Multi-objective evolutionary optimization of extreme gradient boosting regression models of the internal turning of PEEK tubes
EXPERT SYSTEMS WITH APPLICATIONS(2024)
摘要
Internal turning or boring is an attractive machining process for hole enlarging as it presents low costs, good flexibility, and adaptability. The process presents some instability problems due to the high relation length/diameter ratio of the boring bar. To achieve the best process conditions, predictive models must be estimated and optimization must be conducted. This work presents a statistical learning approach for modeling and optimization of the internal turning process in PEEK tubes. Cutting speed, feed rate, and fixture position were considered input parameters. Cross-validation is used for learning and model selection, including k-fold and bootstrap approaches. The results pointed out that the extreme gradient boosting model was the best for all predictors. For R-a the final prediction metrics results were RMSE = 0.1395, MEA = 0.1126, and R-2 = 1.0031, for F-c, RMSE = 1.8609, MAE = 0.9311, and R-2 = 0.9280, and for Ron(t), RMSE = 21.3084, MAE = 17.8053, and R-2 = 0.6562. These results were much superior to the other methods. Multi-objective evolutionary optimization was performed considering the extreme gradient boosting models for roughness, total roundness, and cutting force, besides the deterministic model of the material removal rate. The NSGA-II method was selected considering the hypervolume metric. The pseudo-weight approach is used for select high trade-off solutions to be used in practical production scenarios.
更多查看译文
关键词
Internal turning,Polyetheretherketone,Statistical learning,Machine learning,Extreme gradient boosting,Multi-objective evolutionary optimization
AI 理解论文
溯源树
样例
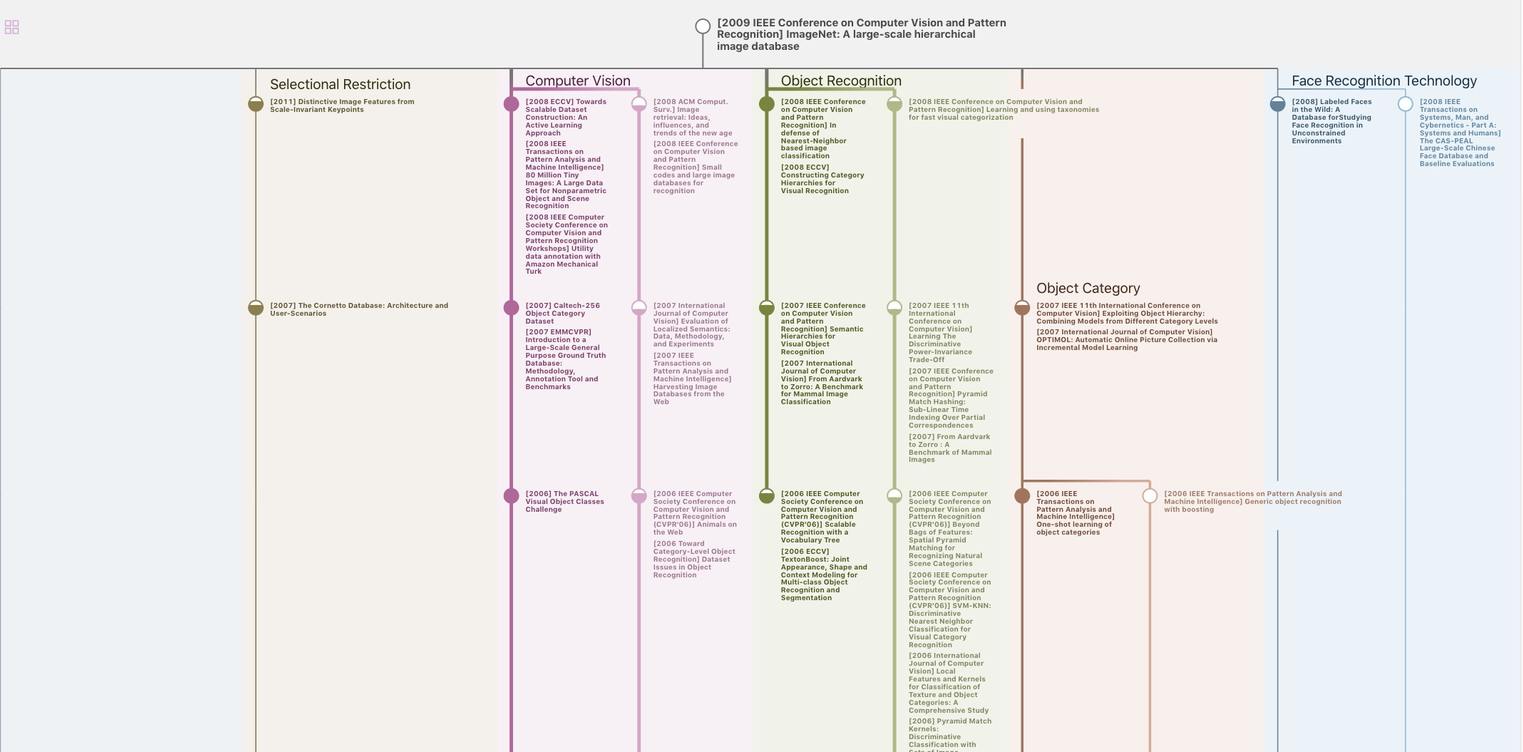
生成溯源树,研究论文发展脉络
Chat Paper
正在生成论文摘要