Joint Specifics and Dual-Semantic Hashing Learning for Cross-Modal Retrieval
NEUROCOMPUTING(2024)
摘要
Due to its low memory and computational requirements, hashing techniques are widely applied for cross modal retrieval. However, there are still two unresolved issues: 1) the class-wise similarity of samples for each modality is not well exploited, and 2) most methods ignore the discriminative capacity of modality-specific information. To solve these two issues, we propose a novel supervised cross-modal hashing method called Joint Specifics and Dual-Semantic Hashing Learning for Cross-Modal Retrieval (SDSHL). SDSHL consists of three methods, i.e., Semantic Embedded Triple Matrix Factorization (SETMF), Modality Specific Dual Semantic Learning (MSDSL) and Modality Consistent Dual Semantic Learning (MCDSL). SETMF utilizes triple matrix factorization to fully explore modality features. MSDSL applies clustering to find the class-wise similarity for each modality, preserving modality-specific information well. MCDSL adopts asymmetric distance-distance difference minimization to capture modality-consistent information among modalities. By using SDSHL, the discrepancies between features and labels are reduced, while both modality-specific and modality-consistent information is well preserved in a shared hash code. Comprehensive experimentation on three benchmark datasets demonstrates the superior performance of SDSHL.
更多查看译文
关键词
Cross-modal,Similarity searching,Label semantic,Sample semantic
AI 理解论文
溯源树
样例
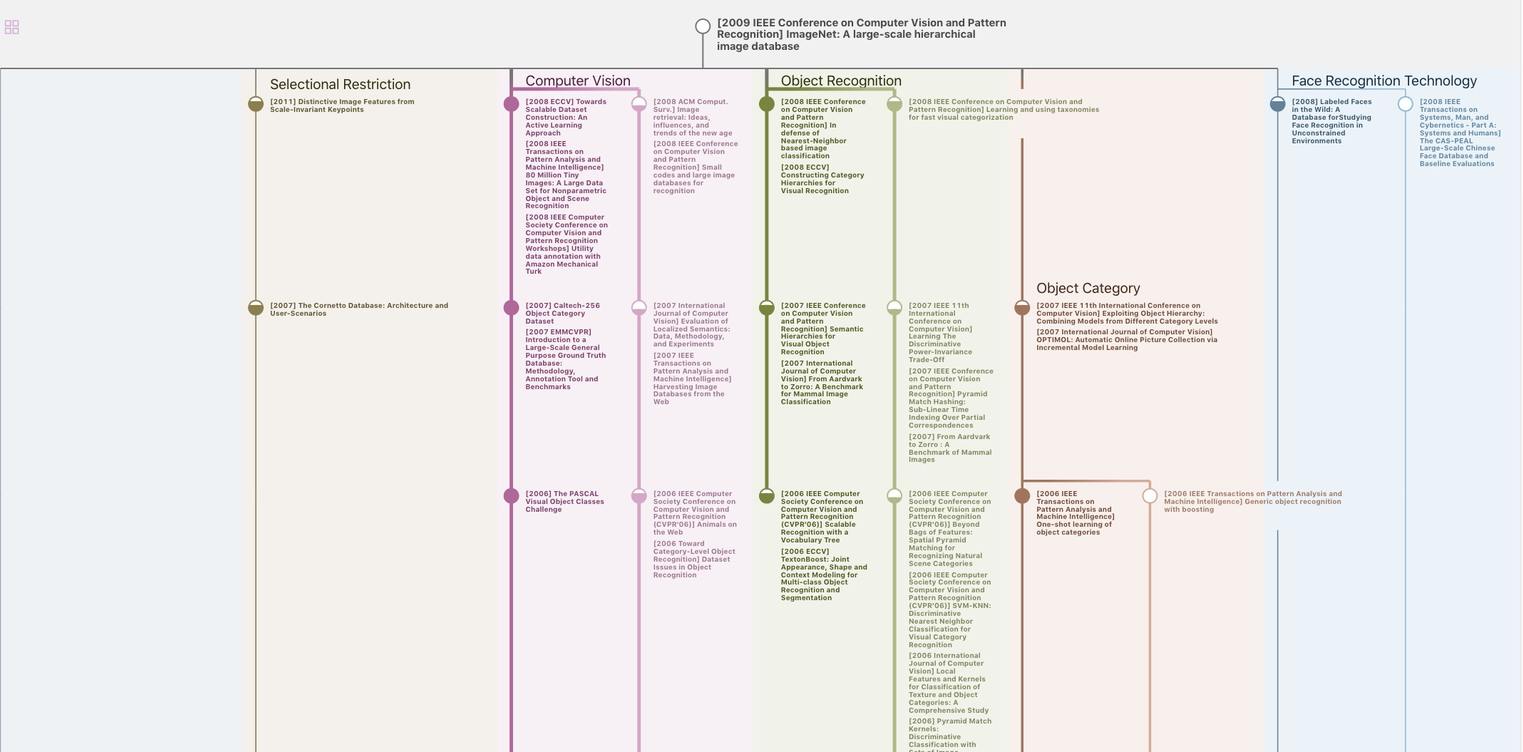
生成溯源树,研究论文发展脉络
Chat Paper
正在生成论文摘要