Multiple kernel graph clustering with shifted Laplacian reconstruction
ENGINEERING APPLICATIONS OF ARTIFICIAL INTELLIGENCE(2024)
摘要
Multiple kernel graph clustering methods have gained favor among researchers for their ability to combine the strengths of graph learning and multiple kernel methods. We propose a novel method named multiple kernel graph clustering with shifted Laplacian reconstruction (SLR-MKGC). Specifically, the kernel matrix is regarded as an affinity graph, and then the graph is transformed to a shifted Laplacian matrix. By decomposing the shifted Laplacian matrix, the latent data representation is obtained, simultaneously preserving the main energy and clustering information. As a result, the effects of noise and redundancy are reduced and the quality of the raw data are improved. Then, the obtained representation is used to reconstruct a final affinity graph with a desired block diagonal structure. Further, we conduct extensive experiments on seven benchmark datasets and compare nine state-of-the-art clustering methods. Experimental results show that SLR-MKGC exhibits excellent performance on most datasets. For example, compared to other state-of-the-art methods, SLR-MKGC achieves a performance improvement of 5.13% on the clustering accuracy term on the BBCSport2 dataset, demonstrating the promise of SLR-MKGC. The source code is available at https://github.com/dililidida/SLR-MKGC.
更多查看译文
关键词
Multiple kernel clustering,Graph clustering,Block diagonal structure,Shifted Laplacian reconstruction
AI 理解论文
溯源树
样例
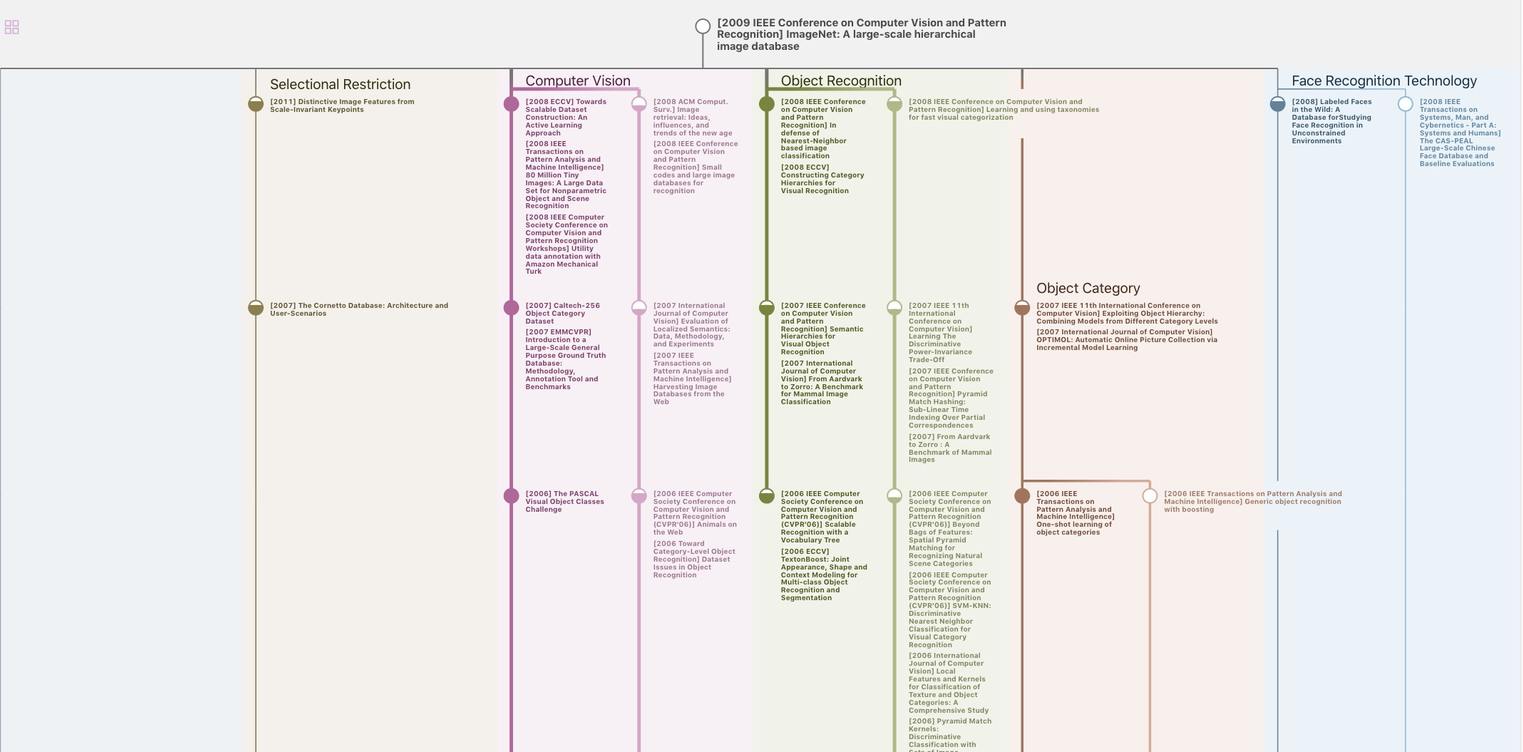
生成溯源树,研究论文发展脉络
Chat Paper
正在生成论文摘要