S3DS: Self-supervised Learning of 3D Skeletons from Single View Images
MM '23: Proceedings of the 31st ACM International Conference on Multimedia(2023)
摘要
3D skeleton is an inherent structure of objects and is often used for shape analysis. However, most supervised deep learning methods, which directly obtain 3D skeletons from 2D images, are constrained by skeleton data preparation. In this paper, we introduce a self-supervised method S3DS: a differentiable rendering-based method to reconstruct a 3D skeleton of shape from its single-view images, by using medial axis transformation (MAT) as its 3D skeleton. We use medial spheres (center positions and radii) to represent the 3D skeleton and use the connectivity of the spheres (medial mesh) to represent the topology. We trained a medial sphere prediction network, which reconstructs 3D skeleton spheres (centers and radii) from a single-view image and renders them into a 2D silhouette with many circles. Because of the radius, the center of the circle will fall on the 2D skeleton. Then the 3D spheres are fitted to the 3D skeleton by fitting many 2D circles onto the 2D skeleton. A mechanism is proposed to generate the connectivity of the discrete medial spheres and construct the 3D topology of the shape. We have conducted extensive experiments on public datasets and proved that S3DS has better performance than baseline and competitive performances with supervised methods on 3D skeletons reconstruction.
更多查看译文
AI 理解论文
溯源树
样例
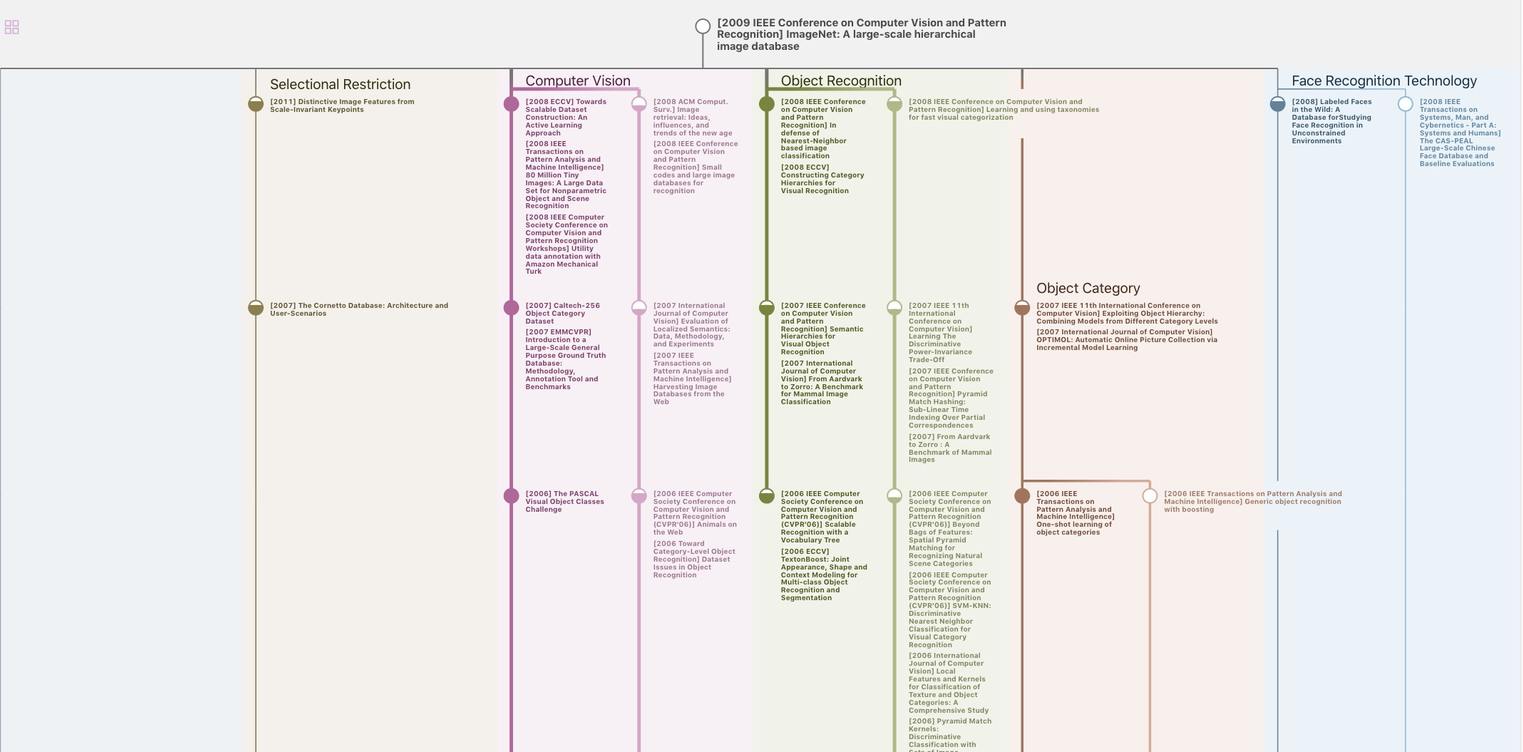
生成溯源树,研究论文发展脉络
Chat Paper
正在生成论文摘要