Reconstruction-based anomaly detection for multivariate time series using contrastive generative adversarial networks
INFORMATION PROCESSING & MANAGEMENT(2024)
摘要
The majority of existing anomaly detection methods for multivariate time series are based on Transformers and Autoencoders owing to their superior capabilities. However, these methods are susceptible to overfitting when there is insufficient data. To address this issue, we propose a novel unsupervised anomaly detection framework, which seamlessly integrates contrastive learning and Generative Adversarial Networks. More concretely, we utilize data augmentation techniques that incorporate geometric distribution masks to expand our training data, thereby enhancing its diversity. Then, a Transformer-based Autoencoder is trained within a Generative Adversarial Network framework to capture the underlying distribution for normal points. Additionally, we incorporate a contrastive loss into our discriminator to effectively regulate the GAN and ensure good generalization. Finally, anomalies are detected based on reconstruction errors. Numerous experiments on five real-world datasets demonstrated that our proposed method can effectively mitigates overfitting issues and obtains superior performance compared to state-of-the-art approaches. In particular, our model could achieve an average improvement of 9.28% in Precision, 11.33% in Recall, and 11.73% in F1-score.
更多查看译文
关键词
Anomaly detection,Multivariate time series,Generative adversarial networks,Contrastive learning
AI 理解论文
溯源树
样例
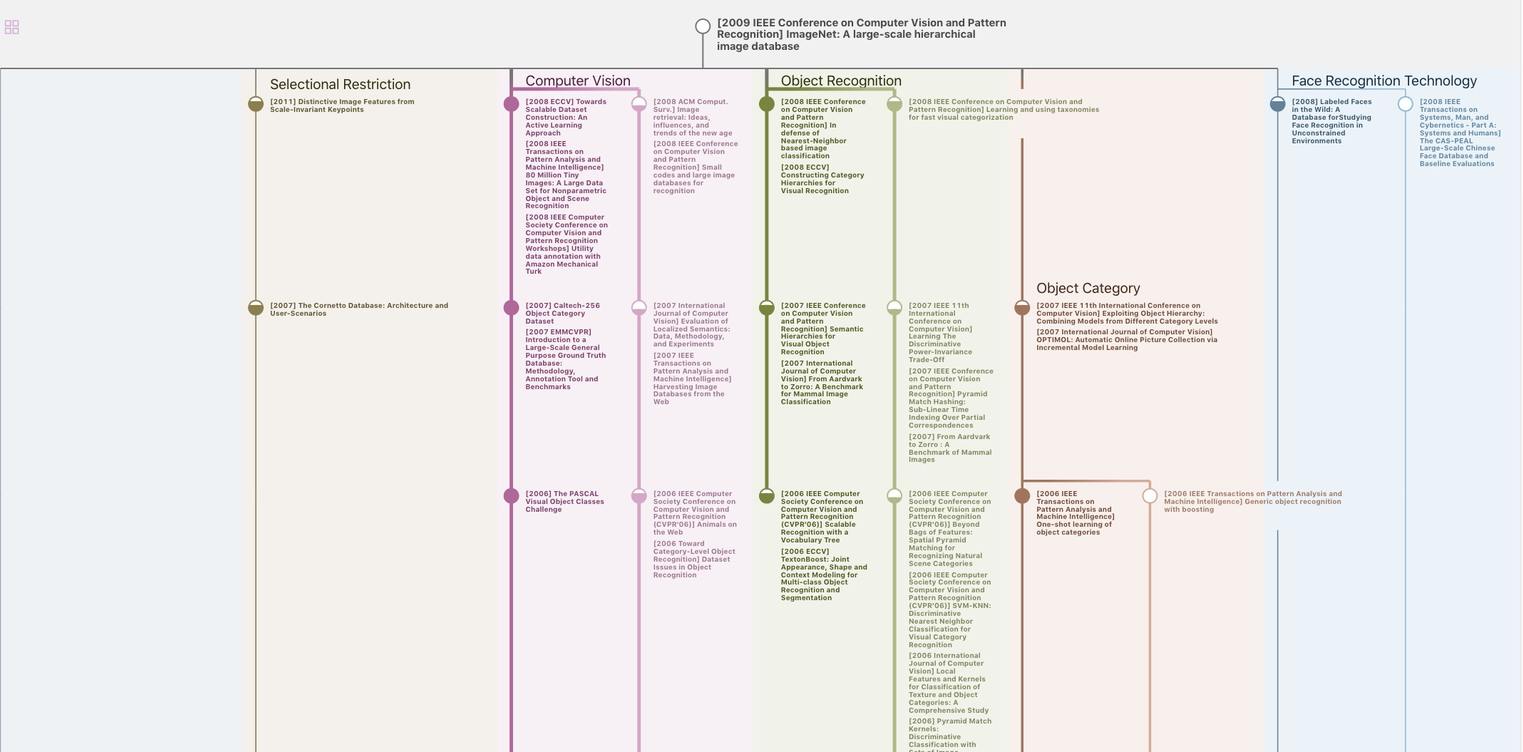
生成溯源树,研究论文发展脉络
Chat Paper
正在生成论文摘要