Preserving Privacy in GANs Against Membership Inference Attack
IEEE TRANSACTIONS ON INFORMATION FORENSICS AND SECURITY(2024)
摘要
Generative Adversarial Networks (GANs) have been widely used for generating synthetic data for cases where there is a limited size real-world data set or when data holders are unwilling to share their data samples. Recent works showed that GANs, due to overfitting and memorization, might leak information regarding their training data samples. This makes GANs vulnerable to Membership Inference Attacks (MIAs). Several defense strategies have been proposed in the literature to mitigate this privacy issue. Unfortunately, defense strategies based on differential privacy are proven to reduce extensively the quality of the synthetic data points. On the other hand, more recent frameworks such as PrivGAN and PAR-GAN are not suitable for small-size training data sets. In the present work, the overfitting in GANs is studied in terms of the discriminator, and a more general measure of overfitting based on the Bhattacharyya coefficient is defined. Then, inspired by Fano's inequality, our first defense mechanism against MIAs is proposed. This framework, which requires only a simple modification in the loss function of GANs, is referred to as the maximum entropy GAN or MEGAN and significantly improves the robustness of GANs to MIAs. As a second defense strategy, a more heuristic model based on minimizing the information leaked from the generated samples about the training data points is presented. This approach is referred to as mutual information minimization GAN (MIMGAN) and uses a variational representation of the mutual information to minimize the information that a synthetic sample might leak about the whole training data set. Applying the proposed frameworks to some commonly used data sets against state-of-the-art MIAs reveals that the proposed methods can reduce the accuracy of the adversaries to the level of random guessing accuracy with a small reduction in the quality of the synthetic data samples.
更多查看译文
关键词
Training,Training data,Generators,Data models,Mutual information,Synthetic data,Modeling,Generative adversarial networks,GANs,membership inference attacks,mutual information,maximum entropy
AI 理解论文
溯源树
样例
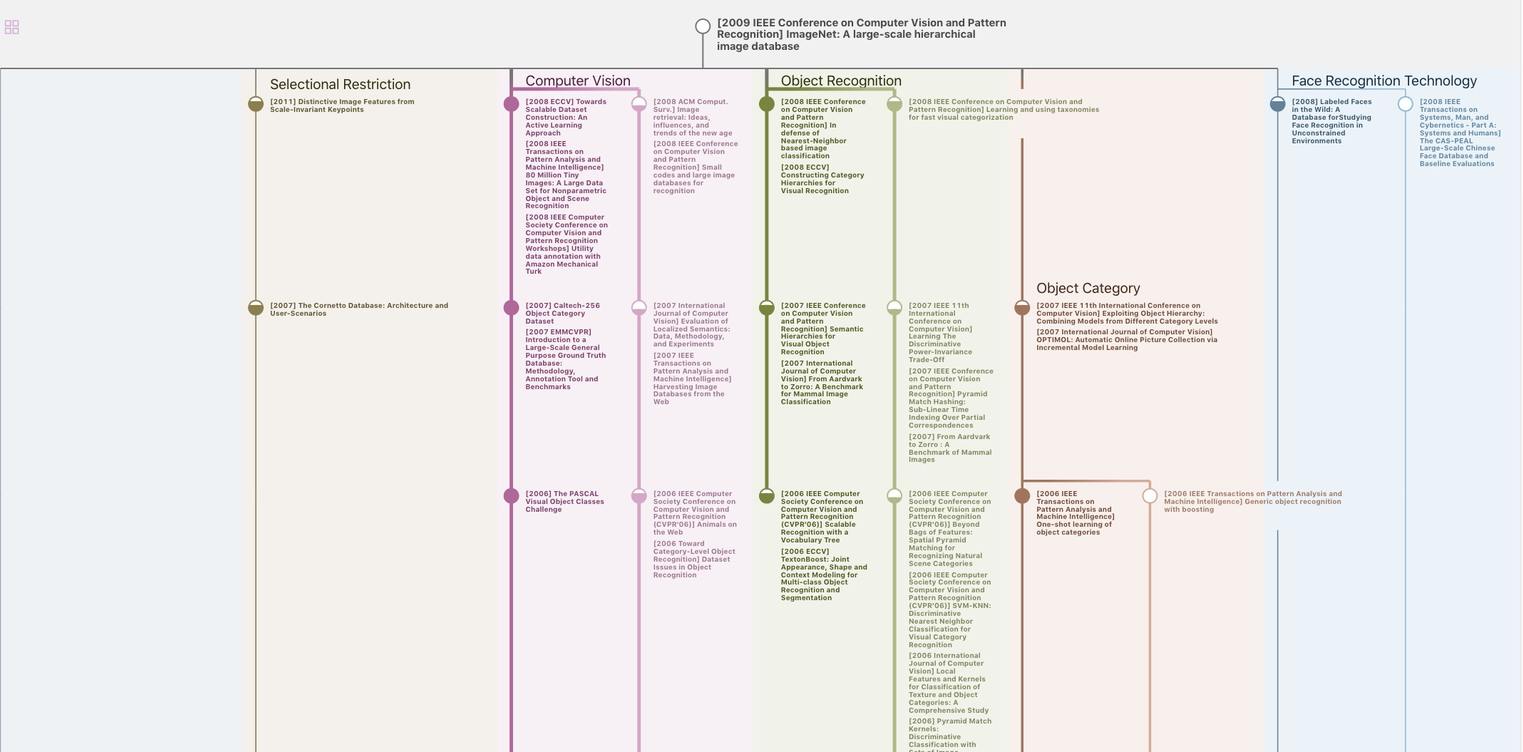
生成溯源树,研究论文发展脉络
Chat Paper
正在生成论文摘要