Neural Network Reconstruction of the Left Atrium using Sparse Catheter Paths.
CoRR(2023)
摘要
Catheter based radiofrequency ablation for pulmonary vein isolation has become the first line of treatment for atrial fibrillation in recent years. This requires a rather accurate map of the left atrial sub-endocardial surface including the ostia of the pulmonary veins, which requires dense sampling of the surface and takes more than 10 minutes. The focus of this work is to provide left atrial visualization early in the procedure to ease procedure complexity and enable further workflows, such as using catheters that have difficulty sampling the surface. We propose a dense encoder-decoder network with a novel regularization term to reconstruct the shape of the left atrium from partial data which is derived from simple catheter maneuvers. To train the network, we acquire a large dataset of 3D atria shapes and generate corresponding catheter trajectories. Once trained, we show that the suggested network can sufficiently approximate the atrium shape based on a given trajectory. We compare several network solutions for the 3D atrium reconstruction. We demonstrate that the solution proposed produces realistic visualization using partial acquisition within a 3-minute time interval. Synthetic and human clinical cases are shown.
更多查看译文
关键词
sparse catheter,left atrium,neural network
AI 理解论文
溯源树
样例
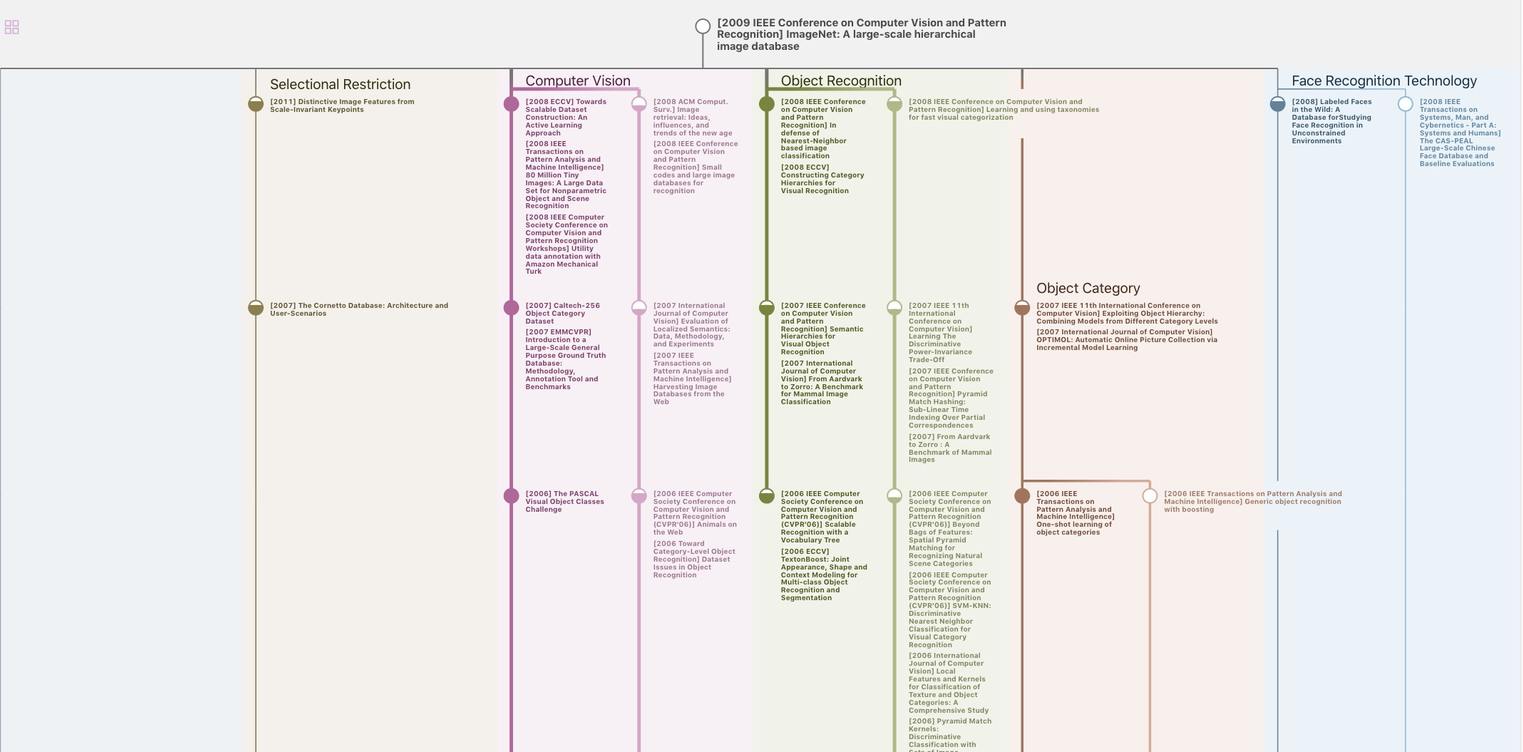
生成溯源树,研究论文发展脉络
Chat Paper
正在生成论文摘要