A Framework for Context-Sensitive Prediction in Time Series - Feasibility Study for Data-Driven Simulation in Medicine
2023 IEEE 10th International Conference on Data Science and Advanced Analytics (DSAA)(2023)
摘要
The need to comprehend and predict the dynamics of complex systems has spurred developments of time series forecasting methods across several disciplines. Nowadays, time series are collected with event logs for an ever increasing number of systems. Event logs can contain prominent information about the system dynamics. This paper addresses joint modelling of time series and event traces, to enhance time series forecasting. We introduce the Non-Homogeneous Markov Chain AutoRegressive model, to best apprehend the combined influence of past events belonging to several event categories, on a system’s dynamics. The originality of our proposal stems from the synchronization of a Hawkes temporal point process with the classical first-order hidden Markov model, through contextual variables. We also instantiate a basic version whose contextual variables only take into account events’ latest occurrences. Our proof-of-concept experiments address a real-world case related to digitally assisted training in anaesthesiology. We demonstrate that the advanced instantiation outperforms the basic instantiation (maximal prediction error percentage: 5.6% versus 13.5%). Finally, we validate the suitability of the advanced instantiation for the desired simulation, by applying real sequences of medical actions to digital patients. We show that we obtain highly realistic simulations.
更多查看译文
关键词
joint modelling,event sequences,time series,time series prediction,dynamic Bayesian network,contextual variables,Hawkes temporal point process,training in anaesthesiology
AI 理解论文
溯源树
样例
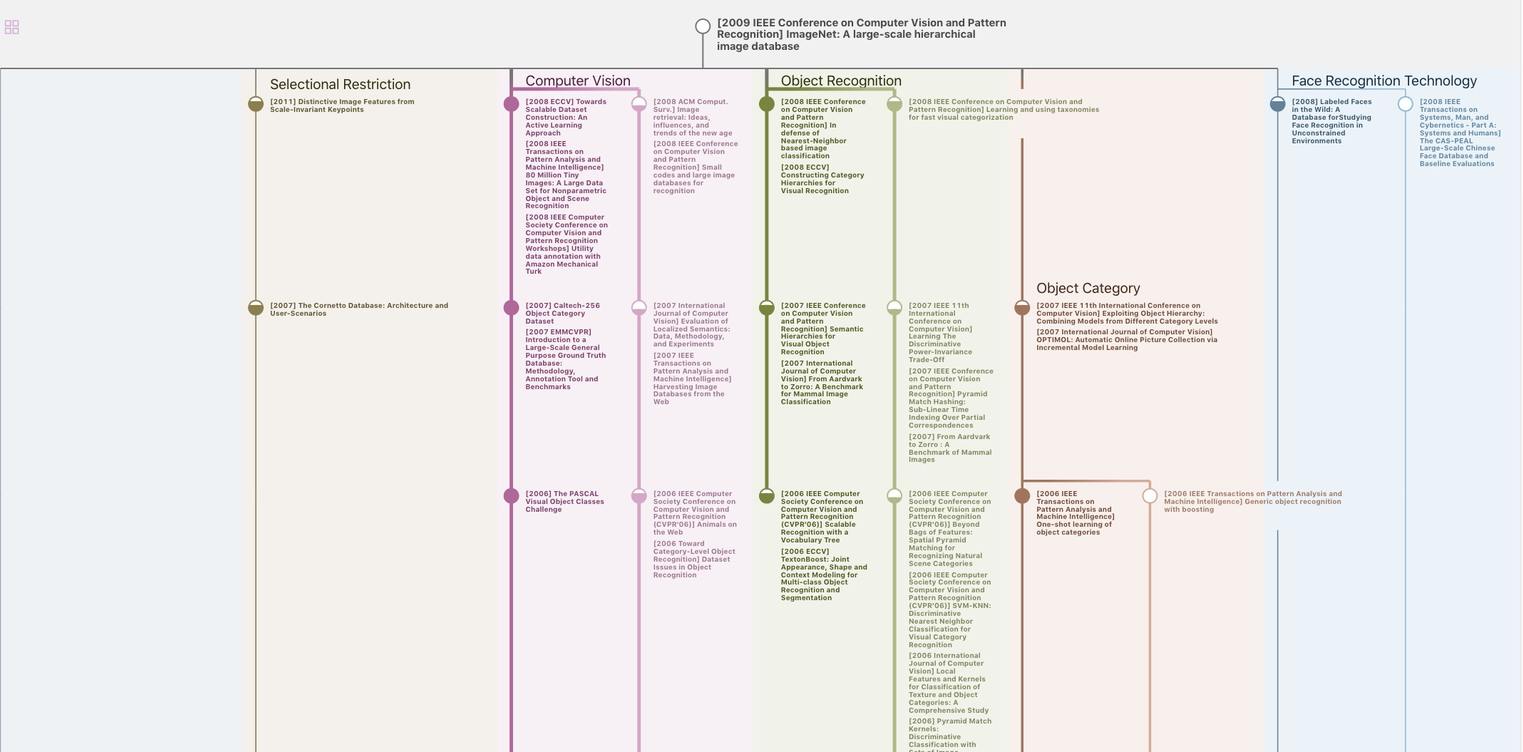
生成溯源树,研究论文发展脉络
Chat Paper
正在生成论文摘要