InFit: Combination Movement Recognition for Intensive Fitness Assistant via Wi-Fi
IEEE TRANSACTIONS ON MOBILE COMPUTING(2023)
摘要
Wi-Fi technology is becoming a promising enabler of device-free fitness tracking to provide reviews and recommendations for effective homely exercise. State-of-the-art Wi-Fi fitness assistants succeed in recognizing the simple meta-movements (e.g., Push-Up and Squat) with discrete and repeatable patterns. Unfortunately, these prior attempts can hardly scale to the combination movements of ever-growing interests in intensive fitness programs. Combination movements are composed of meta-movements that are mutually concatenated or inserted. They have a compound characteristic that inherits from the diversity of combination orders and continuity of meta-movements. The compound characteristic causes substantial training data collection costs and a challenge of combination decomposition that is a prerequisite for providing fine-grained fitness assessment. To this end, we propose InFit, a Wi-Fi-based device-free fitness assistant system for combination movements. First, we design a novel data augmentation method, namely Stitching-based Virtual Sample Generation (SVSG), to reduce the training data collection costs by generating virtual combination movements. Second, a 2-stage combination movement recognition model is designed to learn temporal dependencies between movements and decompose combination movements. From its outputs, we can tell whether a combination movement is standard. Extensive experimental results show that InFit can achieve an average recognition accuracy of 94%. With zero training samples of combination movements, the average accuracy is 40% higher than the baselines. In addition, SVSG can provide a general enhancement on multiple competing schemes with similar sensing tasks.
更多查看译文
关键词
Fitness assistant,combination movement,meta-movement,virtual sample generation,Wi-Fi
AI 理解论文
溯源树
样例
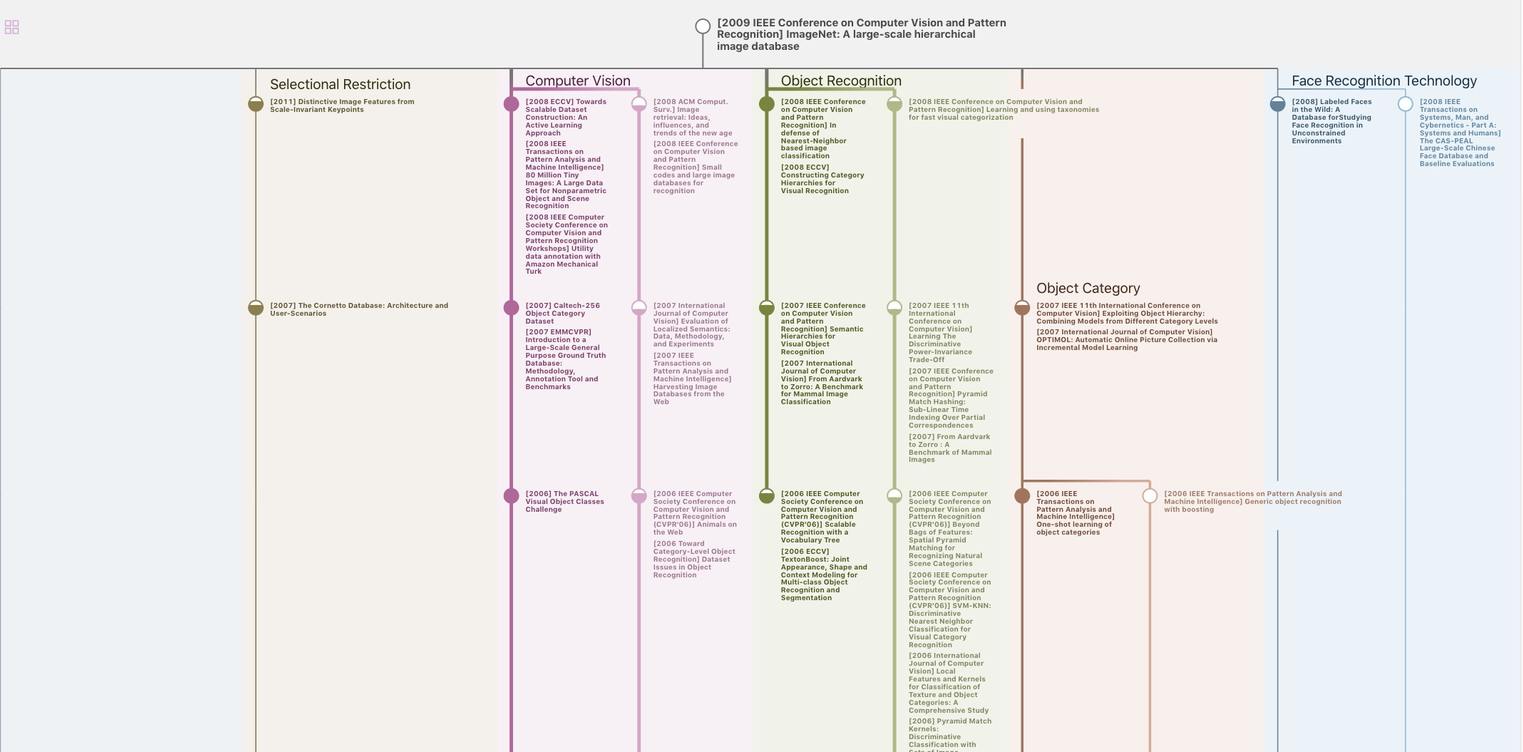
生成溯源树,研究论文发展脉络
Chat Paper
正在生成论文摘要