Look-Ahead Selective Plasticity for Continual Learning of Visual Tasks.
CoRR(2023)
摘要
Contrastive representation learning has emerged as a promising technique for continual learning as it can learn representations that are robust to catastrophic forgetting and generalize well to unseen future tasks. Previous work in continual learning has addressed forgetting by using previous task data and trained models. Inspired by event models created and updated in the brain, we propose a new mechanism that takes place during task boundaries, i.e., when one task finishes and another starts. By observing the redundancy-inducing ability of contrastive loss on the output of a neural network, our method leverages the first few samples of the new task to identify and retain parameters contributing most to the transfer ability of the neural network, freeing up the remaining parts of the network to learn new features. We evaluate the proposed methods on benchmark computer vision datasets including CIFAR10 and TinyImagenet and demonstrate state-of-the-art performance in the task-incremental, class-incremental, and domain-incremental continual learning scenarios.
更多查看译文
关键词
continual learning,visual tasks,look-ahead
AI 理解论文
溯源树
样例
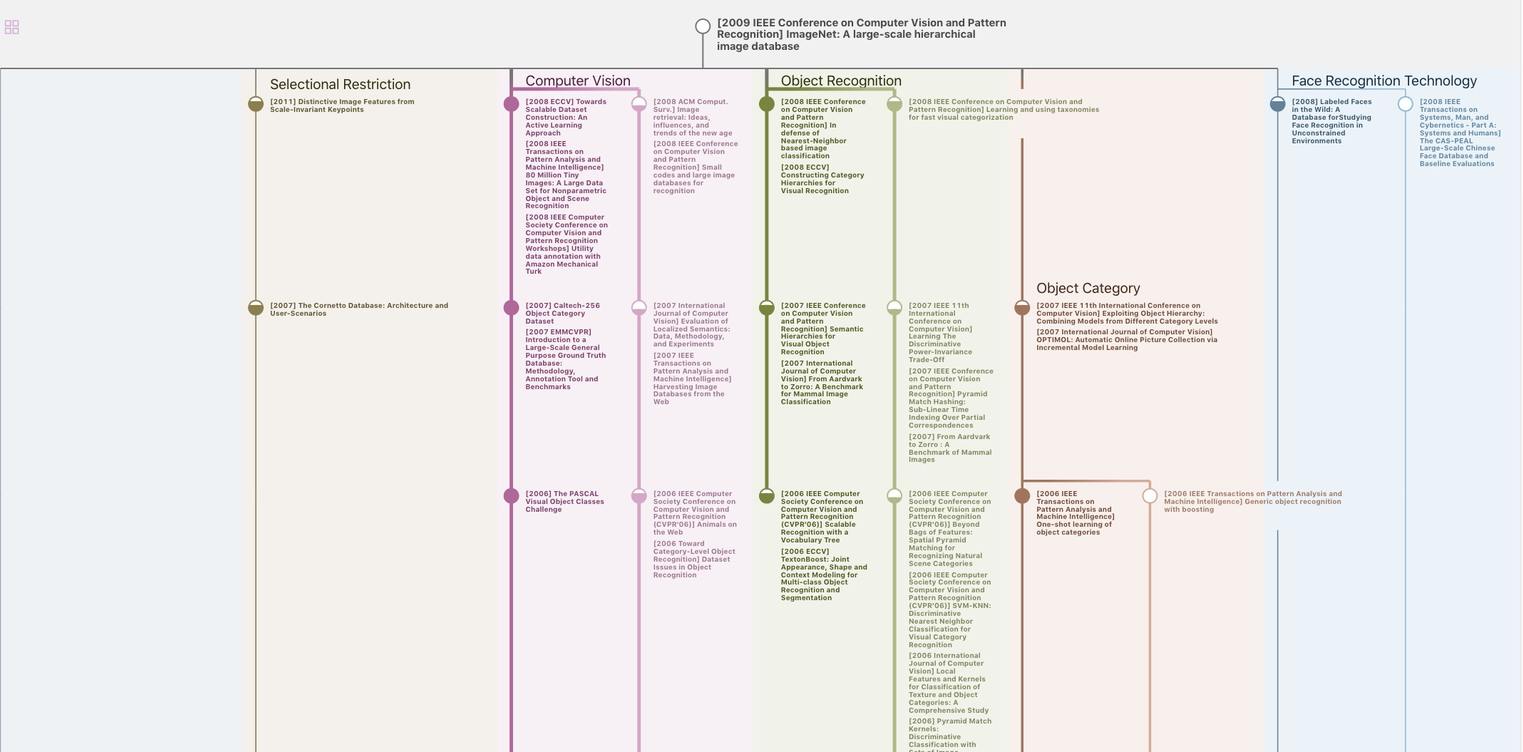
生成溯源树,研究论文发展脉络
Chat Paper
正在生成论文摘要