Cross-Image Attention for Zero-Shot Appearance Transfer.
CoRR(2023)
摘要
Recent advancements in text-to-image generative models have demonstrated a remarkable ability to capture a deep semantic understanding of images. In this work, we leverage this semantic knowledge to transfer the visual appearance between objects that share similar semantics but may differ significantly in shape. To achieve this, we build upon the self-attention layers of these generative models and introduce a cross-image attention mechanism that implicitly establishes semantic correspondences across images. Specifically, given a pair of images -- one depicting the target structure and the other specifying the desired appearance -- our cross-image attention combines the queries corresponding to the structure image with the keys and values of the appearance image. This operation, when applied during the denoising process, leverages the established semantic correspondences to generate an image combining the desired structure and appearance. In addition, to improve the output image quality, we harness three mechanisms that either manipulate the noisy latent codes or the model's internal representations throughout the denoising process. Importantly, our approach is zero-shot, requiring no optimization or training. Experiments show that our method is effective across a wide range of object categories and is robust to variations in shape, size, and viewpoint between the two input images.
更多查看译文
关键词
attention,appearance,transfer,cross-image,zero-shot
AI 理解论文
溯源树
样例
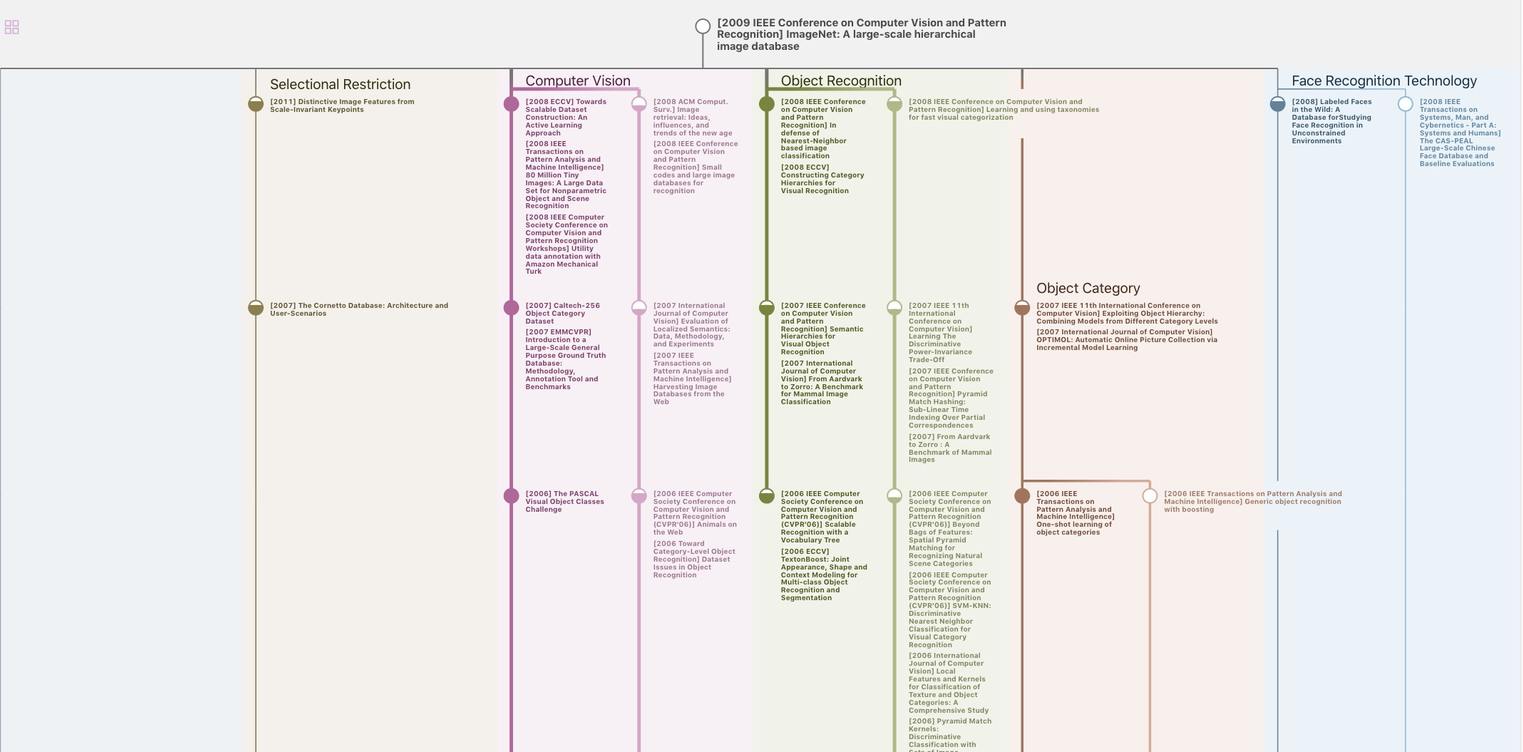
生成溯源树,研究论文发展脉络
Chat Paper
正在生成论文摘要