CausalCite: A Causal Formulation of Paper Citations
CoRR(2023)
摘要
Evaluating the significance of a paper is pivotal yet challenging for the
scientific community. While the citation count is the most commonly used proxy
for this purpose, they are widely criticized for failing to accurately reflect
a paper's true impact. In this work, we propose a causal inference method,
TextMatch, which adapts the traditional matching framework to high-dimensional
text embeddings. Specifically, we encode each paper using the text embeddings
by large language models (LLMs), extract similar samples by cosine similarity,
and synthesize a counterfactual sample by the weighted average of similar
papers according to their similarity values. We apply the resulting metric,
called CausalCite, as a causal formulation of paper citations. We show its
effectiveness on various criteria, such as high correlation with paper impact
as reported by scientific experts on a previous dataset of 1K papers,
(test-of-time) awards for past papers, and its stability across various
sub-fields of AI. We also provide a set of findings that can serve as suggested
ways for future researchers to use our metric for a better understanding of a
paper's quality. Our code and data are at
https://github.com/causalNLP/causal-cite.
更多查看译文
关键词
citations,causalcite formulation,paper
AI 理解论文
溯源树
样例
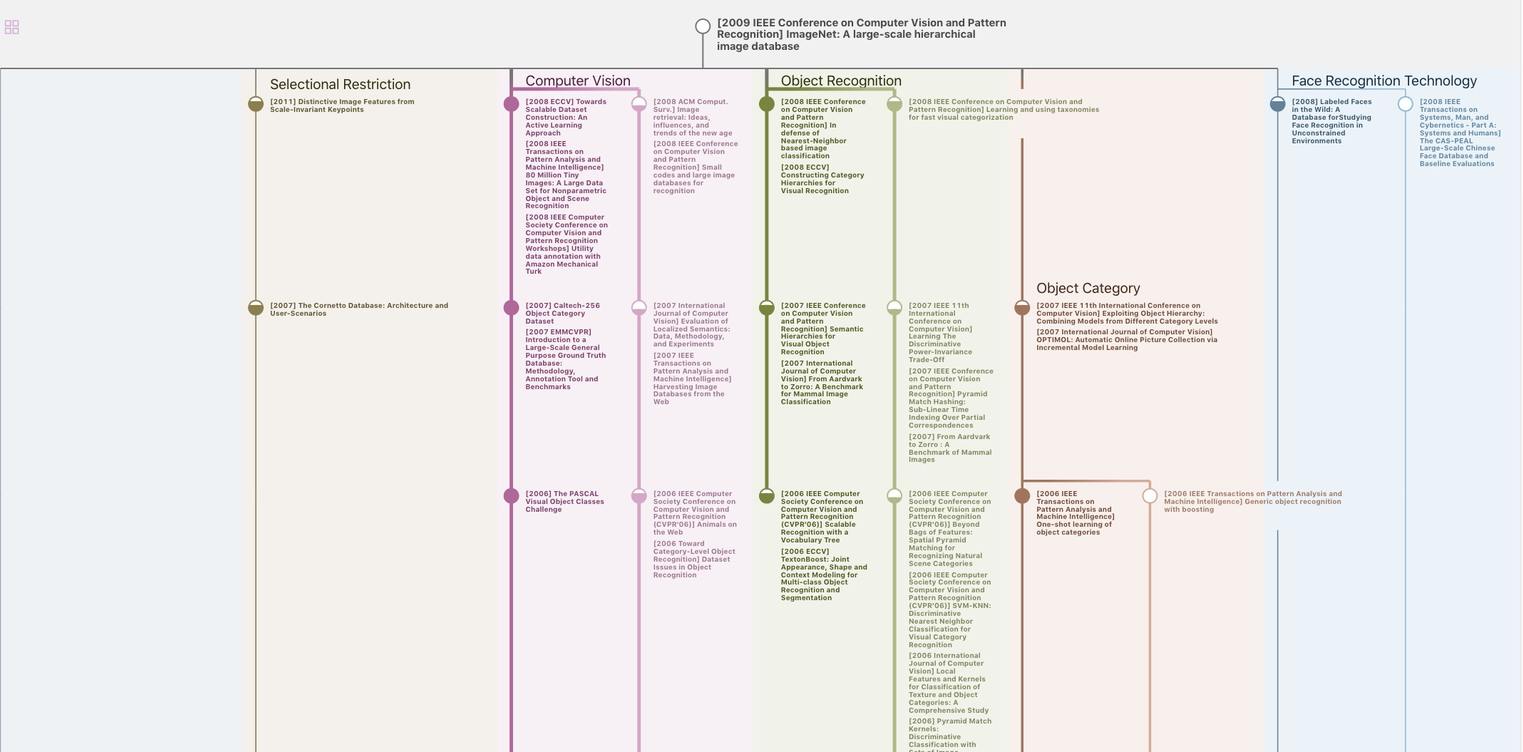
生成溯源树,研究论文发展脉络
Chat Paper
正在生成论文摘要