An Extremely Lightweight Network with Blueprint Separable Convolution and Pruning for Diagnosing High-speed Train Bogie Faults
2023 CAA Symposium on Fault Detection, Supervision and Safety for Technical Processes (SAFEPROCESS)(2023)
摘要
The high-speed train bogie has complex structures, various conditions, and high maintenance demands. As a result, an extremely lightweight network (ELN) is proposed in this paper for HST fault diagnosis and may be employed in hand-held terminals. Firstly, a lightweight architecture based on blueprint separable convolution (BSConv) is designed as a feature extractor. Secondly, the mask mechanism is introduced to temporarily deactivate low-weight neurons in order to further diminish network size. Finally, the optimal network is fixed and deployed after learning and evaluation. Experiments prove that ELN has an extremely lightweight structure as well as high failure recognition accuracy.
更多查看译文
关键词
Fault diagnosis,high-speed train,bogie,lightweight network
AI 理解论文
溯源树
样例
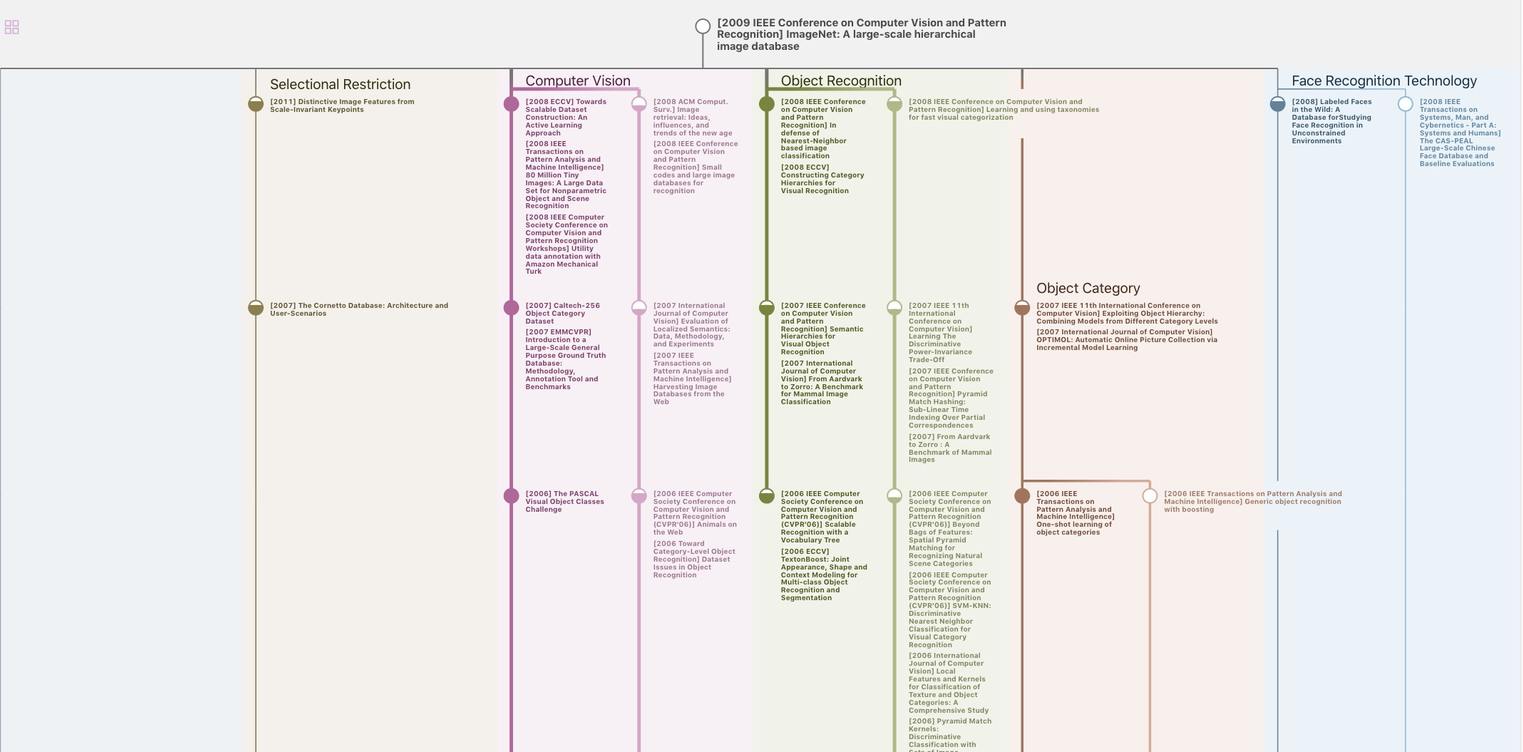
生成溯源树,研究论文发展脉络
Chat Paper
正在生成论文摘要