mSIRM: Cost-Efficient and SLO-aware ML Load Balancing on Fog and Multi-Cloud Network
PROCEEDINGS OF THE 13TH WORKSHOP ON AI AND SCIENTIFIC COMPUTING AT SCALE USING FLEXIBLE COMPUTING INFRASTRUCTURES, FLEXSCIENCE 2023(2023)
摘要
The use of intelligent sensors and edge devices has grown exponentially for automation in the industry to hyper-personalize applications, minimize cost, improve efficiency, and optimize operations. In a typical Internet-of-Thing (IoT) workflow, pre-trained machine-learning (ML) models are placed on edge devices. These devices have limited resources to serve the incoming load and hence judicious capacity planning is required to address future workloads. This generally results in the over-provisioning or under-provisioning of the resources. Cloud offers a flexible infrastructure for unpredictable workloads faced by on-premise instances but at the cost of increased latency. Fog devices and spatially closer-to-edge devices act as intermediaries to cloud resources with lower latency, however, suffer from capacity planning challenges. These challenges can be mitigated by the use of appropriate cloud services and resource configuration. In this work, we present a Multi-cloud System for Inference Request Management (mSIRM) for the edge-fog-cloud continuum. mSIRM has capabilities for serving dynamically varying machine learning inference workloads using flexible infrastructure across different cloud vendors. Furthermore, we show that the use of multiple clouds with different services in conjunction with the fog computing resources results in a significant drop in Service Level Objective (SLO) violations. Specifically, we compare the edge-cloud frameworks developed using machine learning and serverless platforms from popular cloud service providers.
更多查看译文
关键词
MLaaS,inference serving,SLO awareness,cost minimization
AI 理解论文
溯源树
样例
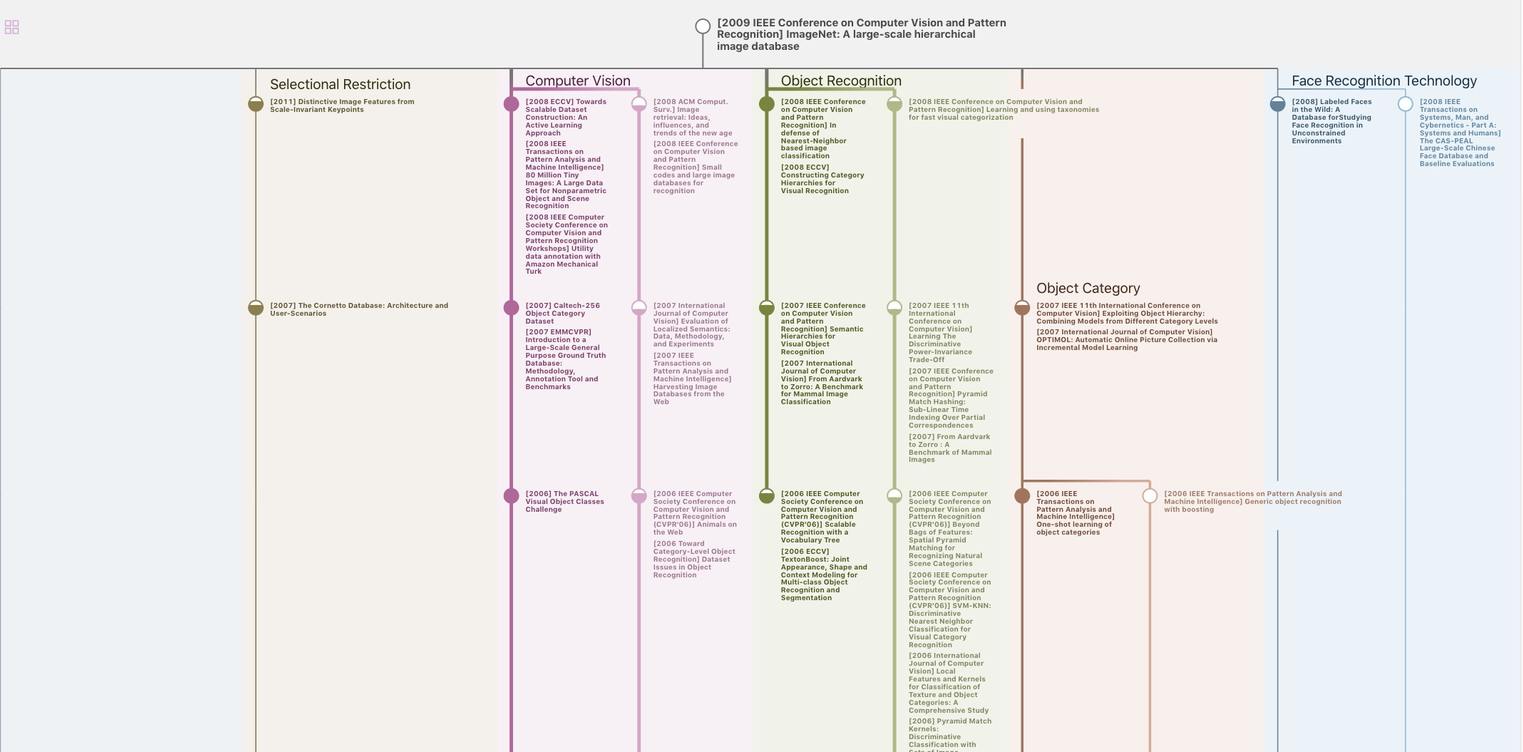
生成溯源树,研究论文发展脉络
Chat Paper
正在生成论文摘要