Inverse identification of plastic anisotropy through multiple non-conventional mechanical experiments
INTERNATIONAL JOURNAL OF SOLIDS AND STRUCTURES(2023)
摘要
In theory, a single non-conventional mechanical experiment generating inhomogeneous strain fields enables to inversely identify an anisotropic yield function. However, several studies have shown that it is challenging to design such a sufficiently data-rich experiment, particularly when it must be conducted on a standard uniaxial tensile machine. Instead of relying on a single non-conventional uniaxial tensile experiment, combining multiple non-conventional experiments is proposed to inversely identify an advanced anisotropic yield function. The feasibility of the proposed method is verified through the inverse identification of the Yld2000-2d yield function using synthetically generated Digital Image Correlation (DIC) data. This approach accounts for the metrological aspects of DIC while avoiding potential experimental errors and uncertainties related to the selected material model. It has been demonstrated that when a single non-conventional tensile test is combined with a non conventional biaxial tensile test, the inversely identified anisotropy parameters are in good agreement with those found through the conventional method. This finding is contingent on maintaining an equal contribution of the strain states from each experiment to the cost function. Excluding overlapping data points and assigning proper weights to the trustworthy data based on the strain state and the level of plastic deformation is found to be key. The numerical results are experimentally validated, thereby revealing the crucial role of the adopted anisotropic yield function. Furthermore, the uncertainty associated with the inversely identified parameters based on three repetitions of the same experiment is discussed.
更多查看译文
关键词
Anisotropic yield criteria,Non-conventional mechanical test,Inverse identification,Finite Element Model Updating,Digital Image Correlation
AI 理解论文
溯源树
样例
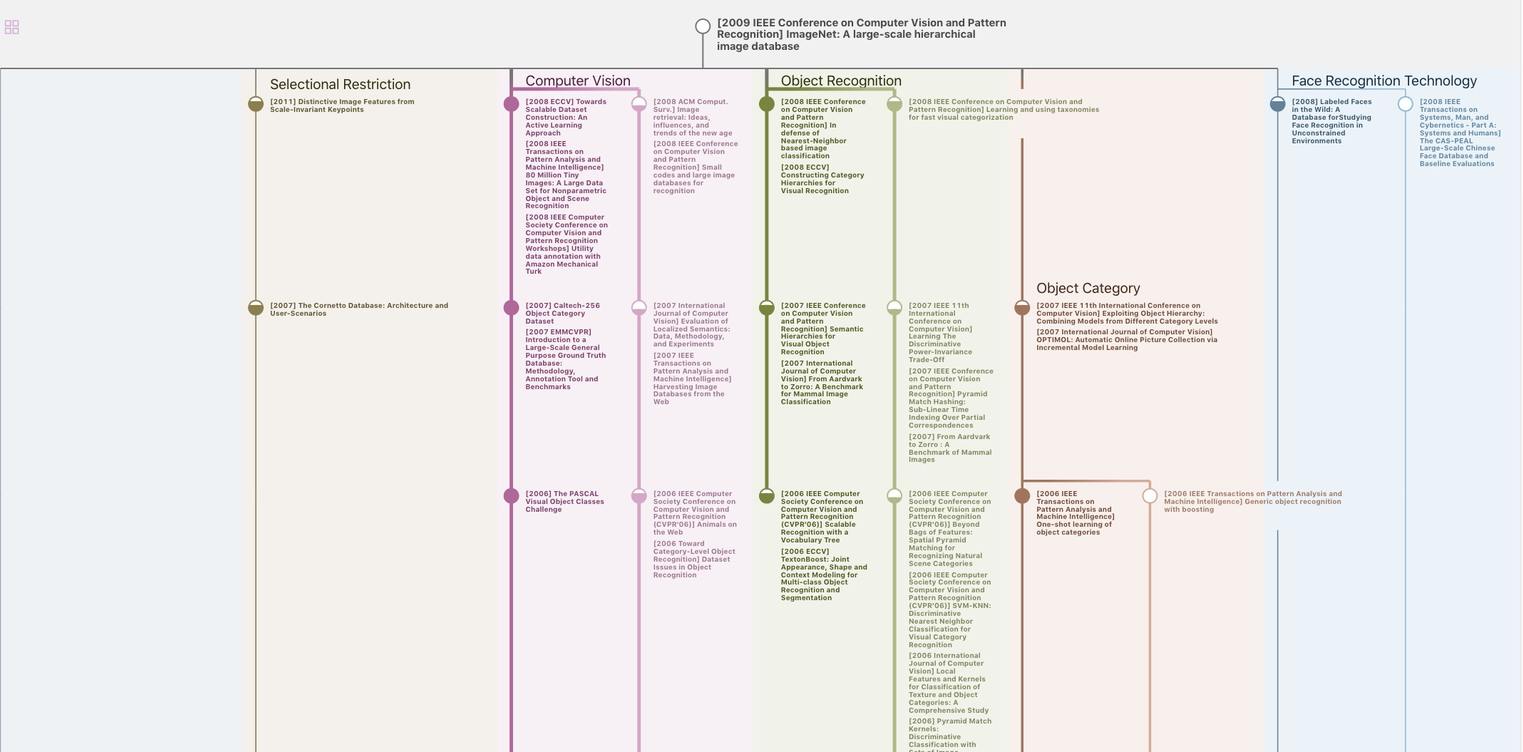
生成溯源树,研究论文发展脉络
Chat Paper
正在生成论文摘要