Reinforcement learning based routing for time-aware shaper scheduling in time-sensitive networks
COMPUTER NETWORKS(2023)
摘要
To guarantee real-time performance and quality-of-service (QoS) of time-critical industrial systems, time-aware shaper (TAS) in time-sensitive networking (TSN) controls frame transmission times in a bridged network using a scheduled gate control mechanism. However, most TAS scheduling methods generate schedules based on pre-configured routes without exploring alternatives for better schedulability, and methods that jointly consider routing and scheduling require enormous runtime and computing resources. To address this problem, we propose a TSN Scheduler with Reinforcement Learning-based Routing (TSLR) that identifies improved load balanced routes for higher schedulability with acceptable complexity using distributional reinforcement learning. We evaluate TSLR through TSN simulations and compare it against state-of-the-art algorithms to demonstrate that TSLR effectively improves TAS schedulability and link utilization in TSN with lower complexity. Specifically, TSLR shows a more than 66% increase in schedulability compared to the other algorithms, and TSLR's scheduling time is reduced by more than 1 h. It also shows flows' transmission latency is less than 25% of their latency deadline requirement and reduces maximum link utilization by approximately 50%.
更多查看译文
关键词
Time-Aware Shaper (TAS),Time-Sensitive Network (TSN),Reinforcement learning,Routing,Scheduling,Network simulation,Network performance evaluation
AI 理解论文
溯源树
样例
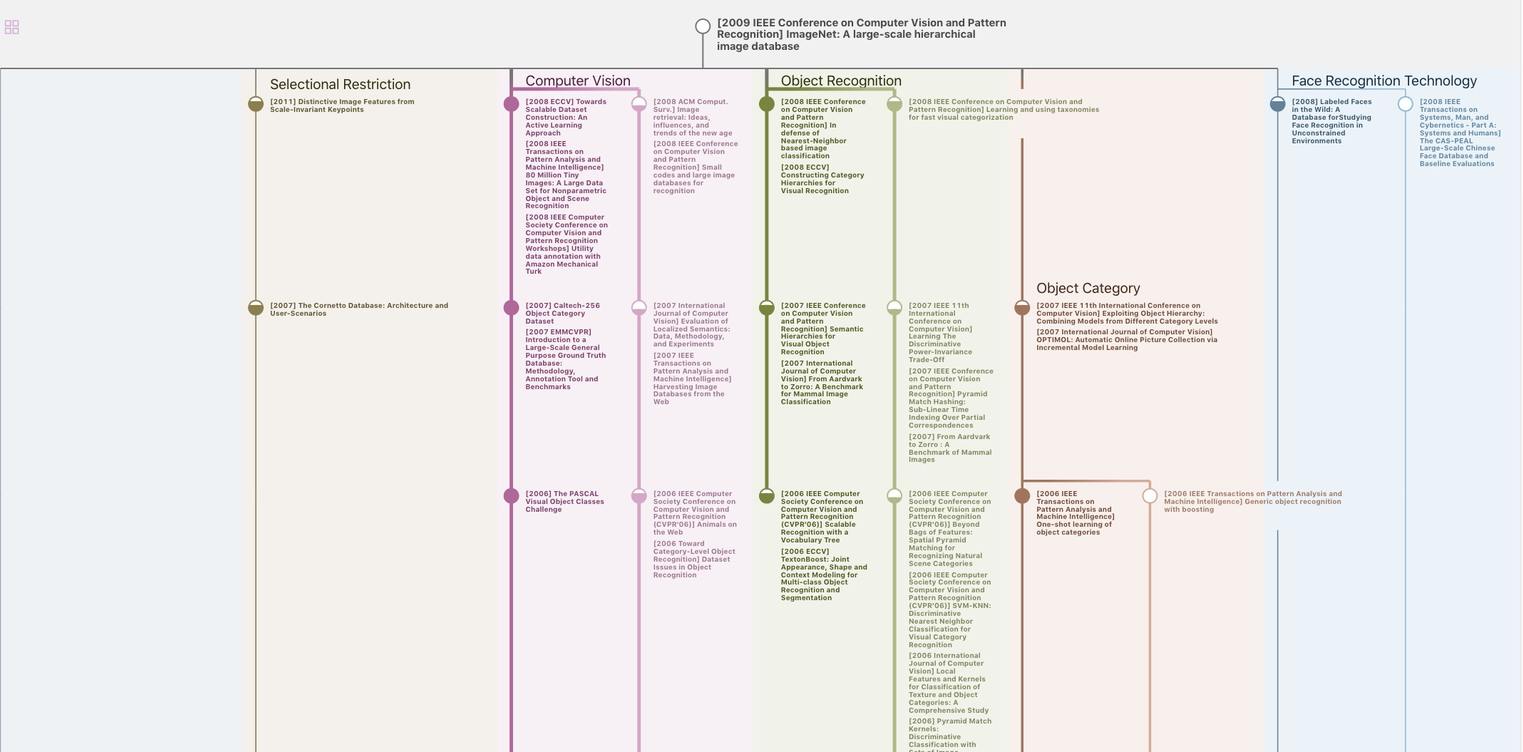
生成溯源树,研究论文发展脉络
Chat Paper
正在生成论文摘要