A framework for label noise filters
PATTERN RECOGNITION(2024)
摘要
In real applications, label noise has a great influence on data modeling. As one kind of label noise treatment method, noise filter has attracted extensive attention recently. The existing filters perform well in dealing with label noise completely at random (NCAR) but poorly in dealing with the label noise in the form of clusters (LLC). Besides, the existing filters may over remove the samples located at the classification boundaries, thereby affecting the generalization performance of classifiers. To fill these gaps, we propose a general elevating framework for label noise filters. The core idea of the framework is to improve the filtering performance of the existing filters by sample reduction. Specifically, since most of the existing filters are based on classifier prediction, and the complexity of samples at the boundary will affect the prediction performance of classifiers. Therefore, reducing the complexity of the boundary sample is very helpful to improve the performance of the filters. To this end, we propose a sample reduction method, which can not only reduce the complexity of the sample at the boundary but also convert LLC to NCAR, to get some representative samples. Next, the filter based on classifier prediction is employed to recognize the noisy representative samples. Finally, the noisy labeled samples in the given data set are found according to the identified noisy representatives. Furthermore, through empirical analysis, we found that compared with some classical metrics for evaluating the performance of noise filters, classification accuracy is more suitable to measure the performance of filters. Exhaustive experiments testify the validity of the framework, and the experimental results demonstrate that the performance of our framework is especially outstanding for LLC treatment.
更多查看译文
关键词
Classification,Label noise,Noise filter,Sample reduction
AI 理解论文
溯源树
样例
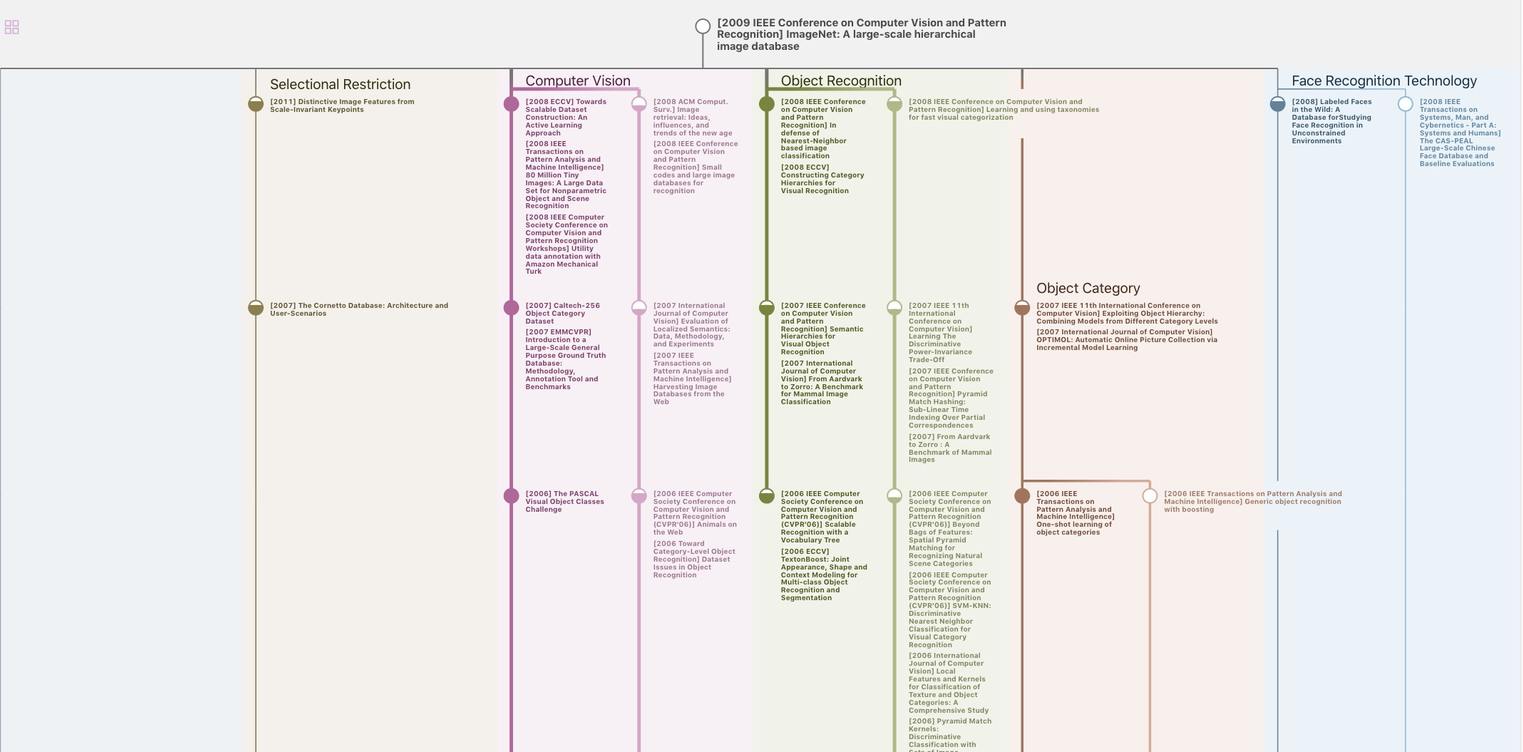
生成溯源树,研究论文发展脉络
Chat Paper
正在生成论文摘要