Automatic Generation of Distributed-Memory Mappings for Tensor Computations
SC '23: Proceedings of the International Conference for High Performance Computing, Networking, Storage and Analysis(2023)
摘要
While considerable research has been directed at automatic parallelization for shared-memory platforms, little progress has been made in automatic parallelization schemes for distributed-memory systems. We introduce an innovative approach to automatically produce distributed-memory parallel code for an important subclass of affine tensor computations common to Coupled Cluster (CC) electronic structure methods, neuro-imaging applications, and deep learning models. We propose a novel systematic approach to modeling the relations and trade-offs of mapping computations and data onto multidimensional grids of homogeneous nodes. Our formulation explores the space of computation and data distributions across processor grids. Tensor programs are modeled as a non-linear symbolic formulation accounting for the volume of data communication and per-node capacity constraints induced under specific mappings. Solutions are found, iteratively, using the Z3 SMT solver, and used to automatically generate efficient MPI code. Our evaluation demonstrates the effectiveness of our approach over Distributed-Memory Pluto and the Cyclops Tensor Framework.
更多查看译文
关键词
Automatic Generation,Tensor Calculation,Parallelization,Nonlinear Form,Coding Efficiency,Communication Constraints,Dimensional Space,Memory Capacity,Field Of Practice,Data Space,Processing Elements,Input Matrix,Null Space,Replication Data,Directed Acyclic Graph,Large Input,Local Computing,Code Generation,Data Placement,Small Kernel,Number Of Processors,2D Grid,Tile Size,Tensor Operations,Output Tensor,Parallel Algorithm,Communication Cost,Distributed Algorithm
AI 理解论文
溯源树
样例
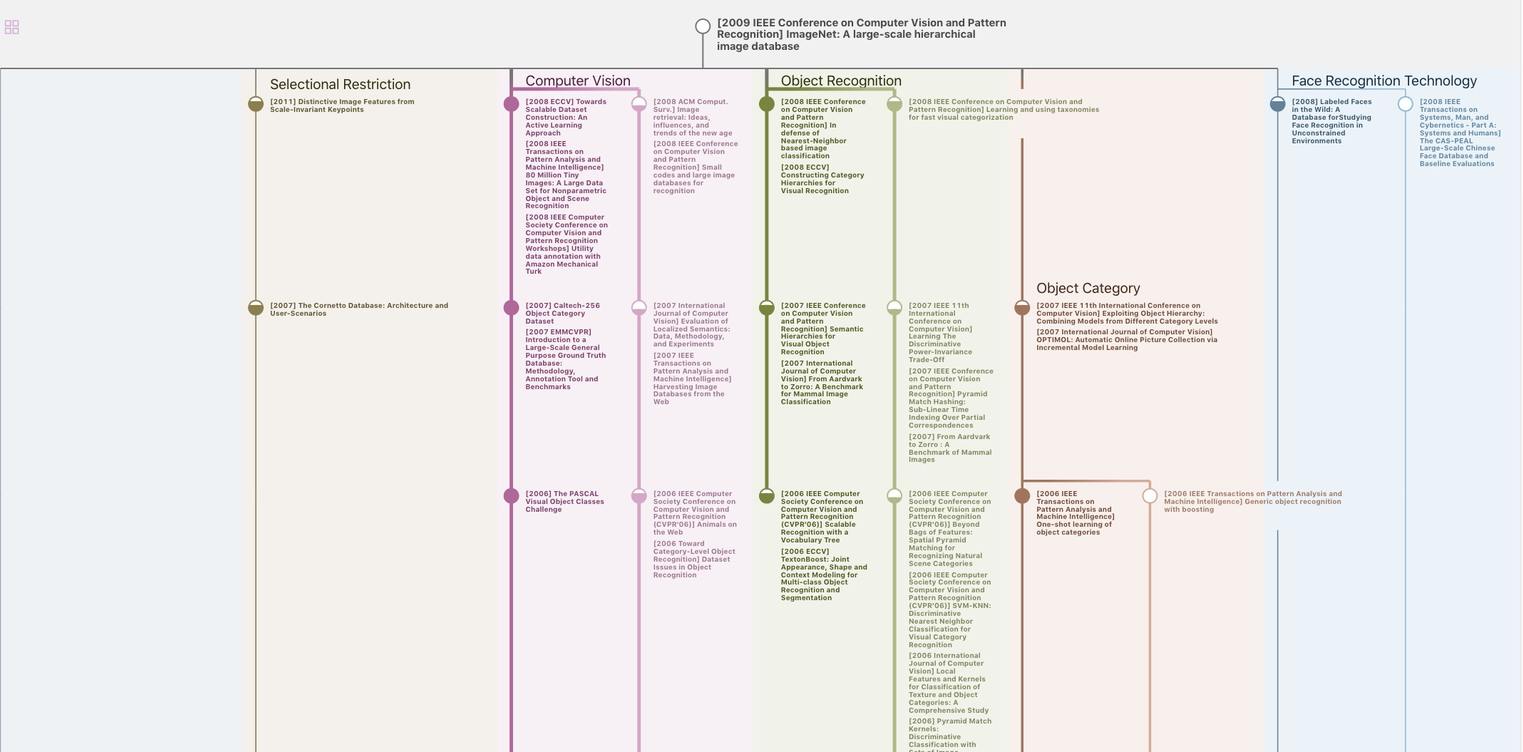
生成溯源树,研究论文发展脉络
Chat Paper
正在生成论文摘要