Multi-State Brain Network Discovery.
CoRR(2023)
摘要
Brain network discovery aims to find nodes and edges from the spatio-temporal signals obtained by neuroimaging data, such as fMRI scans of human brains. Existing methods tend to derive representative or average brain networks, assuming observed signals are generated by only a single brain activity state. However, the human brain usually involves multiple activity states, which jointly determine the brain activities. The brain regions and their connectivity usually exhibit intricate patterns that are difficult to capture with only a single-state network. Recent studies find that brain parcellation and connectivity change according to the brain activity state. We refer to such brain networks as multi-state, and this mixture can help us understand human behavior. Thus, compared to a single-state network, a multi-state network can prevent us from losing crucial information of cognitive brain network. To achieve this, we propose a new model called MNGL (Multi-state Network Graphical Lasso), which successfully models multi-state brain networks by combining CGL (coherent graphical lasso) with GMM (Gaussian Mixture Model). Using both synthetic and real world ADHD 200 fMRI datasets, we demonstrate that MNGL outperforms recent state-of-the-art alternatives by discovering more explanatory and realistic results.
更多查看译文
关键词
brain networks,edge detection,graphical lasso,mixture model
AI 理解论文
溯源树
样例
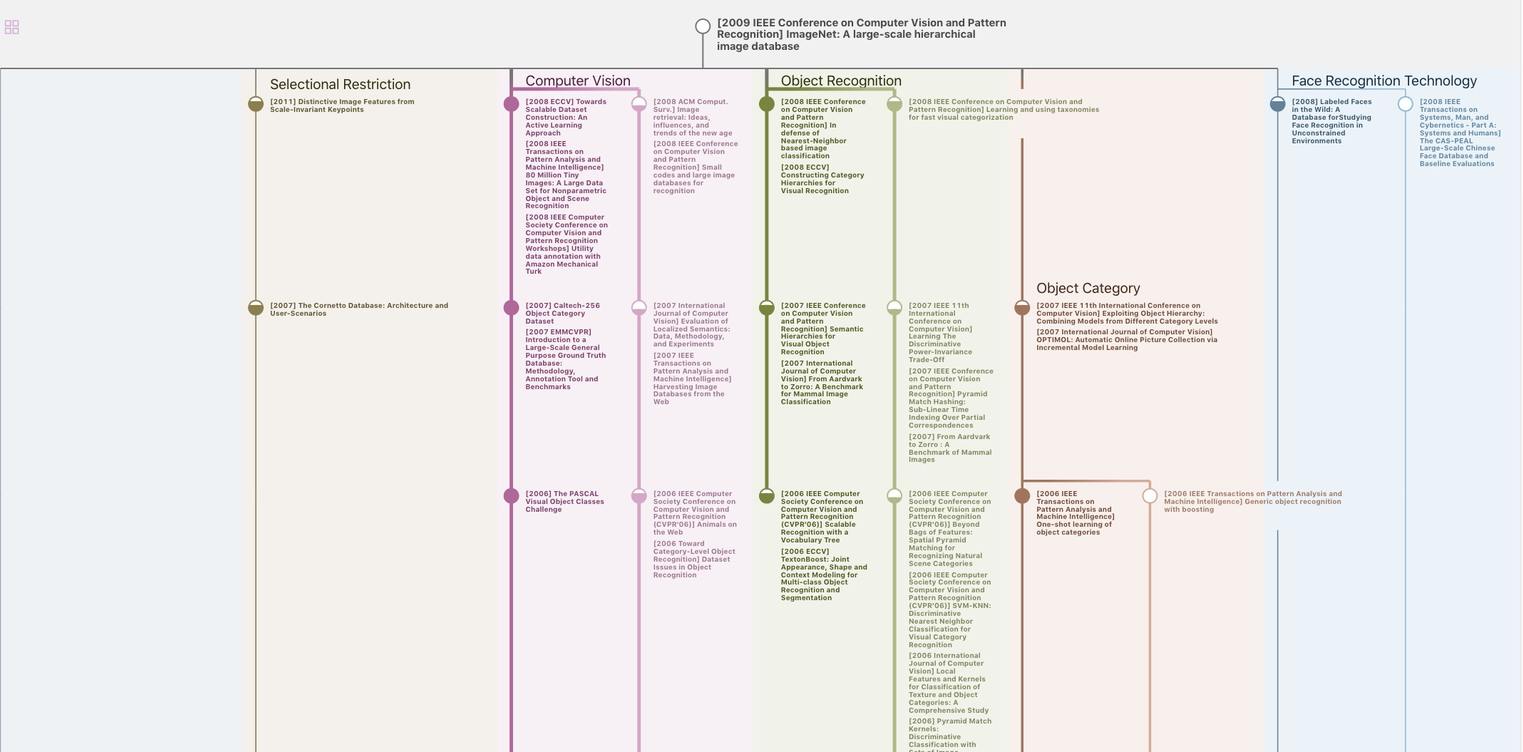
生成溯源树,研究论文发展脉络
Chat Paper
正在生成论文摘要