Causal Effect Estimation under Interference on Hypergraphs.
AI Matters(2023)
摘要
Hypergraphs offer a powerful abstraction for representing multi-way group interactions, allowing hyperedges to connect any number of nodes. In contrast to prevailing approaches that focus on capturing statistical dependencies, our research explores hypergraphs from a causal perspective. Specifically, we tackle the problem of estimating individual treatment effects (ITE) on hypergraphs, aiming to determine the causal impact of interventions (e.g., wearing face covering) on outcomes (e.g., COVID-19 infection) for each individual node. Existing ITE estimation methods either assume no interference between individuals or consider interference only among connected individuals in regular graphs. However, such assumptions may not hold in real-world hypergraphs. Recognizing this, we propose a novel causality learning framework HyperSCI by modeling high-order interference on hyper-graphs. Through extensive experiments on real-world hypergraphs, we validate the effectiveness of HyperSCI and highlight the potential of causal inference in hypergraphs with complex group interactions. 1
更多查看译文
关键词
causal effect estimation,interference
AI 理解论文
溯源树
样例
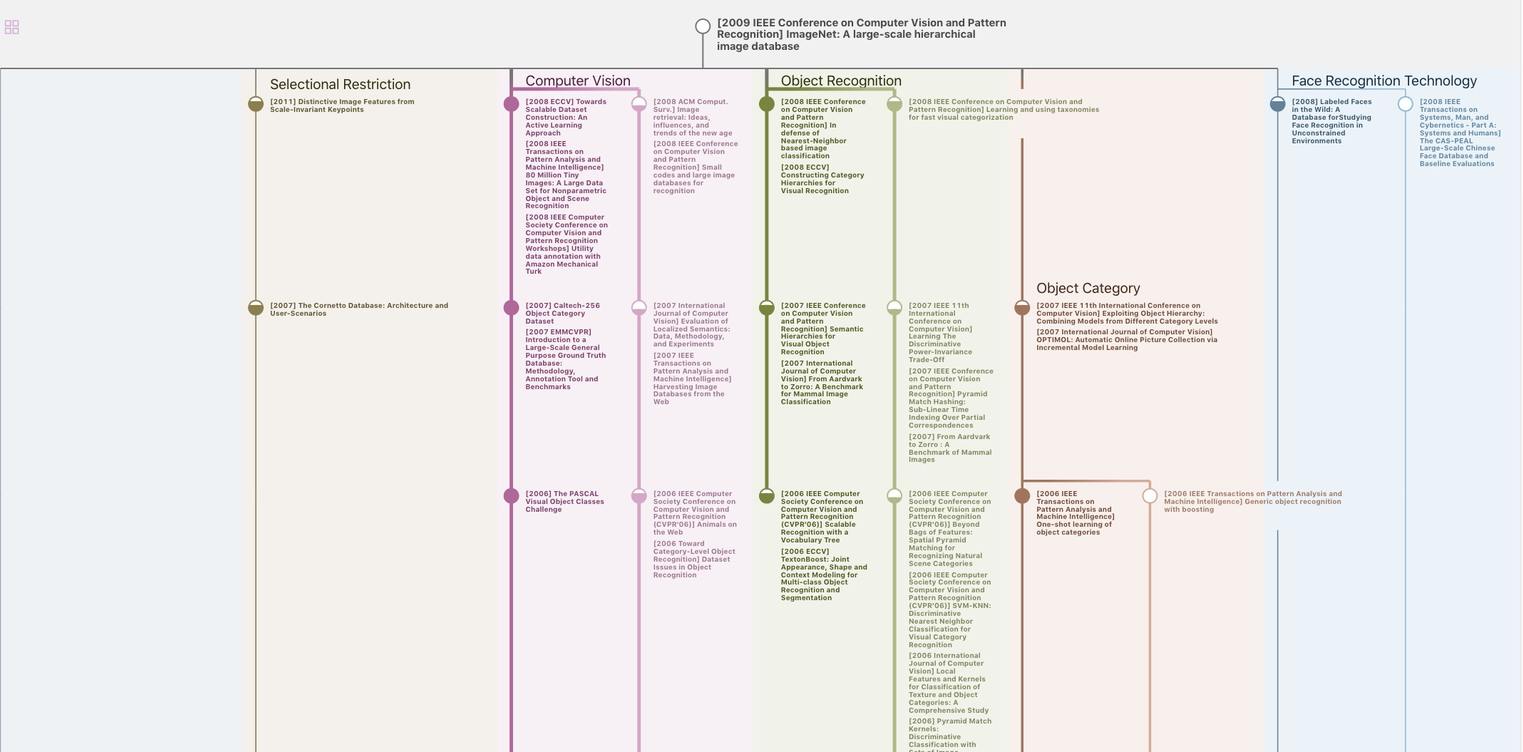
生成溯源树,研究论文发展脉络
Chat Paper
正在生成论文摘要