024)
摘要
Non-blind image deconvolution (NBID) has been a significant and long-standing research topic in the field of imaging sciences. While enduring efforts have been made over the past decades, they are extensively focusing on the scenario where the blur process is artificially assumed, e.g., typically under periodic boundary condition, as the involved matrix inversion can be efficiently tackled using the fast Fourier transforms (FFTs). However, despite its popularity, these boundary conditions are not always appropriate and can lead to severe artifacts in the recovered image in practice. Therefore, image deconvolution with arbitrary boundaries remains as a fundamental problem to be investigated. In this paper, we propose a principled framework with the aid of Plug -and-Play (PnP) scheme to address NBID in the more realistic scenarios of unknown boundaries. Specifically, the local and nonlocal denoiser priors with implicit optimization objectives are incorporated into a unified model, and the degradation operator is formulated as a composition of a convolution (under periodic boundary condition) and a spatial mask that only keeps those pixels which do not depend on the unknown boundary. Two kinds of experiments: deconvolution, and deconvolution with inpainting, both with unknown boundaries are tested. Both quantitative and qualitative evaluations demonstrate that the proposed denoiser-guided PnP framework is highly flexible and effective to cope with the general image deconvolution tasks.
更多查看译文
关键词
Non-blind image deconvolution,Arbitrary boundary conditions,Incomplete observations,Denoiser prior,Plug-and-play ADMM
AI 理解论文
溯源树
样例
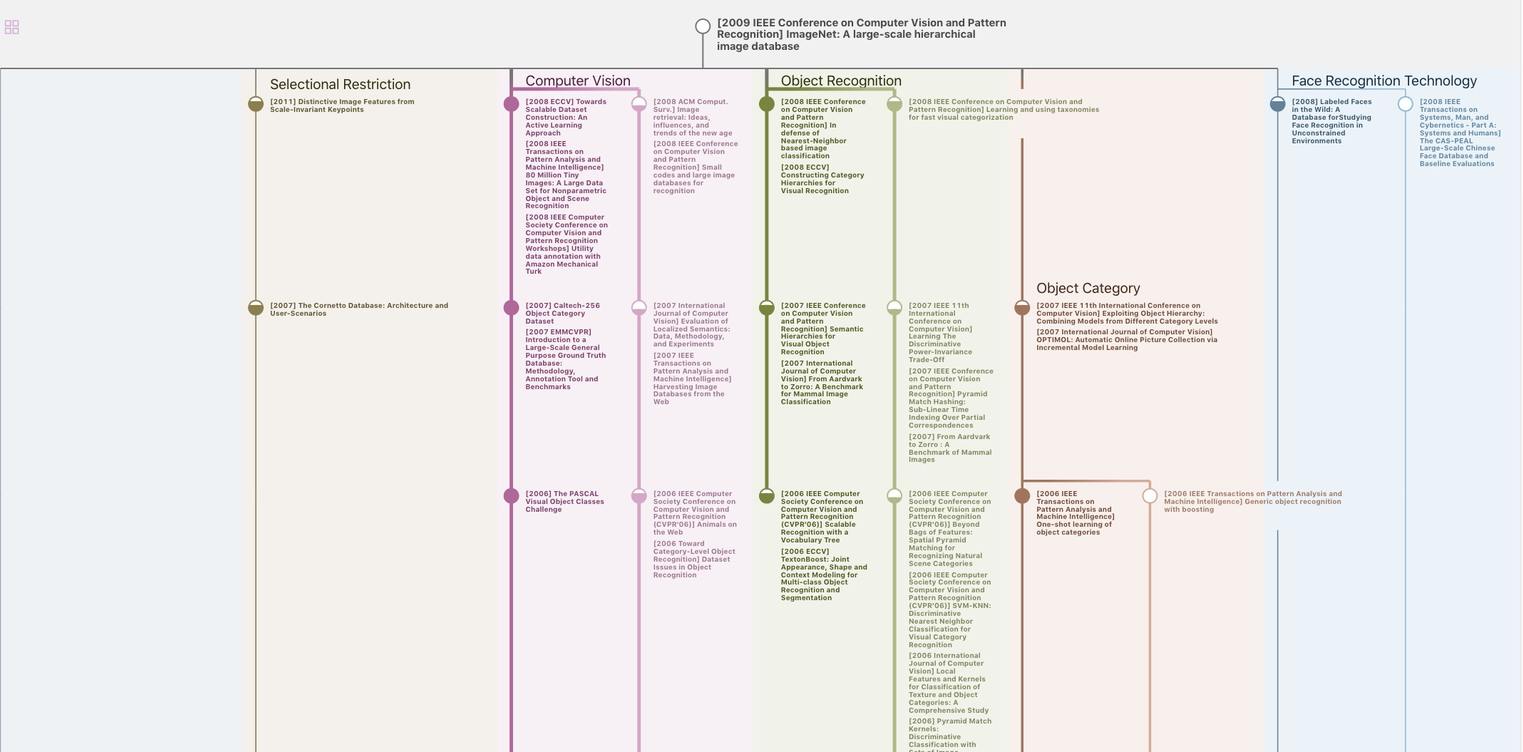
生成溯源树,研究论文发展脉络
Chat Paper
正在生成论文摘要