A Statistical Framework for Analysis of Trial-Level Temporal Dynamics in Fiber Photometry Experiments.
bioRxiv : the preprint server for biology(2024)
摘要
Fiber photometry has become a popular technique to measure neural activity in vivo, but common analysis strategies can reduce detection of effects because they condense within-trial signals into summary measures, and discard trial-level information by averaging across-trials. We propose a novel photometry statistical framework based on functional linear mixed modeling, which enables hypothesis testing of variable effects at every trial time-point, and uses trial-level signals without averaging. This makes it possible to compare the timing and magnitude of signals across conditions while accounting for between-animal differences. Our framework produces a series of plots that illustrate covariate effect estimates and statistical significance at each trial time-point. By exploiting signal autocorrelation, our methodology yields joint 95% confidence intervals that account for inspecting effects across the entire trial and improve the detection of event-related signal changes over common multiple comparisons correction strategies. We reanalyze data from a recent study proposing a theory for the role of mesolimbic dopamine in reward learning, and show the capability of our framework to reveal significant effects obscured by standard analysis approaches. Our method identifies two dopamine components with distinct temporal dynamics that may be hard to explain under currently competing learning theories. In simulation experiments, our methodology yields improved statistical power over common analysis approaches. Finally, we provide an open-source package implementing our framework.
更多查看译文
关键词
fiber photometry experiments,dynamics,trial-level
AI 理解论文
溯源树
样例
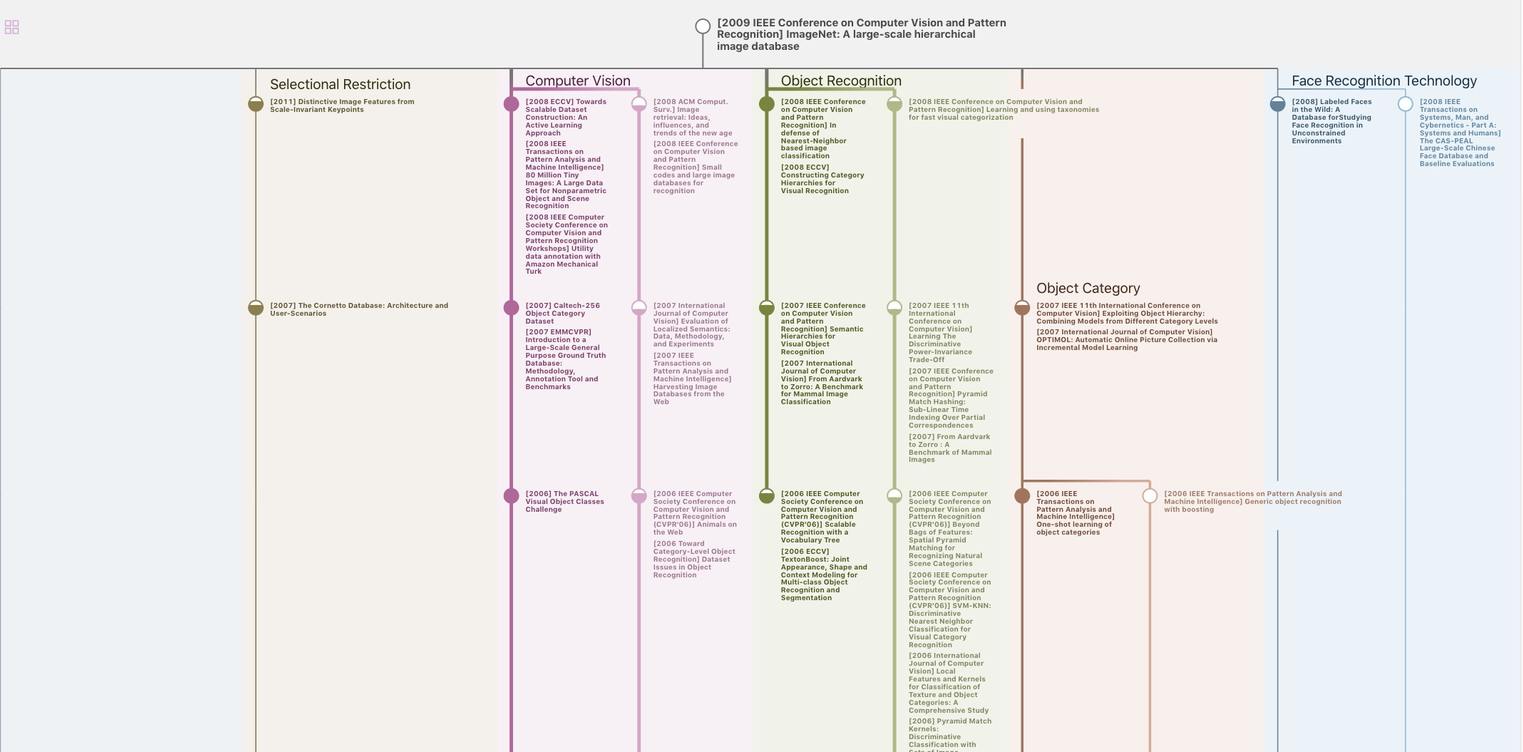
生成溯源树,研究论文发展脉络
Chat Paper
正在生成论文摘要