MF2POSE: Multi-task Feature Fusion Pseudo-Siamese Network for intrusion detection using Category-distance Promotion Loss
KNOWLEDGE-BASED SYSTEMS(2024)
摘要
Intrusion detection is a crucial aspect of modern cybersecurity, aimed at identifying and responding to potential security threats within computer systems, networks, and applications. One of the major challenges faced by intrusion detection systems is the accurate detection and response to attack traffic, which is typically much lower in volume compared to normal traffic. This challenge becomes even more pronounced when the attack traffic is further classified into different attack categories, which may suffer from more severe data imbalance. To address these challenges, we present a novel approach to intrusion detection using a Multi-task Feature Fusion Pseudo-Siamese Network (MF2POSE) and a Category-distance Promotion Loss (CP Loss). The proposed MF2POSE leverages the Pseudo-Siamese Network (POSE-Net), which incorporates both a main network and a siamese network, to simultaneously perform binary-class and multi-class classification of the traffic. Furthermore, the Multi-task Feature Fusion (MF2) module enhances the multi-class classification performance of the main network by incorporating multi-scale internal features from the siamese network. Additionally, the CP Loss is introduced to aggregate the internal feature from the main network belonging to the same category, and separate the internal feature belonging to the different categories. We have conducted comprehensive experiments across two popular intrusion detection datasets, and the experimental results demonstrate the superior performance of our MF2POSE compared to existing state-of-the-art techniques, particularly in multi-class classification scenarios.
更多查看译文
关键词
Intrusion detection,Convolutional neural network,Pseudo siamese network,Multi-task feature fusion,Category-distance promotion loss
AI 理解论文
溯源树
样例
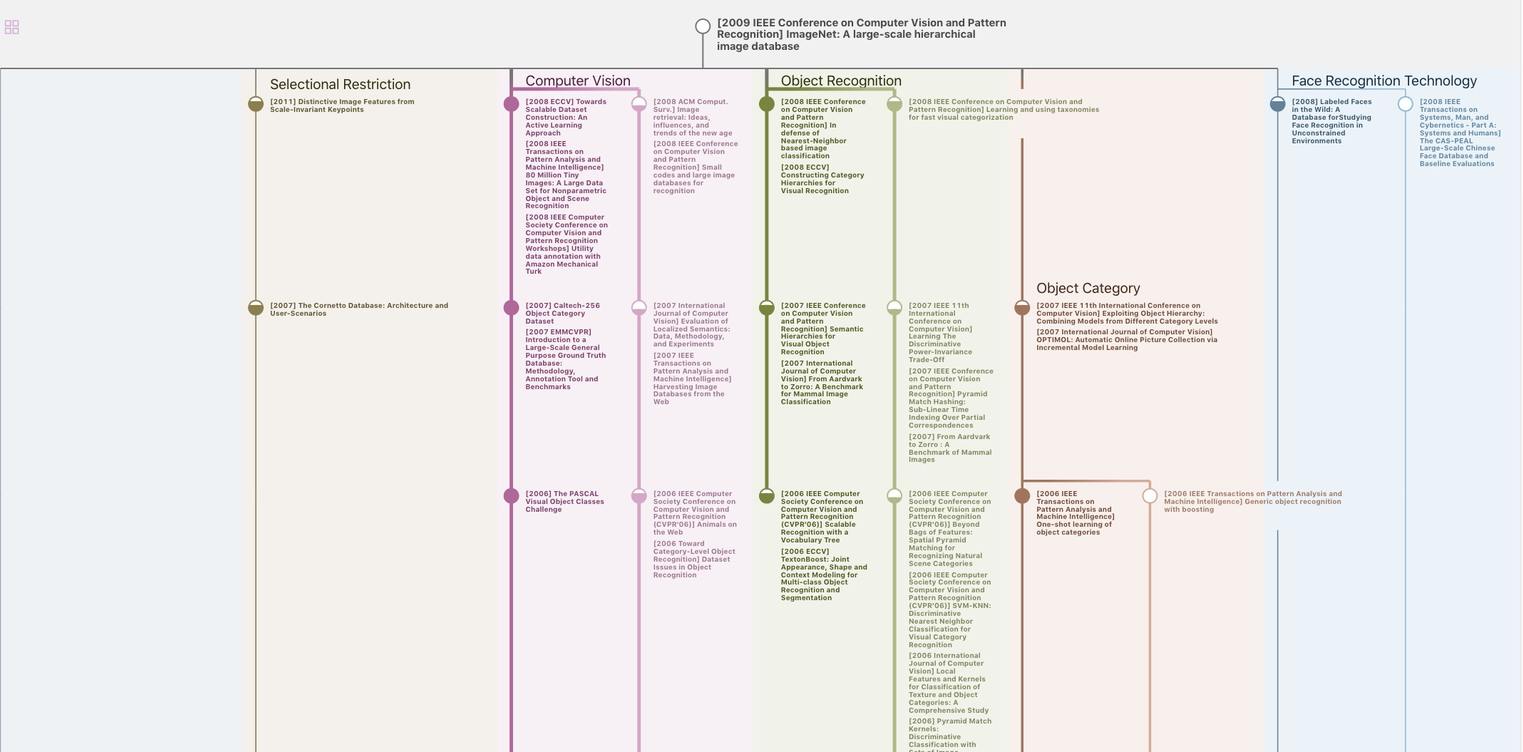
生成溯源树,研究论文发展脉络
Chat Paper
正在生成论文摘要