Particle segregation prediction method in drums combining data-driven and discrete element model.
2023 4th International Conference on Big Data & Artificial Intelligence & Software Engineering (ICBASE)(2023)
摘要
Combining data-driven model and discrete element method (DEM), the long-short term memory (LSTM) method was developed for predicting the flow characteristics of the particle inside rotary drums. In this work, the particle segregation characteristics in drums were first simulated by DEM models with different operating conditions. Then, LSTM model was constructed with the axial segregation and collision energy data generated by DEM simulations, with optimal model parameters determined by hyperparameter optimization. The results show that the predicted particle flow results are basically consistent with the DEM simulation, verifying the interpolation and extrapolation ability. Compared with traditional prediction methods, the LSTM model achieves the most accurate prediction results in dealing with tasks related to time series, which has the potential to rapidly optimize operational and equipment parameters in industrial applications.
更多查看译文
关键词
Keywords-Discrete element method,Data-driven model,Particle mixing and segregation,Prediction method,Rotary drums
AI 理解论文
溯源树
样例
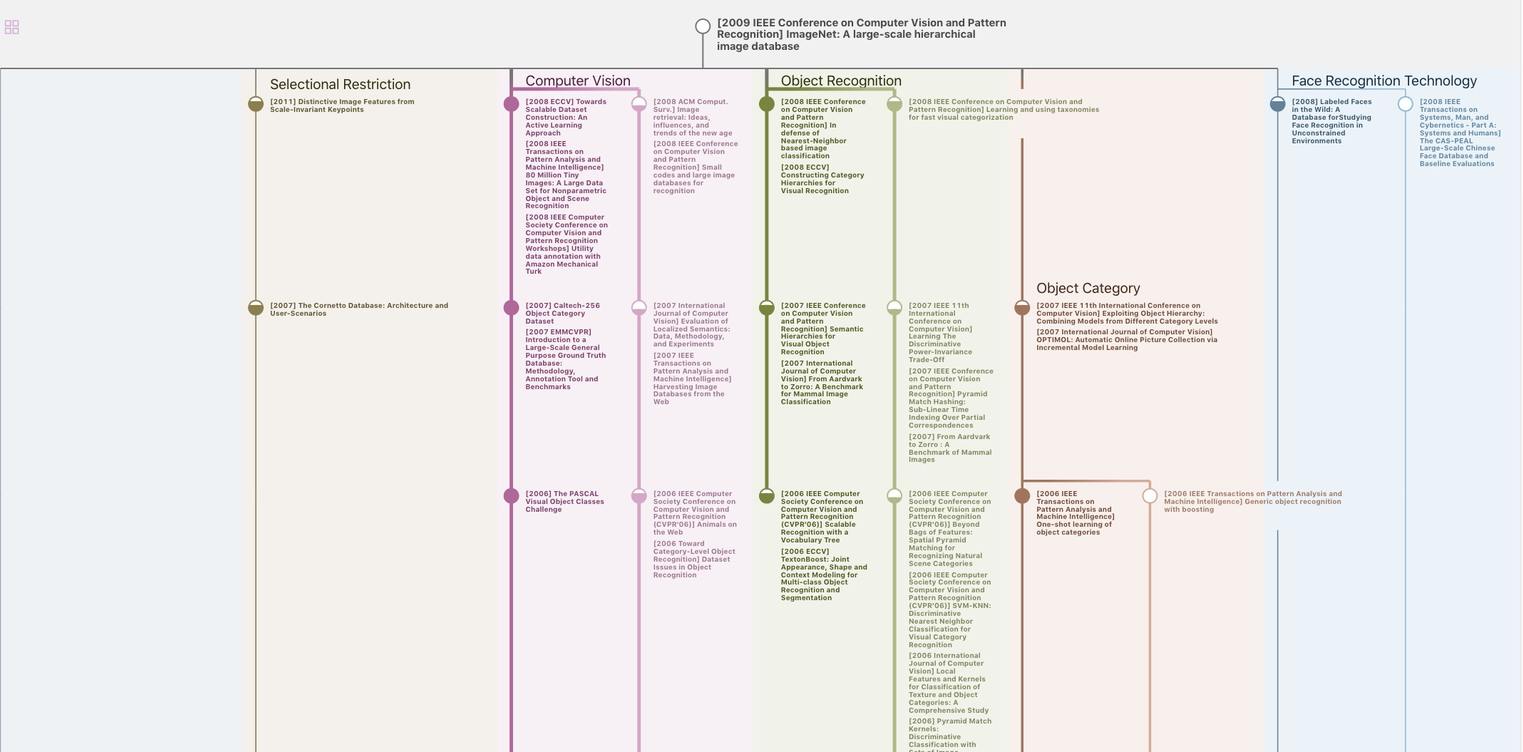
生成溯源树,研究论文发展脉络
Chat Paper
正在生成论文摘要