Acoustic inspired brain-to-sentence decoder for logosyllabic language
biorxiv(2024)
摘要
Many severe neurological diseases, such as stroke and amyotrophic lateral sclerosis, can impair or destroy the ability of verbal communication. Recent advances in brain-computer interfaces (BCIs) have shown promise in restoring communication by decoding neural signals related to speech or motor activities into text. Existing research on speech neuroprosthesis has predominantly focused on alphabetic languages, leaving a significant gap of logosyllabic languages such as Mandarin Chinese which are spoken by more than 15% of the world population. Logosyllabic languages pose unique challenges to brain-to-text decoding due to extended character sets (e.g., 50,000+ for Mandarin Chinese) and complex mapping between characters and pronunciation. To address these challenges, we established a speech BCI designed for Mandarin, decoding speech-related stereoelectroencephalography (sEEG) signals into coherent sentences. We leverage the unique acoustic features of Mandarin Chinese syllables, constructing prediction models for syllable components (initials, tones, and finals), and employ a language model to resolve pronunciation to character ambiguities according to the semantic context. This method leads to a high-performance decoder with a median character accuracy of 71.00% over the full character set, demonstrating huge potentials for clinical application. To our knowledge, we are the first to report brain-to-sentence decoding for logosyllabic languages over full character set with a large intracranial electroencephalography dataset.
### Competing Interest Statement
The authors have declared no competing interest.
更多查看译文
AI 理解论文
溯源树
样例
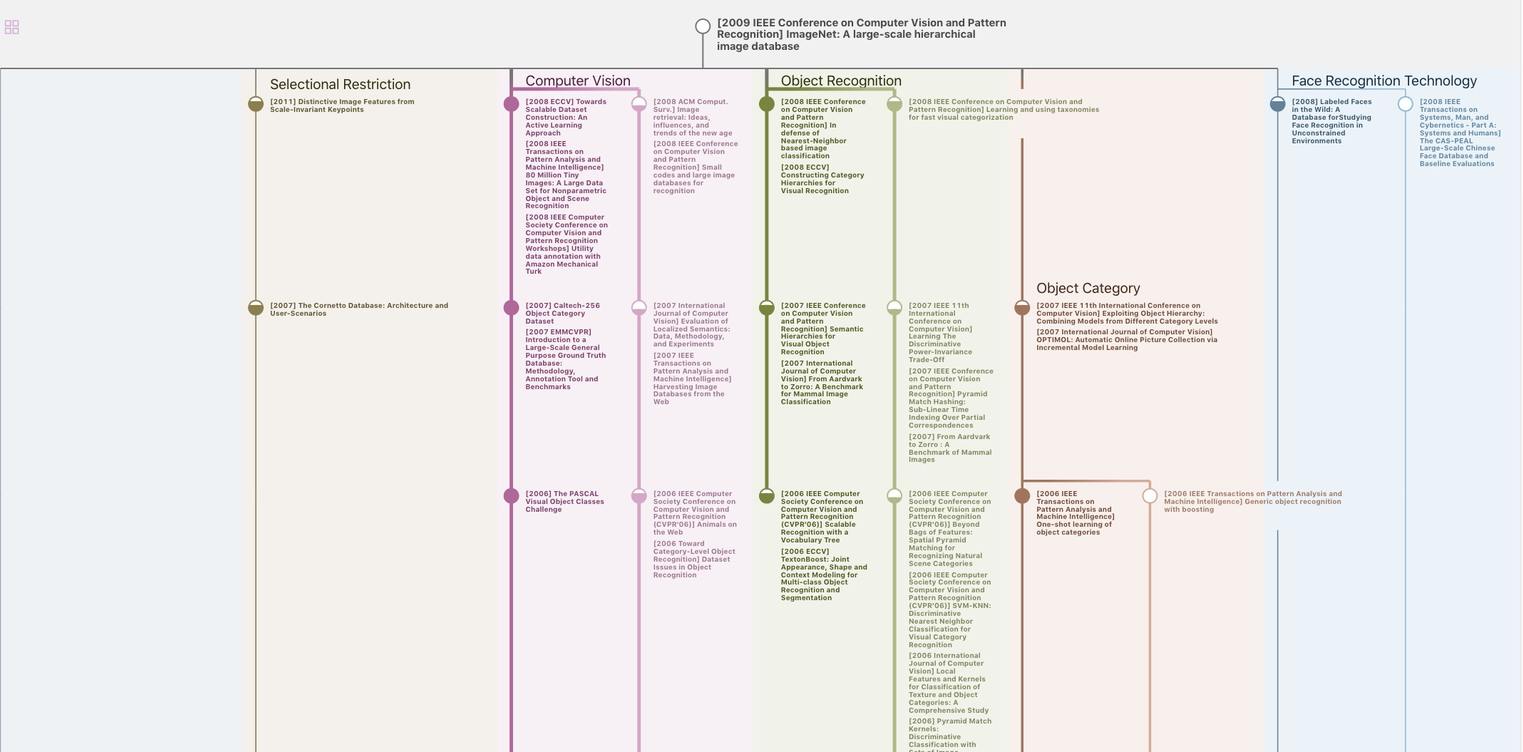
生成溯源树,研究论文发展脉络
Chat Paper
正在生成论文摘要