An attention model with multiple decoders for solving p-Center problems
INTERNATIONAL JOURNAL OF APPLIED EARTH OBSERVATION AND GEOINFORMATION(2023)
摘要
The p-Center Problem (PCP) is a classical discrete facility location problem (FLP) with broad real-world application scenarios, such as public facilities and urban emergency services facilities. Most of the current methods for solving PCP are exact and heuristic. However, as the problem conditions are added or the problem scale is increased, the complexity of exact and heuristic methods for solving PCP increases significantly, which is reflected in the exponentially increased solving time and declined accuracy, respectively. Therefore, this research experiments with an attentional model with multiple decoders for solving the p-Center problem (AMMD-PC), considering different real-world contexts. AMMD-PC learns the construction heuristics with the deep reinforcement learning method, adopting an encoder-multiple decoder structure. The multi-head self-attention mechanism in the encoder learns the feature representation of the candidate facility nodes in different sub-spaces. Multiple decoders train the construction strategy of solutions separately to increase the diversity of solutions so as to find high-quality solutions. Experiments on PCPs of different scales and conditions show that AMMD-PC is superior to traditional heuristic methods in solving time and quality. In addition, the advantages of the AMMD-PC become more pronounced as the complexity of the problem increases to the point where the exact solver cannot compute the optimal solution in an acceptable time.
更多查看译文
关键词
Facility location problem,p-Center problem,Deep reinforcement learning,Attention model,Multiple decoders
AI 理解论文
溯源树
样例
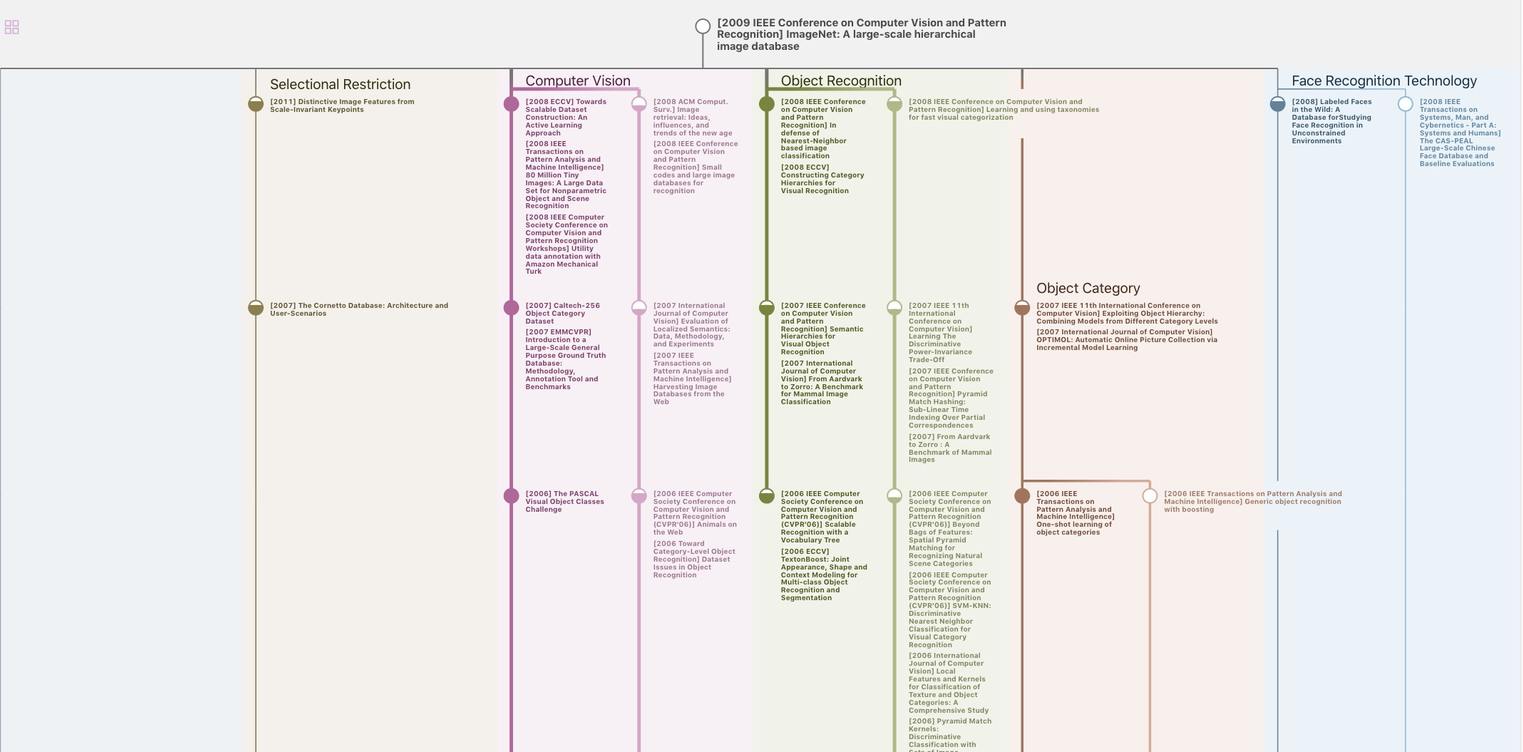
生成溯源树,研究论文发展脉络
Chat Paper
正在生成论文摘要