AdaNI: Adaptive Noise Injection to improve adversarial robustness
COMPUTER VISION AND IMAGE UNDERSTANDING(2024)
摘要
Deep Neural Networks (DNNs) have been proven vulnerable to adversarial perturbations, which narrow their applications in safe-critical scenarios such as video surveillance and autonomous driving. To counter this threat, a very recent line of adversarial defense methods is proposed to increase the uncertainty of DNNs via injecting random noises in both the training and testing process. Note the existing defense methods usually inject noises uniformly to DNNs. We argue that the magnitude of noises is highly correlated with the response of corresponding features and the randomness on important feature spots can further weaken adversarial attacks. As such, we propose a new method, namely AdaNI, which can increase feature randomness via Adaptive Noise Injection to improve the adversarial robustness. Compared to existing methods, our method creates non-unified random noises guided by features and then injects them into DNNs adaptively. Extensive experiments are conducted on several datasets (e.g., CIFAR10, CIFAR100, Mini-ImageNet) with comparisons to state-of-the-art defense methods, which corroborates the efficacy of our method against a variety of powerful white-box attacks (e.g., FGSM, PGD, C&W, Auto Attack) and black-box attacks (e.g., Transferable, ZOO, Square Attack). Moreover, our method is adapted to improve the robustness of DeepFake detection to demonstrate its applicability.
更多查看译文
关键词
Image classification,Adversarial examples,Adversarial robustness
AI 理解论文
溯源树
样例
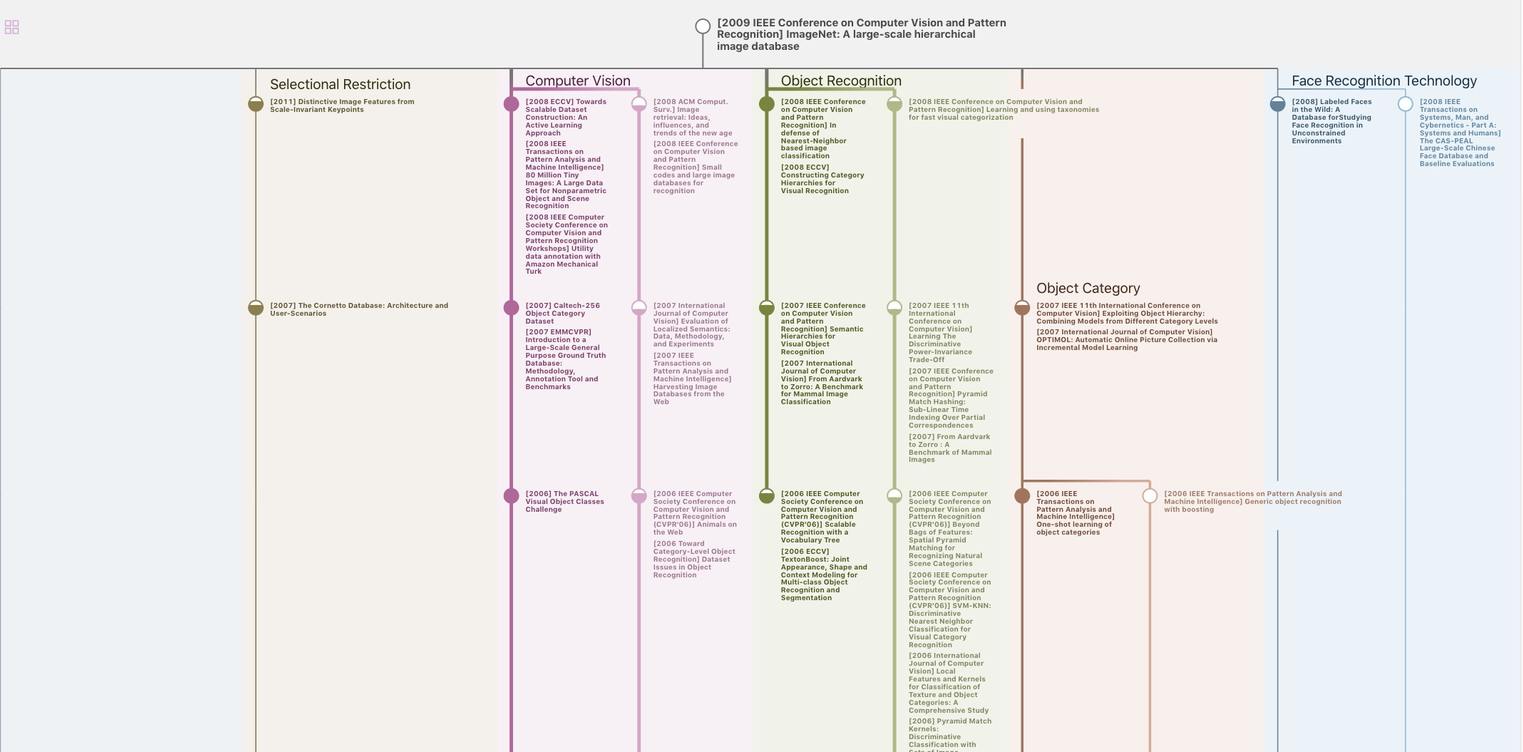
生成溯源树,研究论文发展脉络
Chat Paper
正在生成论文摘要