Stabilization temperature prediction in carbon fiber production using empirical mode decomposition and long short-term memory network
JOURNAL OF CLEANER PRODUCTION(2023)
摘要
Carbon fiber holds significant promise as a sustainable material with diverse applications. The production of carbon fiber involves a critical process known as oxidative stabilization. Optimizing this process necessitates accurate prediction of the stabilization temperature. However, predicting the stabilization temperature based on easily available heater power data encounters formidable challenges due to factors like nonlinearity, nonuniformity, time delay and time-varying effect. To address these issues, this study proposed an innovative method for predicting the stabilization temperature using empirical mode decomposition (EMD) and long shortterm memory (LSTM) network. The distinctive aspect of this proposed method lies in the utilization of two EMDbased reconstruction approaches to preprocess the heater power training and testing data. This helps mitigate the nonlinearity between stabilization temperature and heater power output during the machine learning network training phase and alleviate the time-varying effect of heater power testing data relative to the training data during the network testing phase. Considering the hysteresis effect of heater power output on stabilization temperature, an LSTM network was integrated for accurate stabilization temperature prediction. The proposed EMD-LSTM network model was implemented for stabilization temperature predictions in six oxidation ovens of a carbon fiber production line. The average metrics for predicted results using this method are notably superior, with values of 0.0153 degrees C for root-mean-square error (RMSE), 0.3711 for relative RMSE (rRMSE), 0.0117 degrees C for mean absolute error (MAE), and 0.2838 for relative MAE (rMAE), surpassing the results obtained from the LSTM network model trained and tested using unreconstructed data. The comparative analysis further demonstrates the advantageous performance of the proposed EMD-LSTM network model compared to models based on five widely used machine learning networks. This study offers valuable insights for optimizing the oxidative stabilization process in carbon fiber production, thereby reducing waste heat and exhaust emissions, and promoting cleaner carbon fiber production.
更多查看译文
关键词
Carbon fiber,Stabilization temperature,Prediction,Empirical mode decomposition,Long short-term memory network
AI 理解论文
溯源树
样例
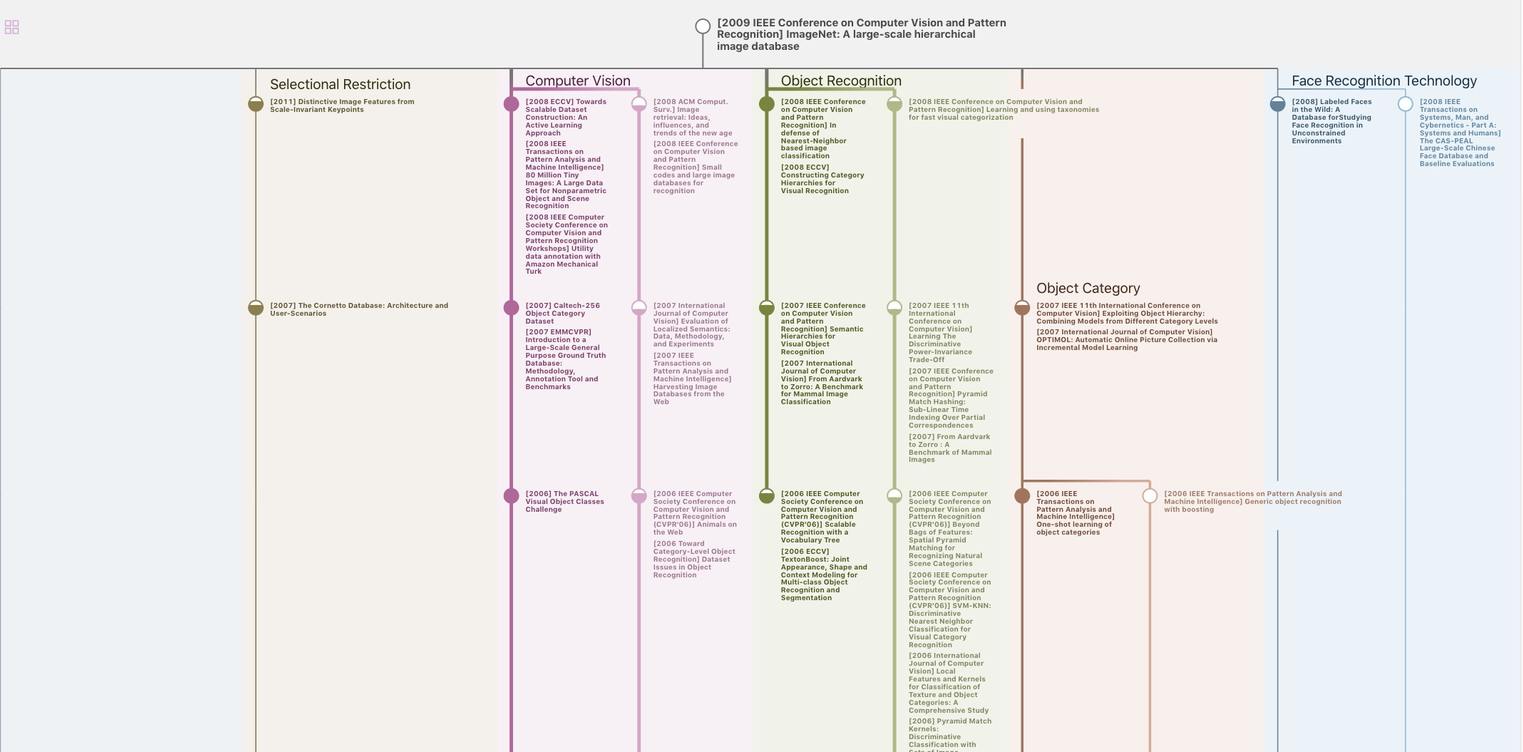
生成溯源树,研究论文发展脉络
Chat Paper
正在生成论文摘要