Pedestrian-specific Bipartite-aware Similarity Learning for Text-based Person Retrieval
MM '23: Proceedings of the 31st ACM International Conference on Multimedia(2023)
摘要
Text-based person retrieval is a challenging task that aims to search pedestrian images with the same identity according to language descriptions. Current methods usually indiscriminately measure the similarity between text and image by matching global visual-textual features and matched local region-word features. However, these methods underestimate the key cue role of mismatched region-word pairs and ignore the problem of low similarity between matched region-word pairs. To alleviate these issues, we propose a novel Pedestrian-specific Bipartite-aware Similarity Learning (PBSL) framework that efficiently reveals the plausible and credible levels of contribution of pedestrian-specific mismatched and matched region-word pairs towards overall similarity. Specifically, to focus on mismatched region-word pairs, we first develop a new co-interactive attention that utilizes cross-modal information to guide the extraction of pedestrian-specific information in a single modality. We then design a negative similarity regularization mechanism to use the negative similarity score as a bias to correct the overall similarity. Additionally, to enhance the contribution of matched region-word pairs, we introduce graph networks to aggregate and propagate local information of pedestrian-specific, using overall visual-textual similarity to evaluate locally matched region-word pairs for weight refinement. Finally, extensive experiments are conducted on the CUHK-PEDES, ICFG-PEDES, and RSTPReid datasets to demonstrate the competitive performance of the proposed PBSL in the text-based person retrieval task.
更多查看译文
AI 理解论文
溯源树
样例
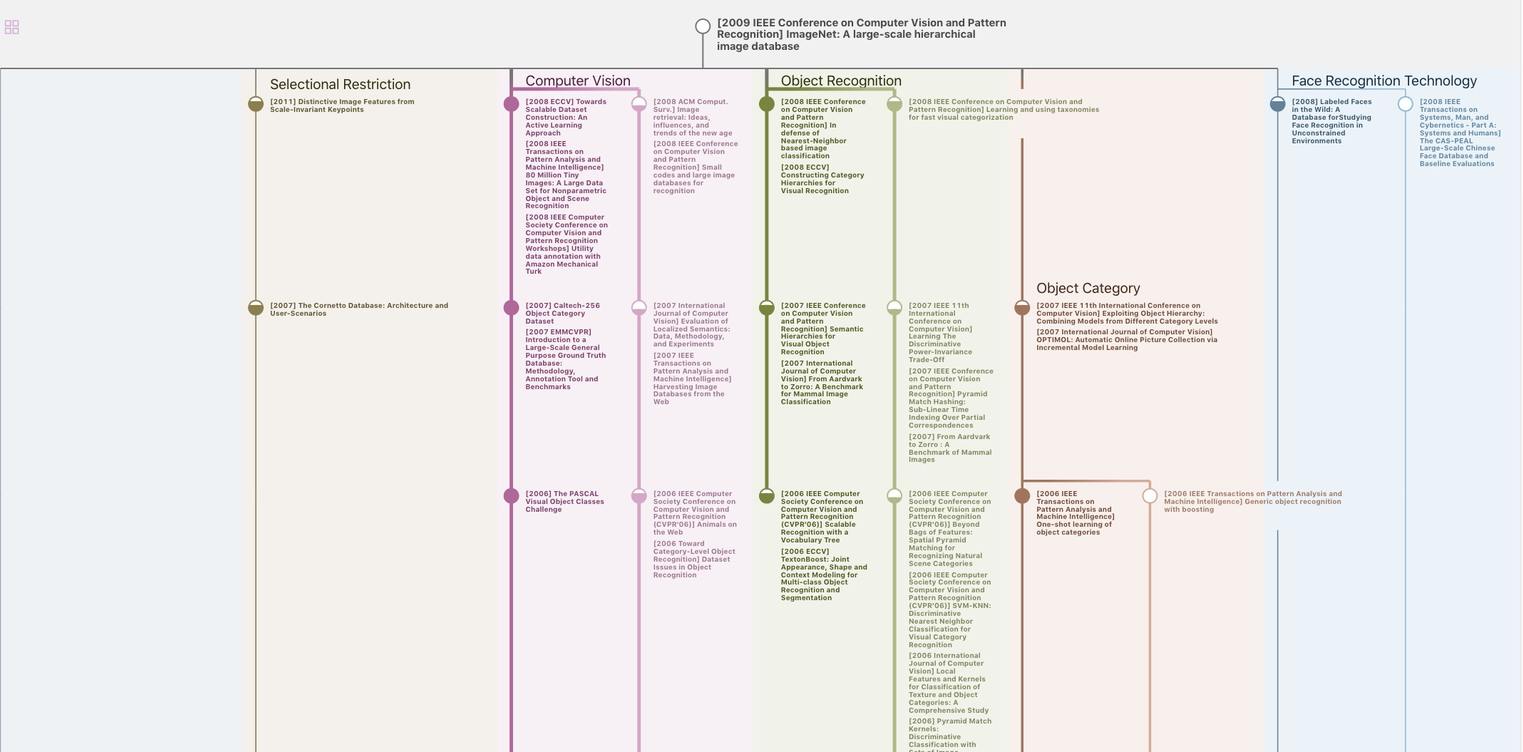
生成溯源树,研究论文发展脉络
Chat Paper
正在生成论文摘要