A Simple Baseline for Open-World Tracking via Self-training
MM '23: Proceedings of the 31st ACM International Conference on Multimedia(2023)
摘要
Open-World Tracking (OWT) presents a challenging yet emerging problem, aiming to track every object of any category. Different from traditional Multi-Object Tracking (MOT), OWT needs to additionally track targets beyond predefined categories in the training set. To address the problem, we propose a simple baseline, SimOWT. We simplify the recently proposed OWT algorithm by streamlining the association module and accelerating the inference speed. By leveraging the self-training paradigm, SimOWT can distinguish unknown-class targets from the background, fully unleashing the potential of TAO-OW dataset. Furthermore, we enhance SimOWT from the perspectives of Pseudo Boxes Merging and Re-Weighting, thereby discovering more targets belonging to unknown classes and reducing the sensitivity of the model to low-quality pseudo-labels. Benefiting from the proposed approaches, SimOWT demonstrates a significant improvement in tracking performance on unknown classes. Moreover, the comprehensive experiments on the TAO-OW benchmark demonstrate that our model outperforms the state-of-the-art OWT method, OWTB, with an absolute gain of 11.2% OWTA and 16.4% detection recall respectively on unknown classes. The code is released at https://github.com/22109095/SimOWT.
更多查看译文
AI 理解论文
溯源树
样例
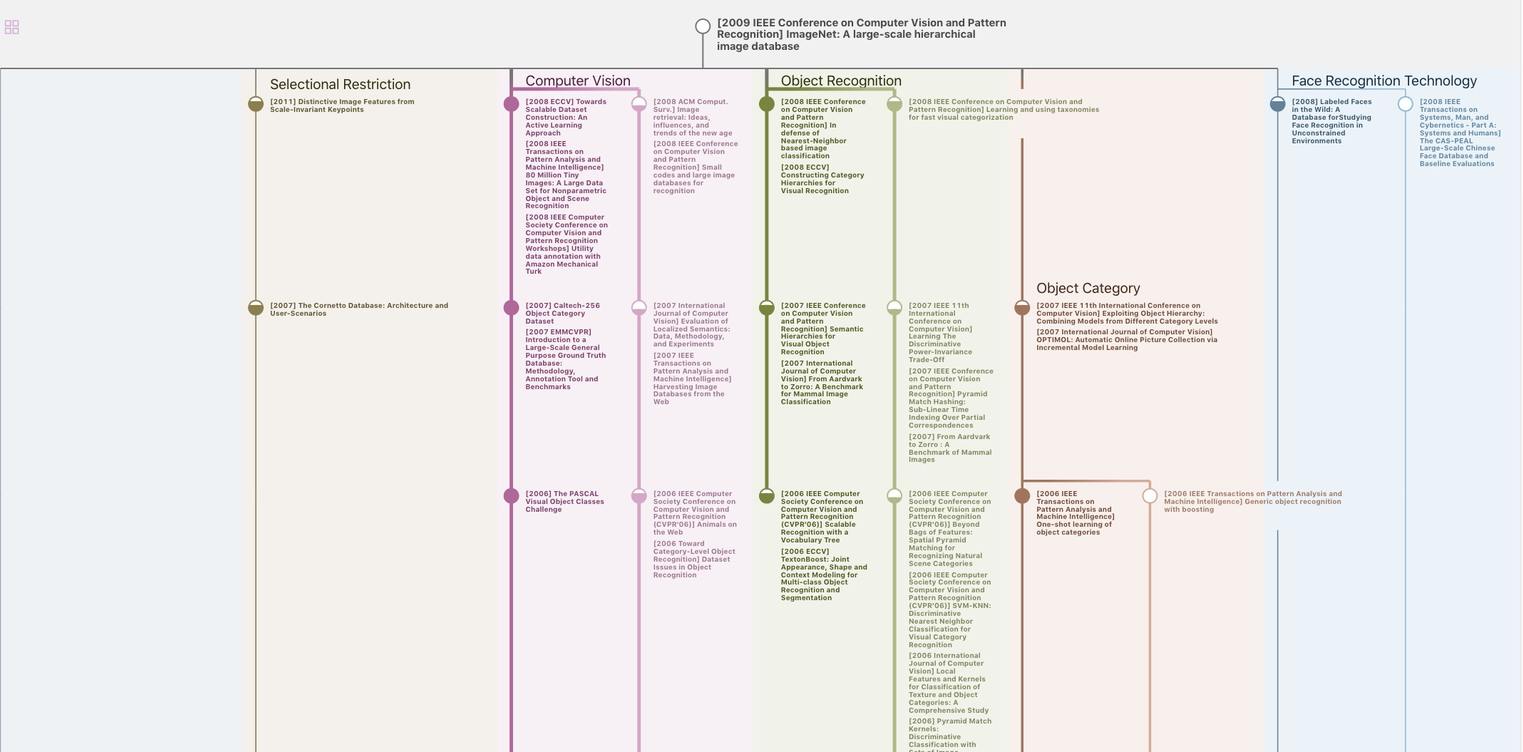
生成溯源树,研究论文发展脉络
Chat Paper
正在生成论文摘要