Data-Driven Deep Learning-Based Rate-Splitting Multiple Access for FDD Massive MIMO-OFDM Systems with Implicit CSI
2023 IEEE 24th International Workshop on Signal Processing Advances in Wireless Communications (SPAWC)(2023)
摘要
In massive multiple-input multiple-output (MIMO) orthogonal frequency division multiplexing (OFDM) systems, the acquisition of accurate channel state information (CSI) and the implementation of spectrally efficient beamforming with limited feedback and pilot overhead present significant challenges, resulting in a substantial decrease in the performance of conventional space division multiple access (SDMA) beamforming. To address these challenges, this paper proposes a novel data-driven deep learning-based rate-splitting multiple access (RSMA) beamforming technique. The proposed approach models the crucial transmission components, including downlink pilot training, uplink pilot feedback, and RSMA beamforming, as a unified end-to-end (E2E) neural network. The network is trained in an E2E manner, eliminating the need for explicit CSI acquisition with reduced pilot and feedback overhead. Simulation results demonstrate that the proposed scheme outperforms state-of-the-art approaches.
更多查看译文
关键词
rate-splitting multiple access (RSMA),orthogonal frequency division multiplexing (OFDM),multiple-input multiple-output (MIMO),precoding,deep learning
AI 理解论文
溯源树
样例
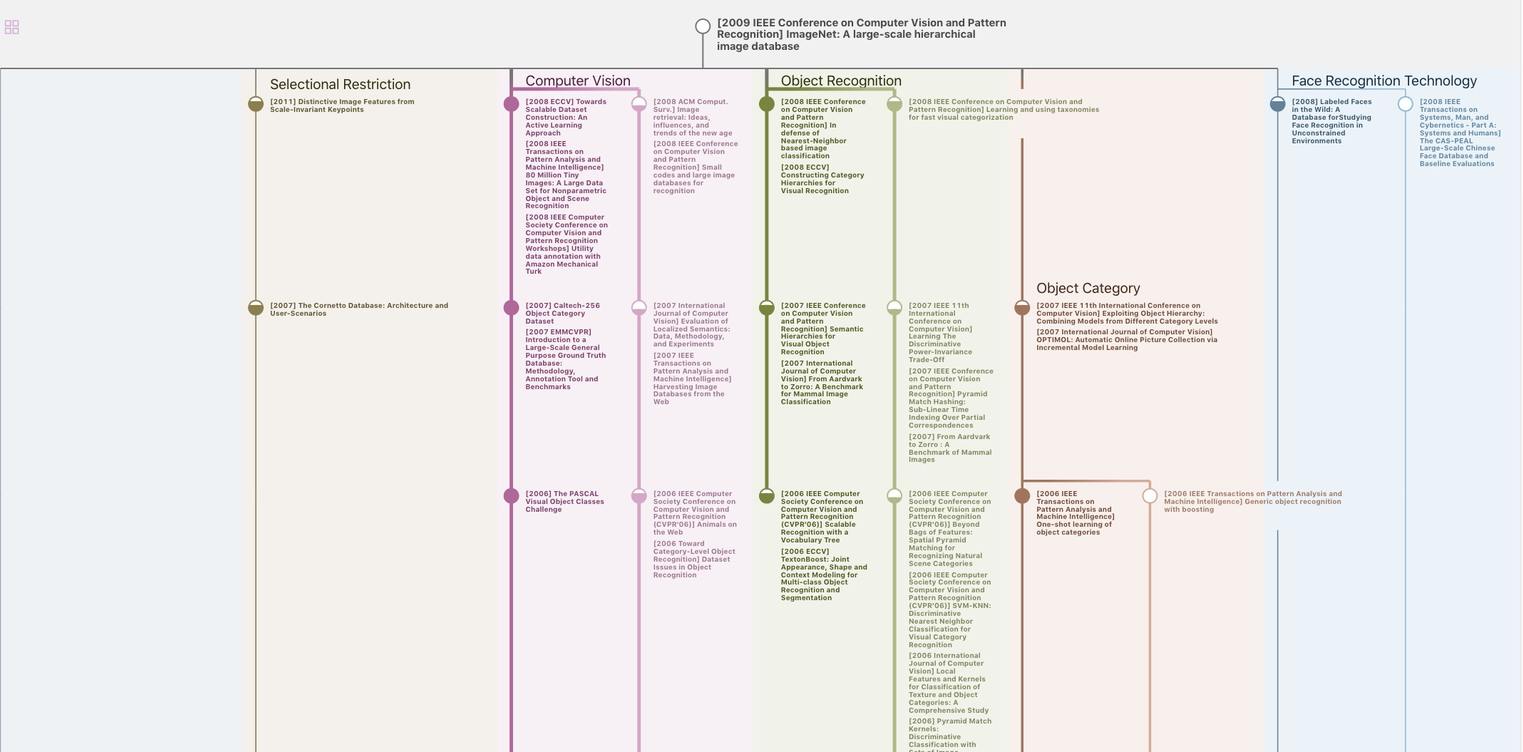
生成溯源树,研究论文发展脉络
Chat Paper
正在生成论文摘要