MLapRVFL: Protein sequence prediction based on Multi-Laplacian Regularized Random Vector Functional Link
COMPUTERS IN BIOLOGY AND MEDICINE(2023)
摘要
Protein sequence classification is a crucial research field in bioinformatics, playing a vital role in facilitating functional annotation, structure prediction, and gaining a deeper understanding of protein function and interactions. With the rapid development of high-throughput sequencing technologies, a vast amount of unknown protein sequence data is being generated and accumulated, leading to an increasing demand for protein classification and annotation. Existing machine learning methods still have limitations in protein sequence classification, such as low accuracy and precision of classification models, rendering them less valuable in practical applications. Additionally, these models often lack strong generalization capabilities and cannot be widely applied to various types of proteins. Therefore, accurately classifying and predicting proteins remains a chal-lenging task. In this study, we propose a protein sequence classifier called Multi-Laplacian Regularized Random Vector Functional Link (MLapRVFL). By incorporating Multi-Laplacian and L2,1 -norm regularization terms into the basic Random Vector Functional Link (RVFL) method, we effectively improve the model's generalization performance, enhance the robustness and accuracy of the classification model. The experimental results on two commonly used datasets demonstrate that MLapRVFL outperforms popular machine learning methods and achieves superior predictive performance compared to previous studies. In conclusion, the proposed MLapRVFL method makes significant contributions to protein sequence prediction.
更多查看译文
关键词
Predict protein sequence,Protein sequence classifier,Multi-laplacian regularization terms,L21-norm,MLapRVFL,RVFL
AI 理解论文
溯源树
样例
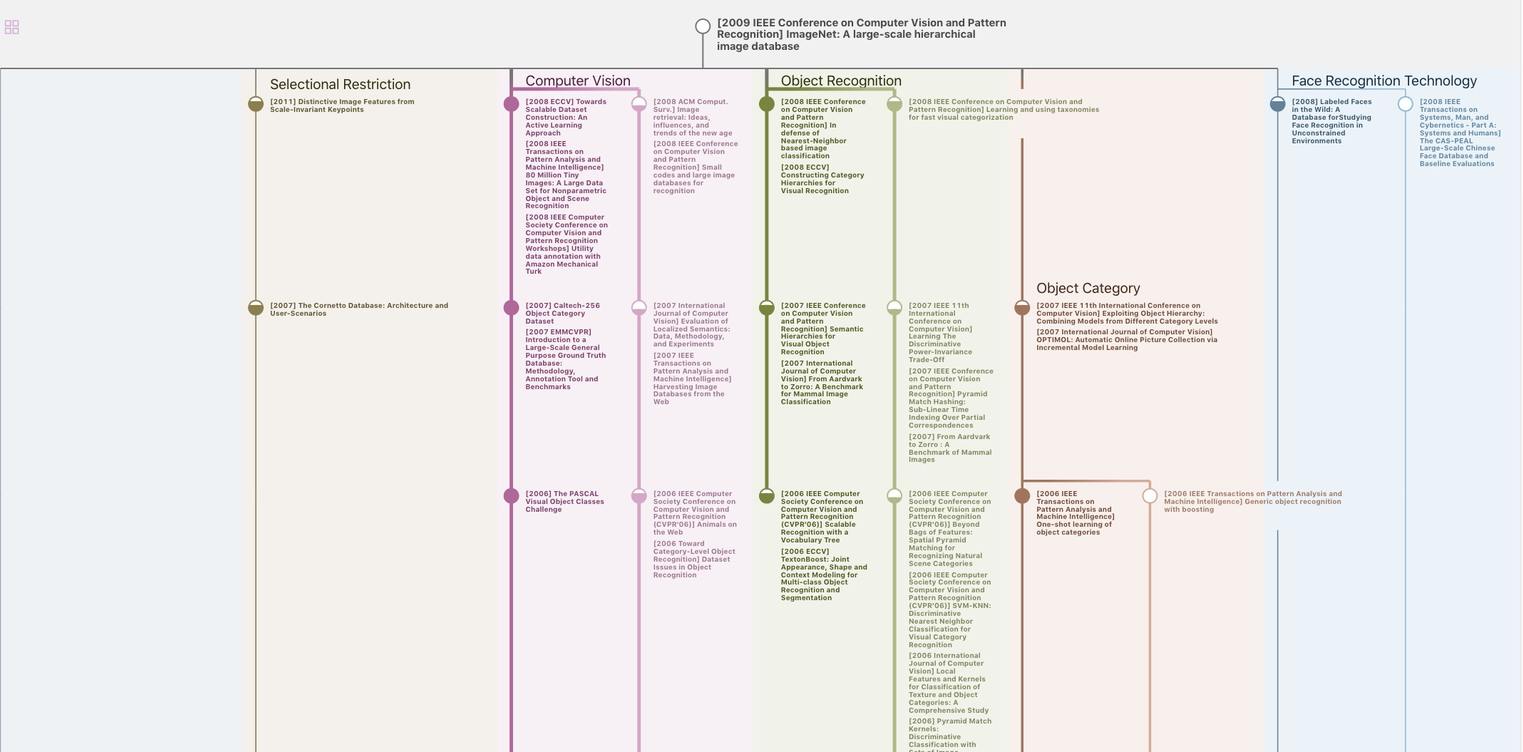
生成溯源树,研究论文发展脉络
Chat Paper
正在生成论文摘要