Automated and reproducible cell identification in mass cytometry using neural networks
Briefings in bioinformatics(2023)
摘要
The principal use of mass cytometry is to identify distinct cell types and changes in their composition, phenotype and function in different samples and conditions. Combining data from different studies has the potential to increase the power of these discoveries in diverse fields such as immunology, oncology and infection. However, current tools are lacking in scalable, reproducible and automated methods to integrate and study data sets from mass cytometry that often use heterogenous approaches to study similar samples. To address these limitations, we present two novel developments: (1) a pre-trained cell identification model named Immunopred that allows automated identification of immune cells without user-defined prior knowledge of expected cell types and (2) a fully automated cytometry meta-analysis pipeline built around Immunopred. We evaluated this pipeline on six COVID-19 study data sets comprising 270 unique samples and uncovered novel significant phenotypic changes in the wider immune landscape of COVID-19 that were not identified when each study was analyzed individually. Applied widely, our approach will support the discovery of novel findings in research areas where cytometry data sets are available for integration.
更多查看译文
关键词
mass cytometry,machine learning,single-cells,immunology
AI 理解论文
溯源树
样例
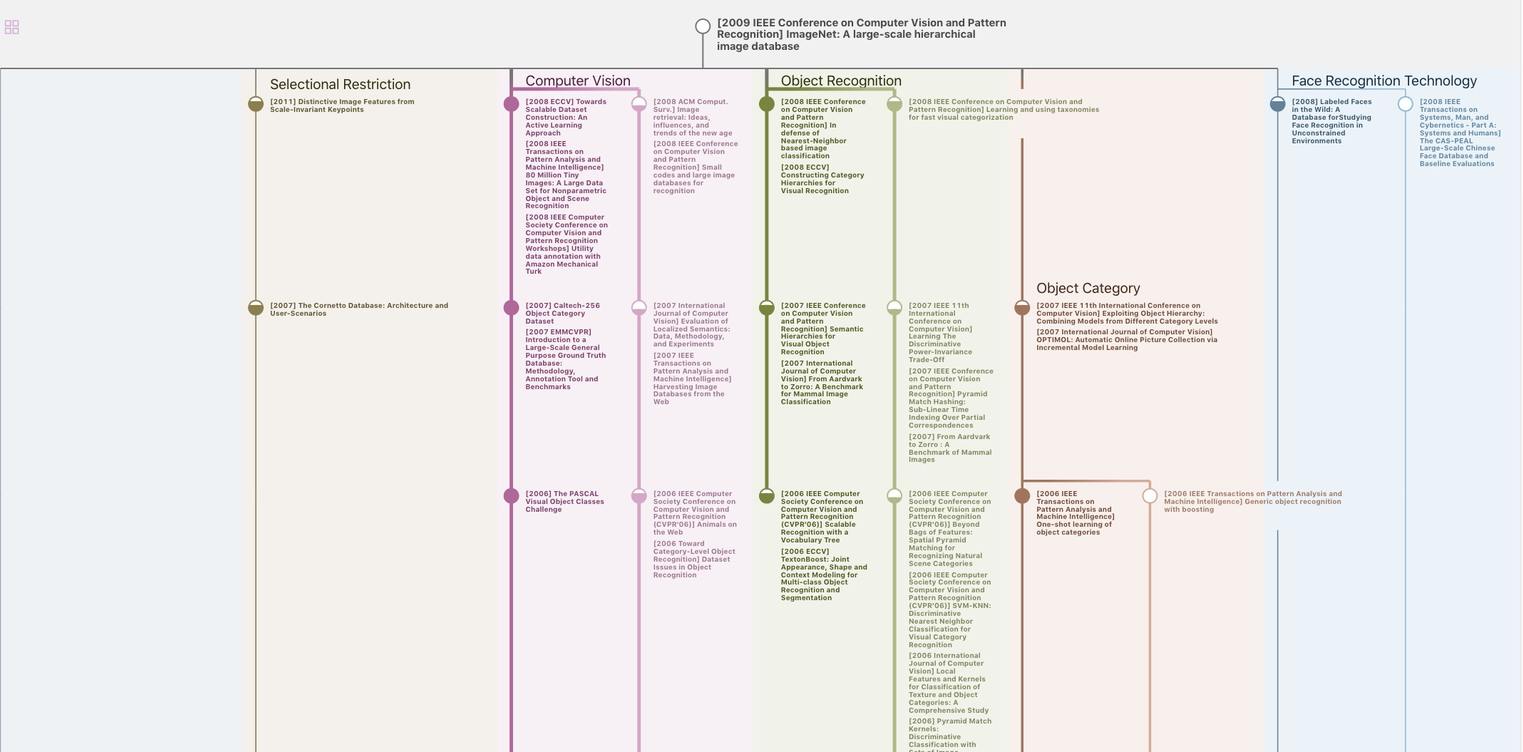
生成溯源树,研究论文发展脉络
Chat Paper
正在生成论文摘要