Shifted GCN-GAT and Cumulative-Transformer based Social Relation Recognition for Long Videos
MM '23: Proceedings of the 31st ACM International Conference on Multimedia(2023)
摘要
Social Relation Recognition is an important part of Video Understanding, providing insights into the information that videos convey. Most previous works mainly focused on graph generation for characters, instead of edges which are more suitable for relation modelling. Furthermore, previous methods tend to recognize social relations for single frames or short video clips within their receptive fields, neglecting the importance of continuous reasoning throughout the entire video. To tackle these challenges, we propose a novel Shifted GCN-GAT and Cumulative-Transformer framework, named SGCAT-CT. The overall architecture consists of an SGCAT module for shifted graph operations on novel relation graphs and a CT module for temporal processing with memory. SGCAT-CT conducts continuous recognition of social relations and memorizes information from as early as the beginning of a long video. Experiments conducted on several video datasets demonstrate encouraging performance on long videos. Our code will be released at https://github.com/HarryWgCN/SGCAT-CT.
更多查看译文
AI 理解论文
溯源树
样例
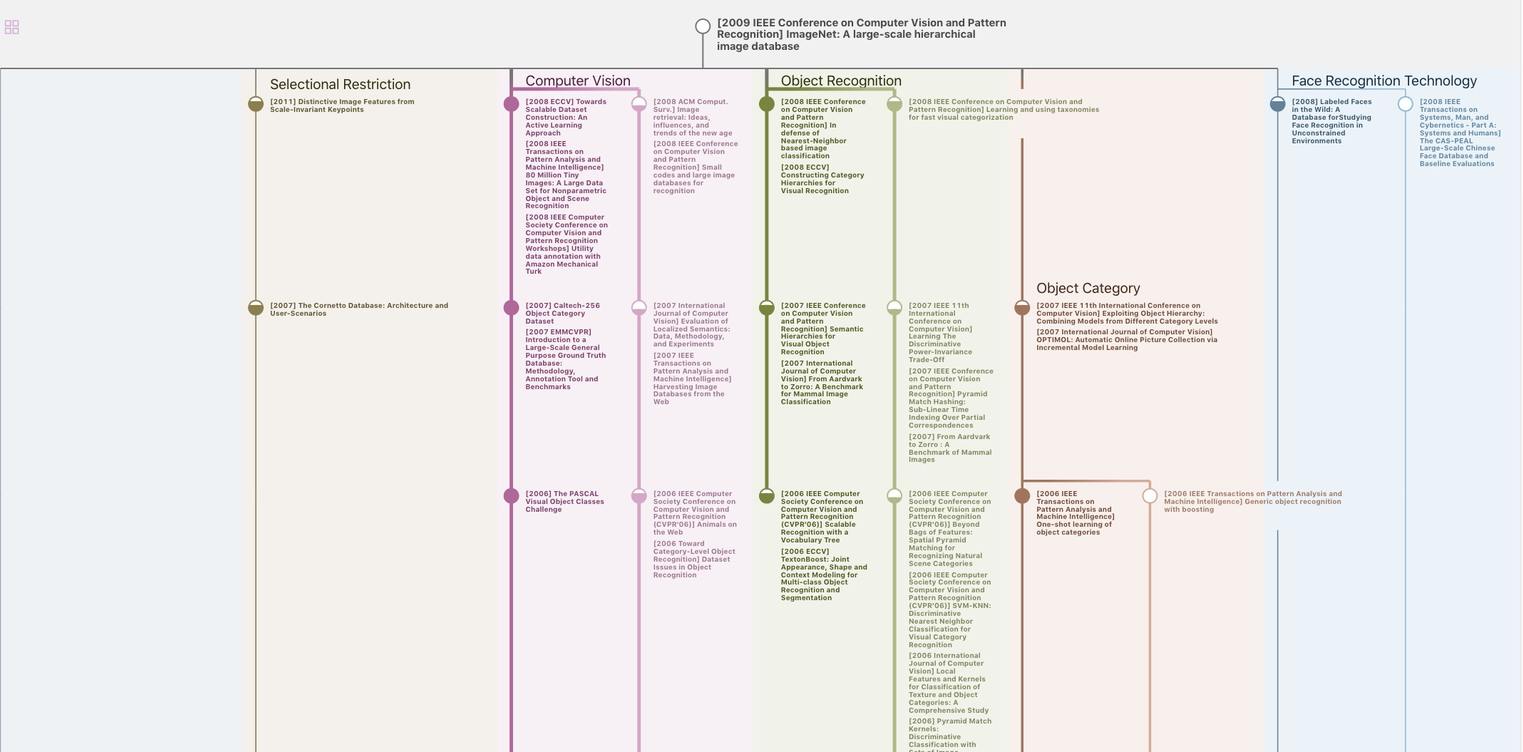
生成溯源树,研究论文发展脉络
Chat Paper
正在生成论文摘要