SAC3: Reliable Hallucination Detection in Black-Box Language Models via Semantic-aware Cross-check Consistency
EMNLP 2023(2023)
摘要
Hallucination detection is a critical step toward understanding the
trustworthiness of modern language models (LMs). To achieve this goal, we
re-examine existing detection approaches based on the self-consistency of LMs
and uncover two types of hallucinations resulting from 1) question-level and 2)
model-level, which cannot be effectively identified through self-consistency
check alone. Building upon this discovery, we propose a novel sampling-based
method, i.e., semantic-aware cross-check consistency (SAC3) that expands on the
principle of self-consistency checking. Our SAC3 approach incorporates
additional mechanisms to detect both question-level and model-level
hallucinations by leveraging advances including semantically equivalent
question perturbation and cross-model response consistency checking. Through
extensive and systematic empirical analysis, we demonstrate that SAC3
outperforms the state of the art in detecting both non-factual and factual
statements across multiple question-answering and open-domain generation
benchmarks.
更多查看译文
关键词
reliable hallucination detection,language models
AI 理解论文
溯源树
样例
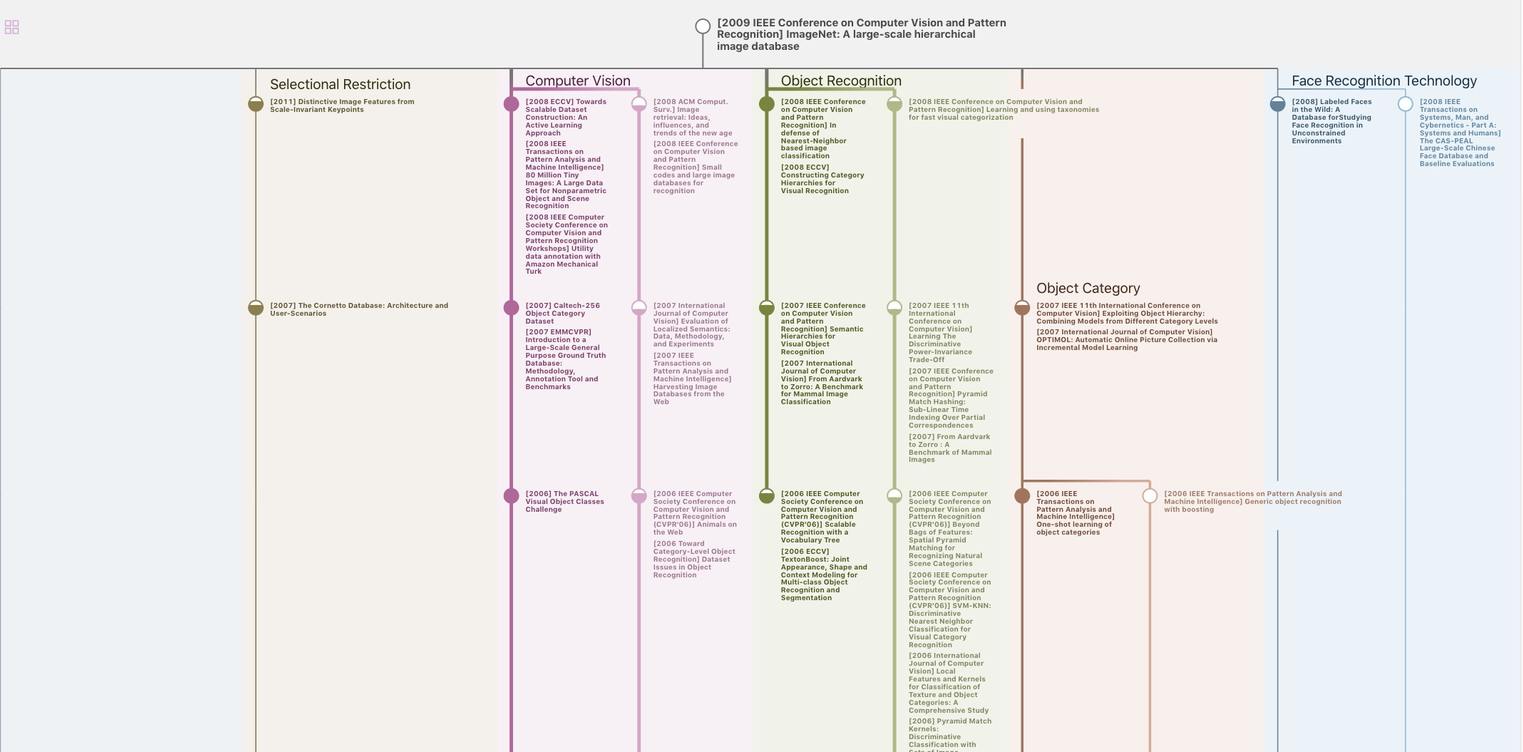
生成溯源树,研究论文发展脉络
Chat Paper
正在生成论文摘要