Contrasting changes in ozone during 2019-2021 between eastern and the other regions of China attributed to anthropogenic emissions and meteorological conditions
SCIENCE OF THE TOTAL ENVIRONMENT(2024)
摘要
Ozone pollution is one of the most severe air quality issues in China that poses a serious threat to human health and ecosystems. During 2019-2021, the maximum daily 8-h average ozone concentrations in eastern China (110-122.5 degrees E, 26-42 degrees N) and the rest of China (ROC) show different decreasing patterns, with ozone concentrations in eastern China decreasing by 14.9 mu g/m3, which is much larger than 4.8 mu g/m3 in ROC. Here, based on two independent methods, the atmospheric chemical transport model (GEOS-Chem) simulations and the machine learning (ML) model (LightGBM) predictions, the reasons for the differences in ozone changes between eastern China and ROC during the warm season (April to September) are investigated. According to the GEOSChem (LightGBM) results, changes in the meteorological conditions contributed to an ozone decrease by 7.3 (6.8) mu g/m3 in eastern China due to decreased chemical production and an ozone decrease by 6.8 (7.0) mu g/m3 in ROC attributed to the weakened horizontal and vertical advection. With the influence of meteorological factors excluded, the observations show that changes in anthropogenic emissions resulted in an ozone decrease by 7.6 (8.1) mu g/m3 in eastern China and an ozone increase by 2.0 (2.2) mu g/m3 in ROC, which is primarily induced by the changes in NOx emissions. The surface measurements and satellite retrievals also indicate that the reduction in NOx emissions in ROC is less efficient than that in the more developed eastern China, leading to contrasting changes in ozone concentrations between eastern China and ROC during 2019-2021. Our results highlight the critical need to reduce ozone precursor emissions in the rest regions of China apart from eastern China.
更多查看译文
关键词
Ozone,Emission,GEOS-Chem,Machine learning,Meteorology
AI 理解论文
溯源树
样例
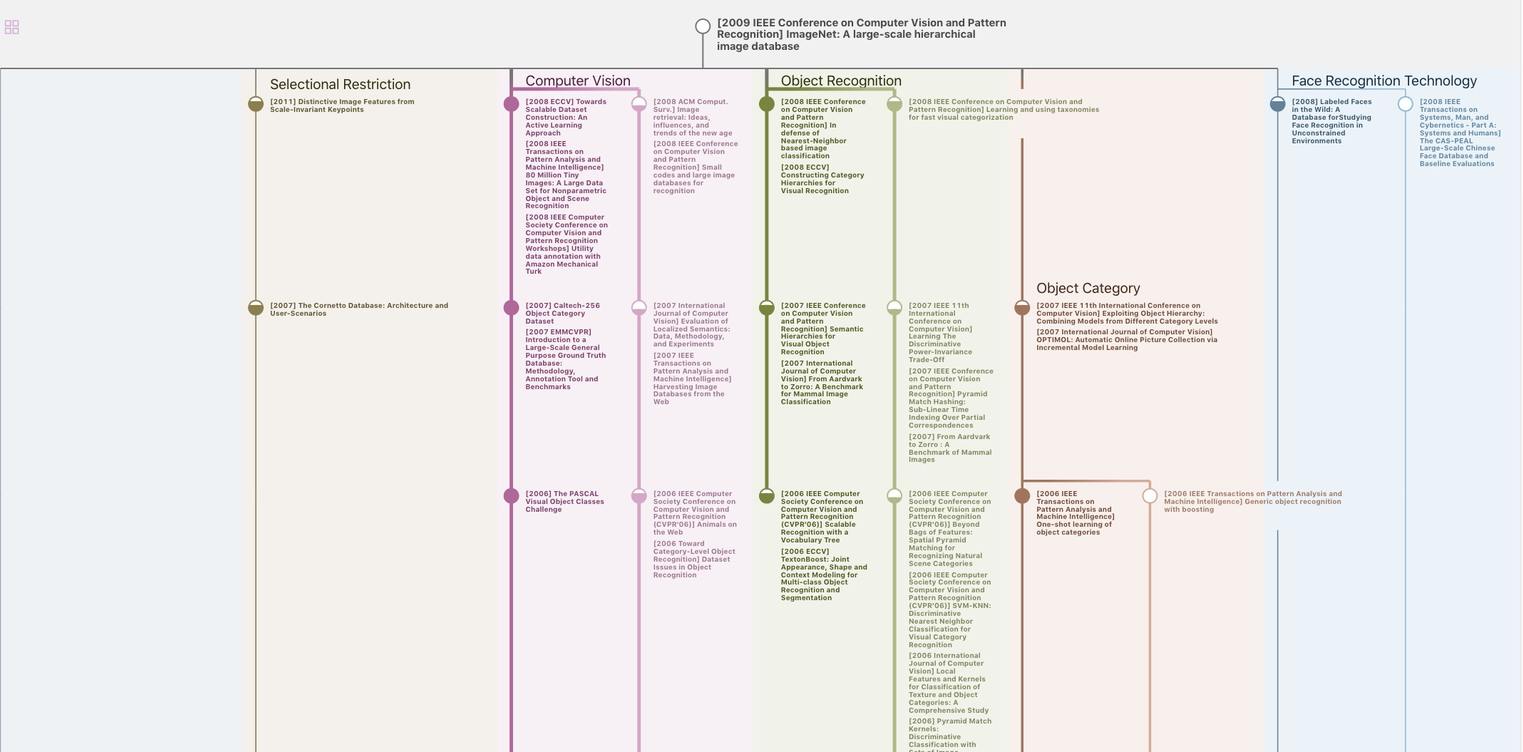
生成溯源树,研究论文发展脉络
Chat Paper
正在生成论文摘要