AdaptiveSound: An Interactive Feedback-Loop System to Improve Sound Recognition for Deaf and Hard of Hearing Users
PROCEEDINGS OF THE 25TH INTERNATIONAL ACM SIGACCESS CONFERENCE ON COMPUTERS AND ACCESSIBILITY, ASSETS 2023(2023)
摘要
Sound recognition tools have wide-ranging impacts for deaf and hard of hearing (DHH) people from being informed of safety-critical information (e.g., fire alarms, sirens) to more mundane but still useful information (e.g., door knock, microwave beeps). However, prior sound recognition systems use models that are pre-trained on generic sound datasets and do not adapt well to diverse variations of real-world sounds. We introduce AdaptiveSound, a real-time system for portable devices (e.g., smartphones) that allows DHH users to provide corrective feedback to the sound recognition model to adapt the model to diverse acoustic environments. AdaptiveSound is informed by prior surveys of sound recognition systems, where DHH users strongly desired the ability to provide feedback to a pre-trained sound recognition model to fine-tune it to their environments. Through quantitative experiments and field evaluations with 12 DHH users, we show that AdaptiveSound can achieve a significantly higher accuracy (+14.6%) than prior state-of-the art systems in diverse real-world locations (e.g., homes, parks, streets, and malls) with little end-user effort (about 10 minutes of feedback).
更多查看译文
关键词
Accessibility,deaf,Deaf,hard of hearing,sound awareness,audio event detection,applied machine learning,reinforcement learning,incremental learning,human-in-the-loop,Human-AI
AI 理解论文
溯源树
样例
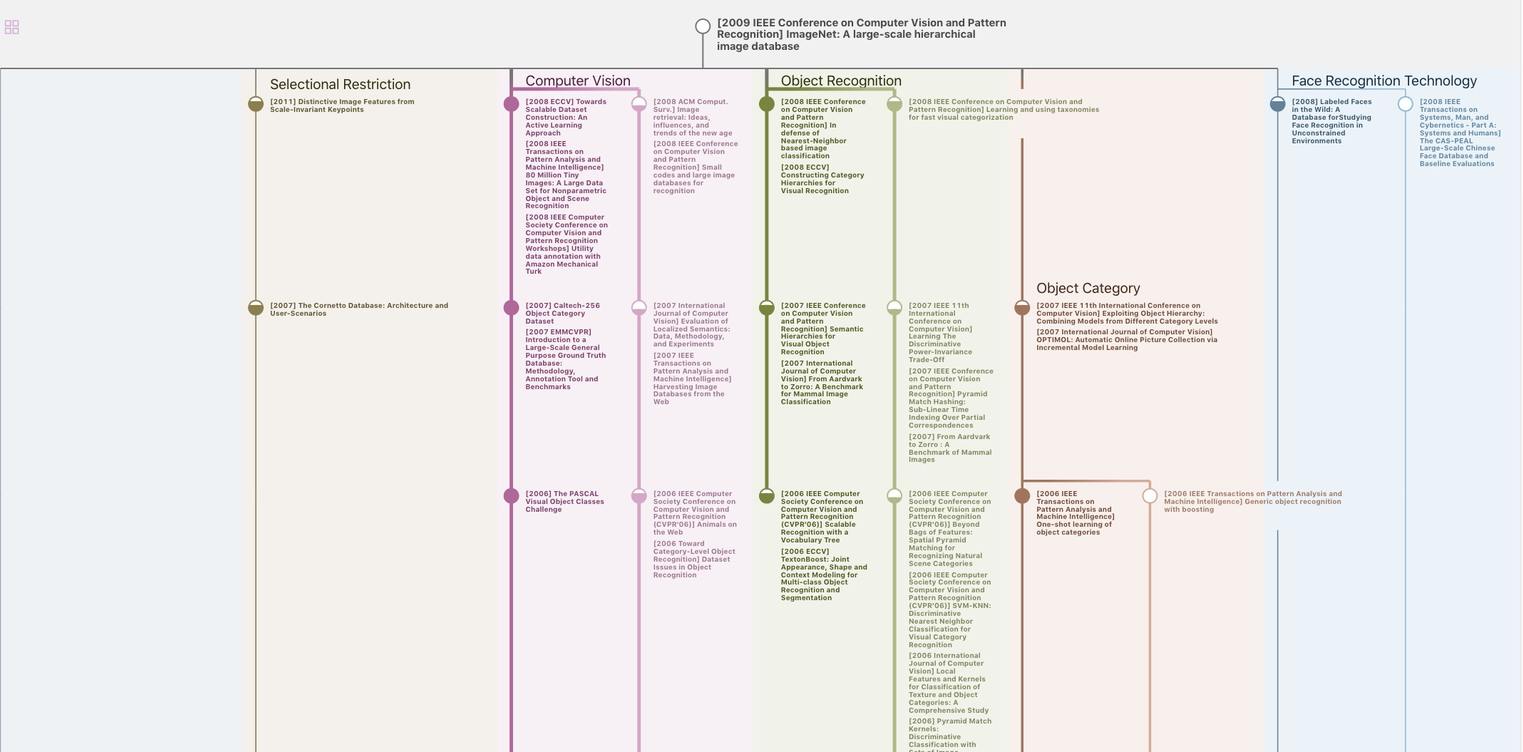
生成溯源树,研究论文发展脉络
Chat Paper
正在生成论文摘要