Informative Classes Matter: Towards Unsupervised Domain Adaptive Nighttime Semantic Segmentation
MM '23: Proceedings of the 31st ACM International Conference on Multimedia(2023)
摘要
Unsupervised Domain Adaptive Nighttime Semantic Segmentation (UDA-NSS) aims to adapt a robust model from a labeled daytime domain to an unlabeled nighttime domain. However, current advanced segmentation methods ignore the illumination effect and class discrepancies of different semantic classes during domain adaptation, showing an uneven prediction phenomenon. It is the completely ignored and underexplored issues of ''hard-to-adapt'' classes that some classes have a large performance gap between existing UDA-NSS methods and supervised learning counterparts while others have a very low performance gap. To realize ''hard-to-adapt'' classes' more sufficient learning and facilitate the UDA-NSS task, we present an Online Informative Class Sampling (OICS) strategy to adaptively mine informative classes from the target nighttime domain according to the corresponding spectrogram mean and the class frequency via our Informative Mixture of Experts. Furthermore, an Informativeness-based cross-domain Mixed Sampling (InforMS) framework is designed to focus on informative classes from the target nighttime domain by vesting their higher sampling probabilities when cross-domain mixing sampling and achieves better performance in UDA-NSS tasks. Consequently, our method outperforms state-of-the-art UDA-NSS methods by large margins on three widely-used benchmarks (e.g., ACDC, Dark Zurich, and Nighttime Driving). Notably, our method achieves state-of-the-art performance with 65.1% mIoU on ACDC-night-test and 55.4% mIoU on ACDC-night-val.
更多查看译文
AI 理解论文
溯源树
样例
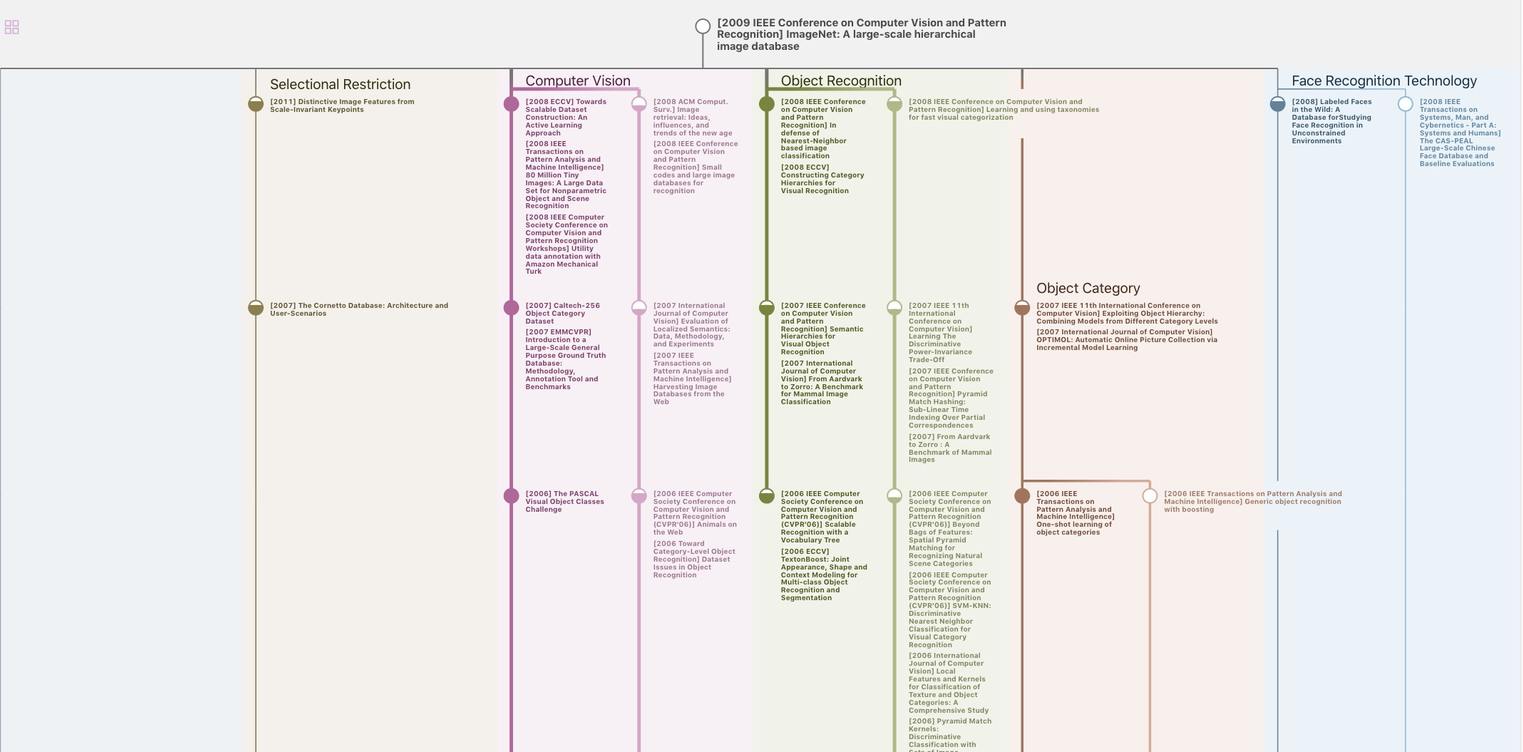
生成溯源树,研究论文发展脉络
Chat Paper
正在生成论文摘要