Sequential reversible jump MCMC for dynamic Bayesian neural networks
NEUROCOMPUTING(2024)
摘要
The challenge to automatically design machine learning-based models for datasets with varying dimensions (features) remains open, especially in the context of unstructured and noisy data. Bayesian neural networks employ Markov chain Monte Carlo (MCMC) and variational inference methods for training (sampling) model parameters. However, the progress of MCMC methods in deep learning has been slow due to high computa-tional requirements and uninformative priors of model parameters. Reversible jump MCMC allows sampling of model parameters of variable lengths; hence, it has the potential to train Bayesian neural networks effectively. In this paper, we implement reversible jump MCMC sampling for training dynamic Bayesian neural networks that feature cascaded neural networks with dynamic hidden and input neurons. Our dynamic Bayesian neural network can model unstructured data of varying dimensions and provide uncertainty quantification in model predictions. We apply the methodology to selected regression and classification problems from the literature. The results show that our method provides an effective approach for the dynamic exploration of models while featuring uncertainty quantification that not only caters to model parameters but also extends to model topology. This opens up the road for uncertainty quantification in dynamic deep learning models for dynamic and unstructured data streams.
更多查看译文
关键词
Deep learning,Reversible jump
AI 理解论文
溯源树
样例
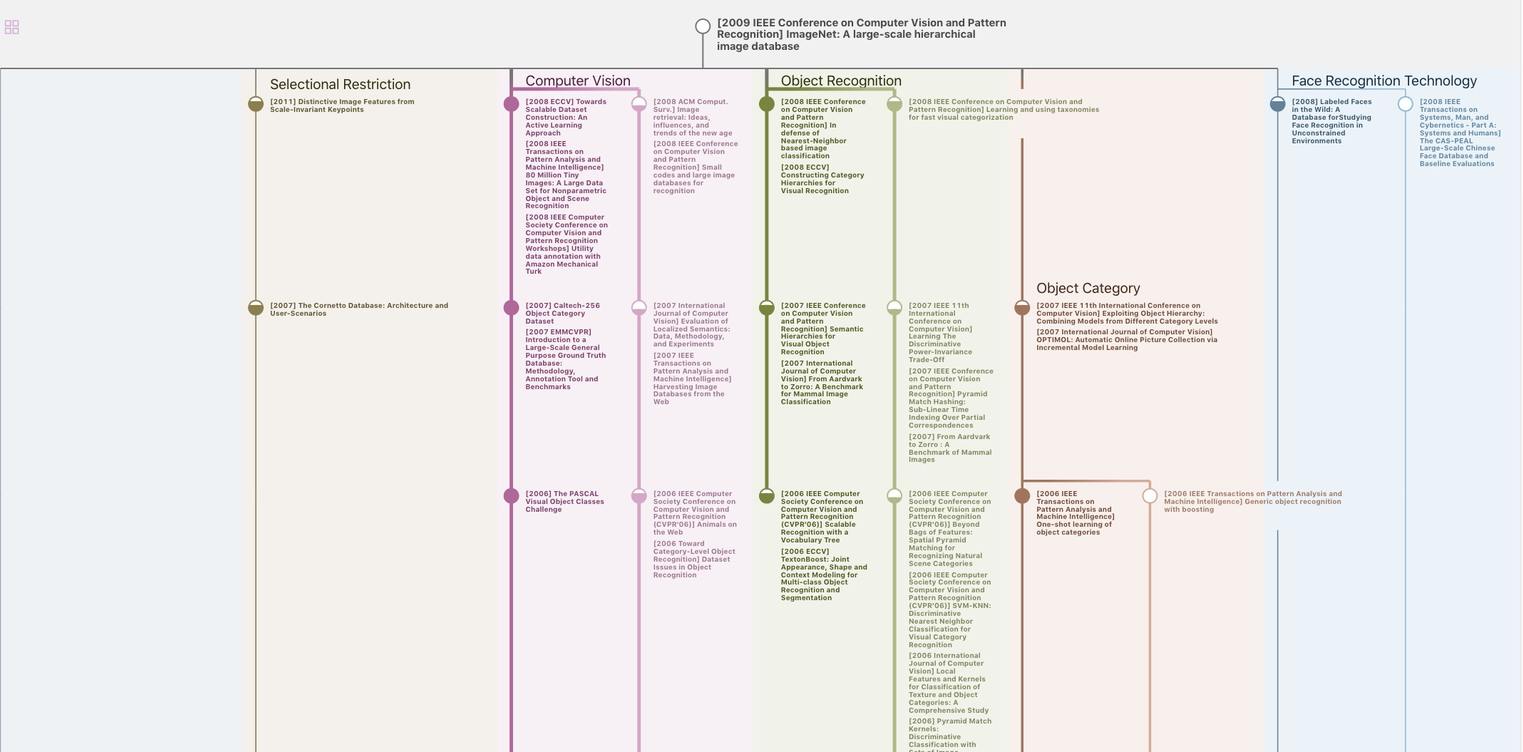
生成溯源树,研究论文发展脉络
Chat Paper
正在生成论文摘要