Unfolding temporal networks through statistically significant graph evolution rules
2023 IEEE 10th International Conference on Data Science and Advanced Analytics (DSAA)(2023)
摘要
Understanding and extracting knowledge from temporal networks is crucial to understand their dynamic nature and gain insights into their evolutionary characteristics Existing approaches to network growth often rely on single-parameterized mechanisms, neglecting the diverse and heterogeneous behaviors observed in contemporary techno-social networks. To overcome this limitation, methods based on graph evolution rules (GER) mining have proven promising GERs capture interpretable patterns describing the transformation of a small subgraph into a new subgraph, providing valuable insights into evolutionary behaviors However, current approaches primarily focus on estimating subgraph frequency, neglecting the evaluation of rule significance. To address this gap, we propose a tailored null model integrated into the GERM algorithm, the first and most stable graph evolution rule mining method. Our null model preserves the graph’s static structure while shuffling timestamps, maintaining temporal distribution, and introducing randomness to event sequences By employing a z-score test, we identify statistically significant rules deviating from the null model We evaluate our methodology on three temporal networks representing co-authorship and mutual online message exchanges Our results demonstrate that the introduction of the null model affects the evaluation and interpretation of identified rules, revealing the prevalence of under-represented rules and suggesting that temporal factors and other mechanisms may impede or facilitate evolutionary paths. These findings provide deeper insights into the dynamics and mechanisms driving temporal networks, highlighting the importance of assessing the significance of the evolution patterns in understanding network evolution.
更多查看译文
关键词
graph evolution mining,subgraph mining,graph evolution rule,null models
AI 理解论文
溯源树
样例
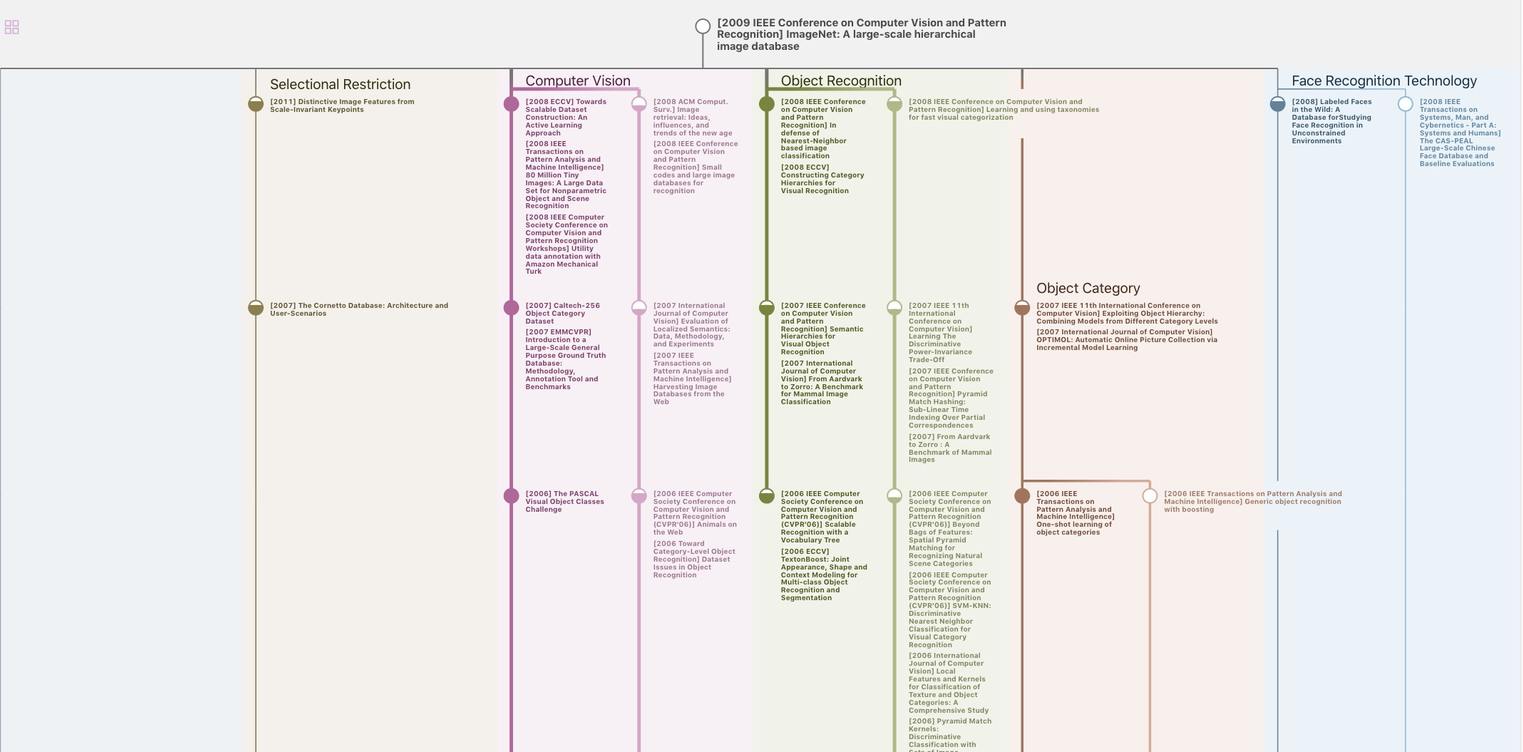
生成溯源树,研究论文发展脉络
Chat Paper
正在生成论文摘要