A Marginal Distributionally Robust MMSE Estimation for a Multisensor System With Kullback-Leibler Divergence Constraints.
IEEE Trans. Signal Process.(2023)
摘要
In this paper, we study a novel marginal distributionally robust minimum mean-squared error (MDR-MMSE) estimation problem for a random state vector in a multisensor system consisting of several sensors linked with a fusion center by employing the minimax viewpoint, which involves finding the estimator with the best performance under the least favorable distribution within an uncertainty set. In contrast to previous studies, the uncertainty set includes a family of densities whose marginal densities are located in a neighborhood defined by placing a threshold on the Kullback-Leibler (KL) divergence between actual and nominal Gaussian marginal densities, which is suitable for the scenario of the multisensor system. Despite the infinite-dimensional optimization nature of the MDR-MMSE estimation problem, we prove that there exists an affine estimator and a corresponding Gaussian distribution constituting its saddle point solution, which can be obtained by solving a finite-dimensional convex optimization problem. In addition, the “partially affine” and “partially Gaussian” properties are also analysed for all saddle point solutions. Moreover, we proved that the optimal estimator for a reduced MDR-MMSE estimation problem with all inactive sensors removed is also optimal for the original problem with all sensors remaining, thus reducing the communication burden of sending the observations from inactive sensors to the fusion center. Additionally, three distinct criteria for evaluating the relationships between the active sensors and the thresholds are established. Numerical experiments are also provided to verify the superiority of our proposed model.
更多查看译文
关键词
Robust MMSE estimation, Kullback-Leibler (KL) divergence, minimax problem
AI 理解论文
溯源树
样例
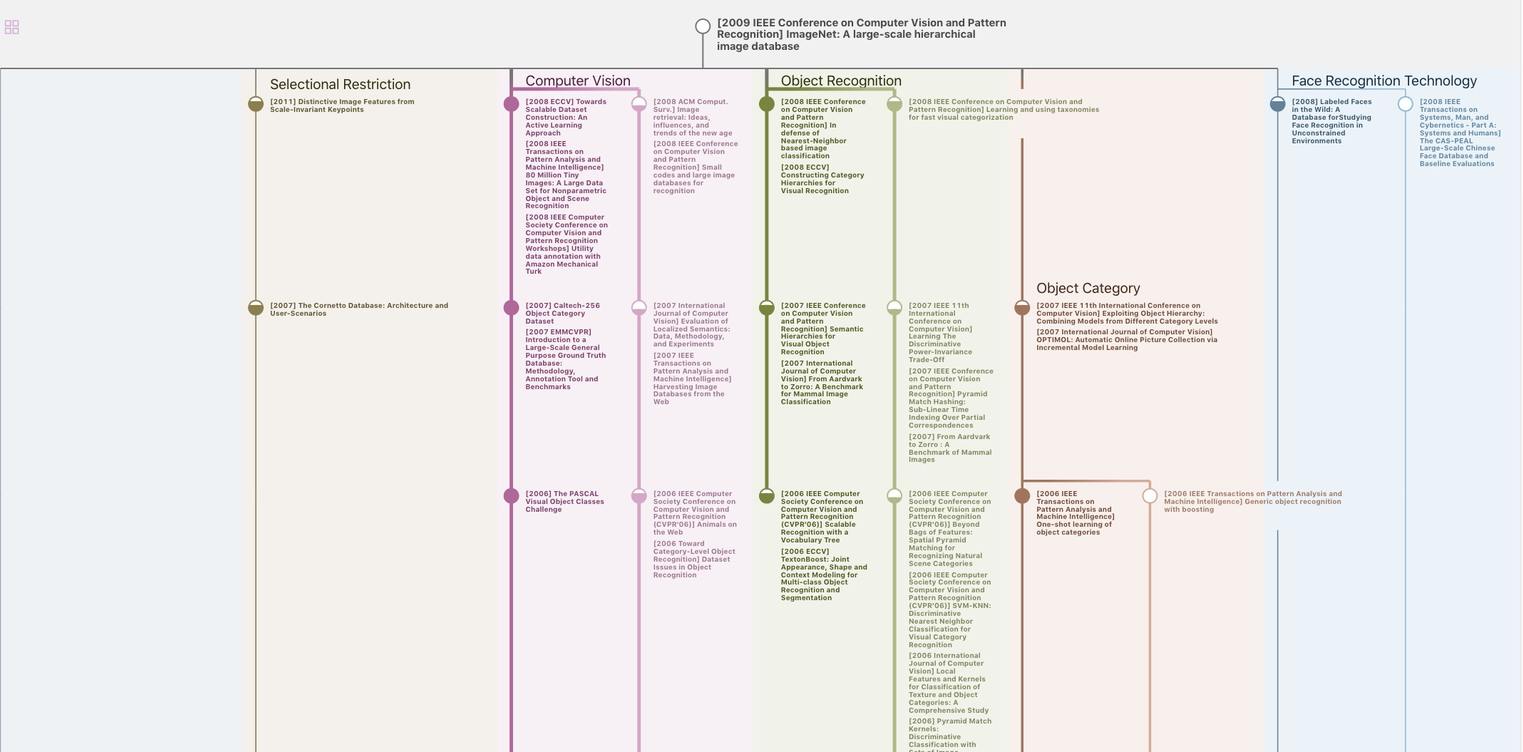
生成溯源树,研究论文发展脉络
Chat Paper
正在生成论文摘要