Adversarial Examples in the Physical World: A Survey.
CoRR(2023)
摘要
Deep neural networks (DNNs) have demonstrated high vulnerability to adversarial examples. Besides the attacks in the digital world, the practical implications of adversarial examples in the physical world present significant challenges and safety concerns. However, current research on physical adversarial examples (PAEs) lacks a comprehensive understanding of their unique characteristics, leading to limited significance and understanding. In this paper, we address this gap by thoroughly examining the characteristics of PAEs within a practical workflow encompassing training, manufacturing, and re-sampling processes. By analyzing the links between physical adversarial attacks, we identify manufacturing and re-sampling as the primary sources of distinct attributes and particularities in PAEs. Leveraging this knowledge, we develop a comprehensive analysis and classification framework for PAEs based on their specific characteristics, covering over 100 studies on physical-world adversarial examples. Furthermore, we investigate defense strategies against PAEs and identify open challenges and opportunities for future research. We aim to provide a fresh, thorough, and systematic understanding of PAEs, thereby promoting the development of robust adversarial learning and its application in open-world scenarios.
更多查看译文
关键词
adversarial examples,physical world
AI 理解论文
溯源树
样例
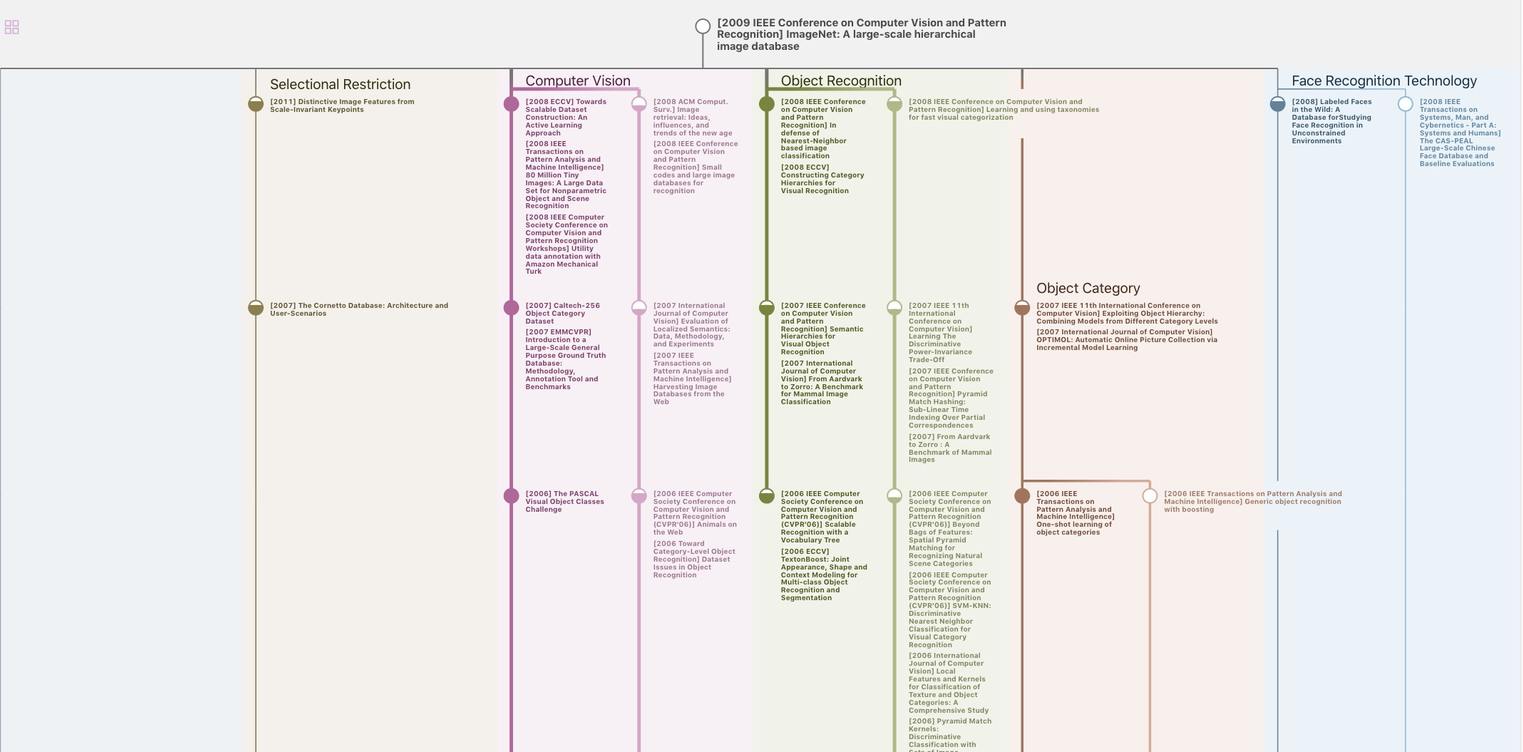
生成溯源树,研究论文发展脉络
Chat Paper
正在生成论文摘要