Receiver-Agnostic Radio Frequency Fingerprint Identification via Feature Disentanglement
2023 IEEE 24th International Workshop on Signal Processing Advances in Wireless Communications (SPAWC)(2023)
摘要
The Radio Frequency Fingerprint Identification (RFFI) technique utilizes the subtle and unintentional modulation present in the transmitted RF waveform, caused by the non-ideal characteristics of the device, to uniquely identify an emitter. RFFI has recently gained significant attention, and deep learning (DL) approach has achieved superior performance. In this paper, we study the DL-based RFFI with an emphasis on the fingerprint contamination by receivers. Specifically, during reception, the receivers’ fingerprints are inevitably introduced, and different receivers affect emitters’ fingerprints differently, thereby degrading recognition performance when the RFFI recognition model is deployed at different receivers. To mitigate the receivers’ effect, we propose a receiver-independent recognition model based on the idea of feature disentanglement. With the aid of some carefully designed model structure, loss functions and training strategy, the proposed model learns a feature space, in which the emitters’ features are disentangled from the receivers’, through multi-receiver’s training data. When deploying the model on a new receiver, the emitter-dependent features are exploited to achieve receiver-independent identification. Experiments on two real-world datasets demonstrate that our method significantly outperforms the baseline method without considering fingerprint contamination.
更多查看译文
关键词
Radio Frequency Fingerprint Identification,receiver-agnostic,domain generalization,feature disentanglement
AI 理解论文
溯源树
样例
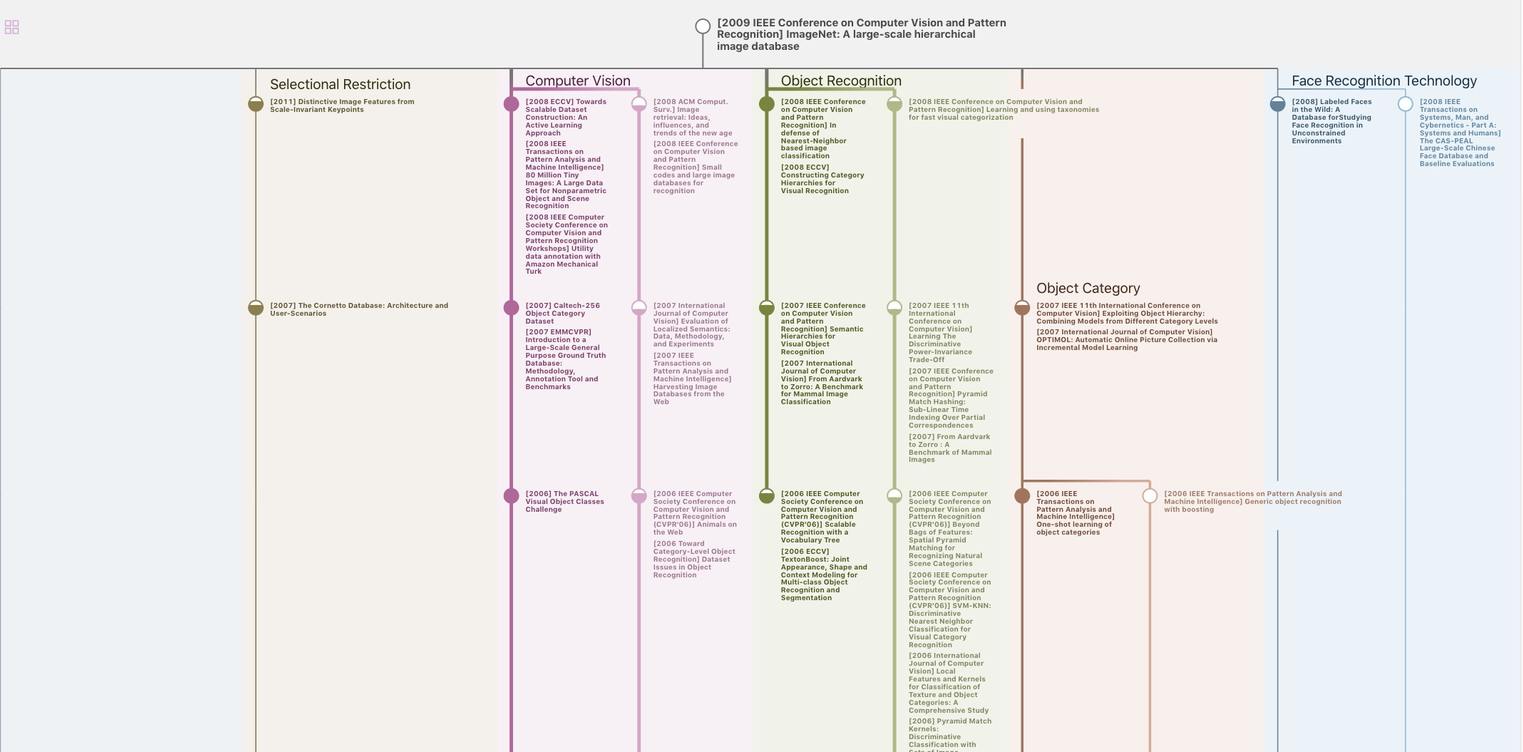
生成溯源树,研究论文发展脉络
Chat Paper
正在生成论文摘要