Protocol for hunting PM
EnvSys '23: Proceedings of the 1st International Workshop on Advances in Environmental Sensing Systems for Smart Cities(2023)
摘要
Particulate Matter (PM) is a major air pollutant that has the potential for adversely affecting human health. Actionable data on the spatial distribution of temporal variability of PM 2.5 emission hot spots in large cities are sparse. The main objective of this research is to provide a protocol for using search agents to hunt for PM 2.5 emission hot spots in urban environments. We propose short range identification of variability of harmful PM 2.5 concentrations can be achieved using IoT devices mounted on a mobile platform. We propose that long range identification the PM 2.5 emission hot spots can attained by searching through the city on different days. We applied this approach to Hyderabad, India by fixing a mobile platform on a street car. We corrected the IoT device measurement errors by calibrating the sensing component data against a reference instrument co-located on the mobile platform. We identified that random forest regression was the most suitable technique to reduce the variability between the IoT devices. The spatial variability of PM 2.5 harmful emission hot spots at industrial settings and congested roads were identified. The temporal variability based on image processing shows a weak correlation between PM 2.5 concentrations and number of vehicles, and PM 2.5 and visibility. The Hyderabad PM 2.5 emission hot spots findings demonstrate a clear need to inform people with heart and lung conditions when it is unhealthy to be outside; and when it is unhealthy for children and elderly people to be outside for prolonged periods. Our emission hunting approach can be applied to any mobile platform carried by people walking, cycling or by drones and robots in any city.
更多查看译文
AI 理解论文
溯源树
样例
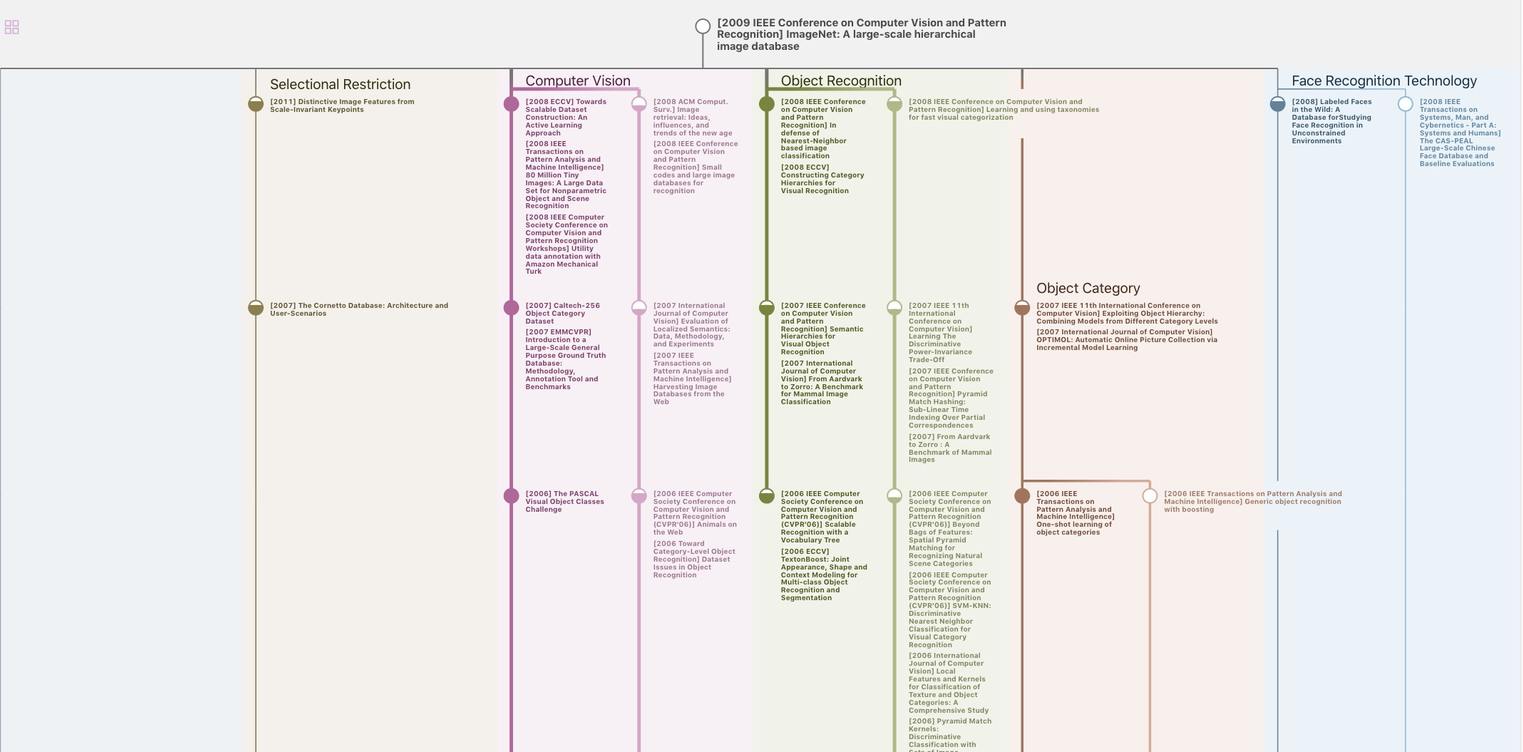
生成溯源树,研究论文发展脉络
Chat Paper
正在生成论文摘要