S-OmniMVS: Incorporating Sphere Geometry into Omnidirectional Stereo Matching
MM '23: Proceedings of the 31st ACM International Conference on Multimedia(2023)
摘要
Multi-fisheye stereo matching is a promising task that employs the traditional multi-view stereo (MVS) pipeline with spherical sweeping to acquire omnidirectional depth. However, the existing omnidirectional MVS technologies neglect fisheye and omnidirectional distortions, yielding inferior performance. In this paper, we revisit omnidirectional MVS by incorporating three sphere geometry priors: spherical projection, spherical continuity, and spherical position. To deal with fisheye distortion, we propose a new distortion-adaptive fusion module to convert fisheye inputs into distortion-free spherical tangent representations by constructing a spherical projection space. Then these multi-scale features are adaptively aggregated with additional learnable offsets to enhance content perception. To handle omnidirectional distortion, we present a new spherical cost aggregation module with a comprehensive consideration of the spherical continuity and position. Concretely, we first design a rotation continuity compensation mechanism to ensure omnidirectional depth consistency of left-right boundaries without introducing extra computation. On the other hand, we encode the geometry-aware spherical position and push them into the cost aggregation to relieve panoramic distortion and perceive the 3D structure. Furthermore, to avoid the excessive concentration of depth hypothesis caused by inverse depth linear sampling, we develop a segmented sampling strategy that combines linear and exponential spaces to create S-OmniMVS, along with three sphere priors. Extensive experiments demonstrate the proposed method outperforms the state-of-the-art (SoTA) solutions by a large margin on various datasets both quantitatively and qualitatively.
更多查看译文
AI 理解论文
溯源树
样例
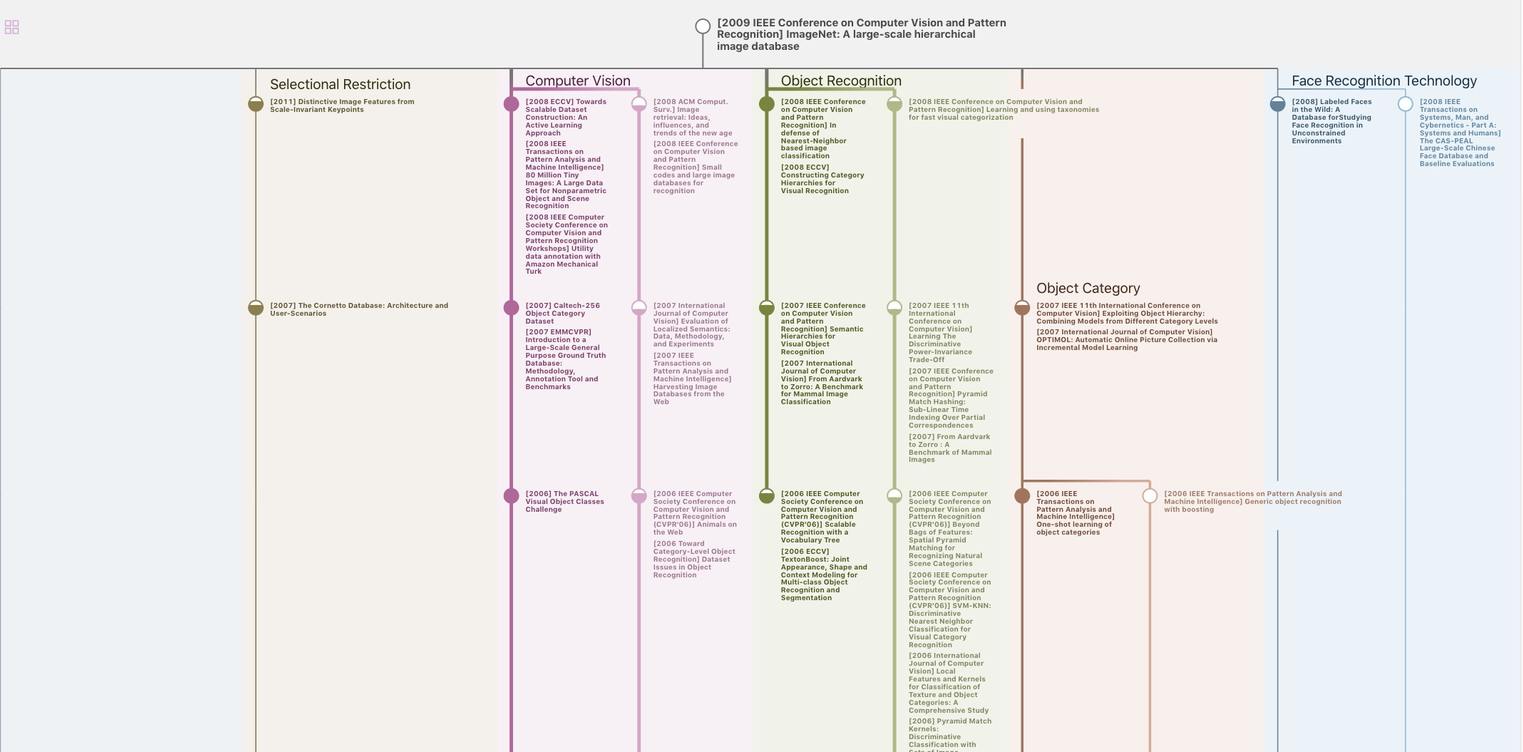
生成溯源树,研究论文发展脉络
Chat Paper
正在生成论文摘要