Truly Scale-Equivariant Deep Nets with Fourier Layers
NeurIPS(2023)
摘要
In computer vision, models must be able to adapt to changes in image resolution to effectively carry out tasks such as image segmentation; This is known as scale-equivariance. Recent works have made progress in developing scale-equivariant convolutional neural networks, e.g., through weight-sharing and kernel resizing. However, these networks are not truly scale-equivariant in practice. Specifically, they do not consider anti-aliasing as they formulate the down-scaling operation in the continuous domain. To address this shortcoming, we directly formulate down-scaling in the discrete domain with consideration of anti-aliasing. We then propose a novel architecture based on Fourier layers to achieve truly scale-equivariant deep nets, i.e., absolute zero equivariance-error. Following prior works, we test this model on MNIST-scale and STL-10 datasets. Our proposed model achieves competitive classification performance while maintaining zero equivariance-error.
更多查看译文
关键词
layers,fourier,deep,scale-equivariant
AI 理解论文
溯源树
样例
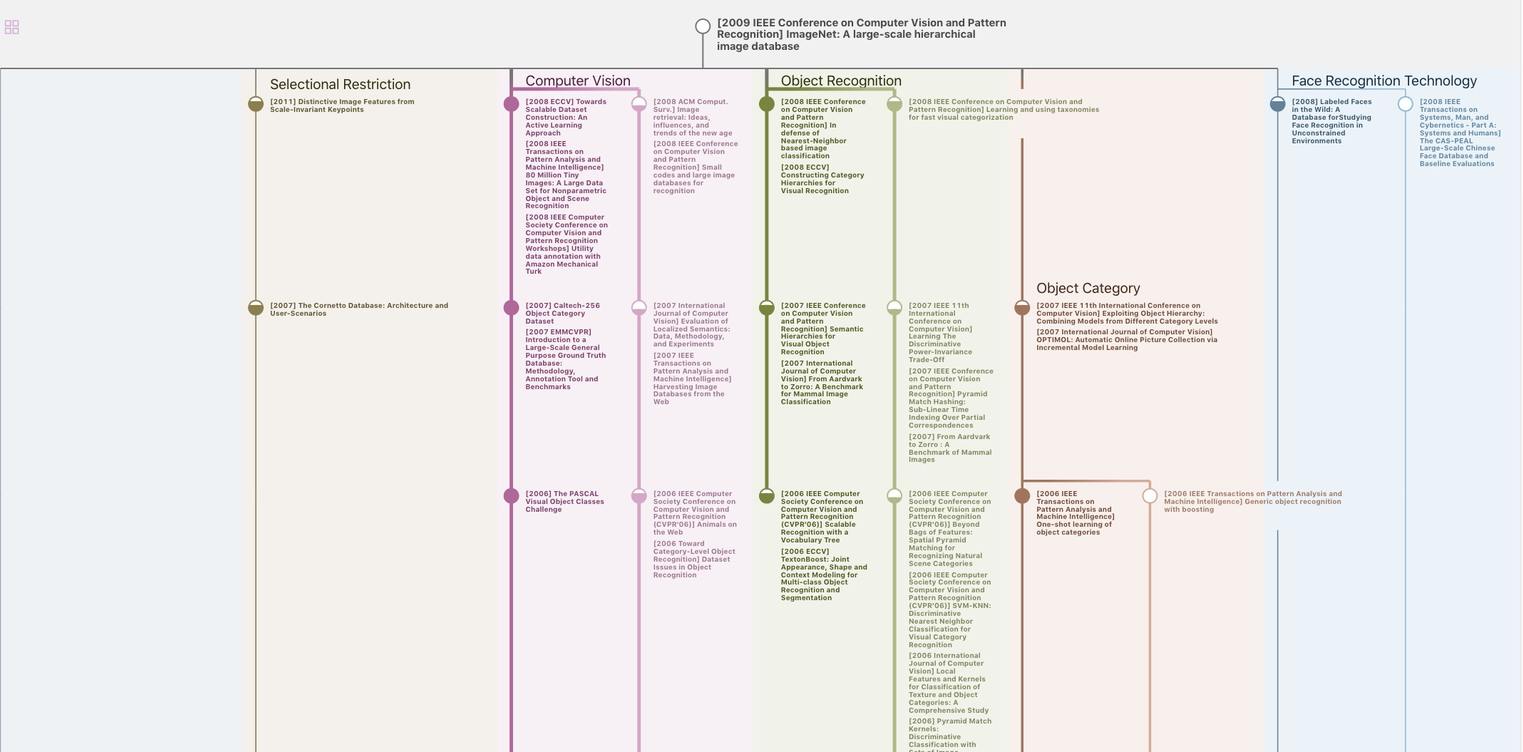
生成溯源树,研究论文发展脉络
Chat Paper
正在生成论文摘要