Characterizing Reliability of Three-version Traffic Sign Classifier System through Diversity Metrics
2023 IEEE 34th International Symposium on Software Reliability Engineering (ISSRE)(2023)
摘要
The N-version machine learning (ML) system is an architecture approach to enhance the reliability of ML system outputs by exploiting ML model diversity and input data diversity. While existing studies theoretically show the relation between diversity metrics and system reliability, there is a shortage of empirical studies validating reliability models with diversity parameters in real datasets. In this paper, focusing on traffic sign recognition tasks, we empirically analyze the impact of diversity parameter estimations for predicting the reliability of three-version traffic sign classifier systems. Using five real-world traffic sign datasets, we confirm that the three-version architecture effectively enhances system reliability by applying diverse models and diversified input images. Then, we estimate the diversity parameters and apply them to variants of reliability prediction models. The prediction residuals between the observed reliability and the predicted reliability are mostly less than 0.017 across all data sets, which is half of the residual achieved by the conventional prediction model, except for the architecture of a single model with triple input. As the estimated values of diversity parameters tend to be stable with a relatively small number of samples, we consider that the reliability prediction models using diversity parameters are useful in the early-stage design of ML systems.
更多查看译文
关键词
Classification,Diversity,Machine learning system,N-version programming,Reliability
AI 理解论文
溯源树
样例
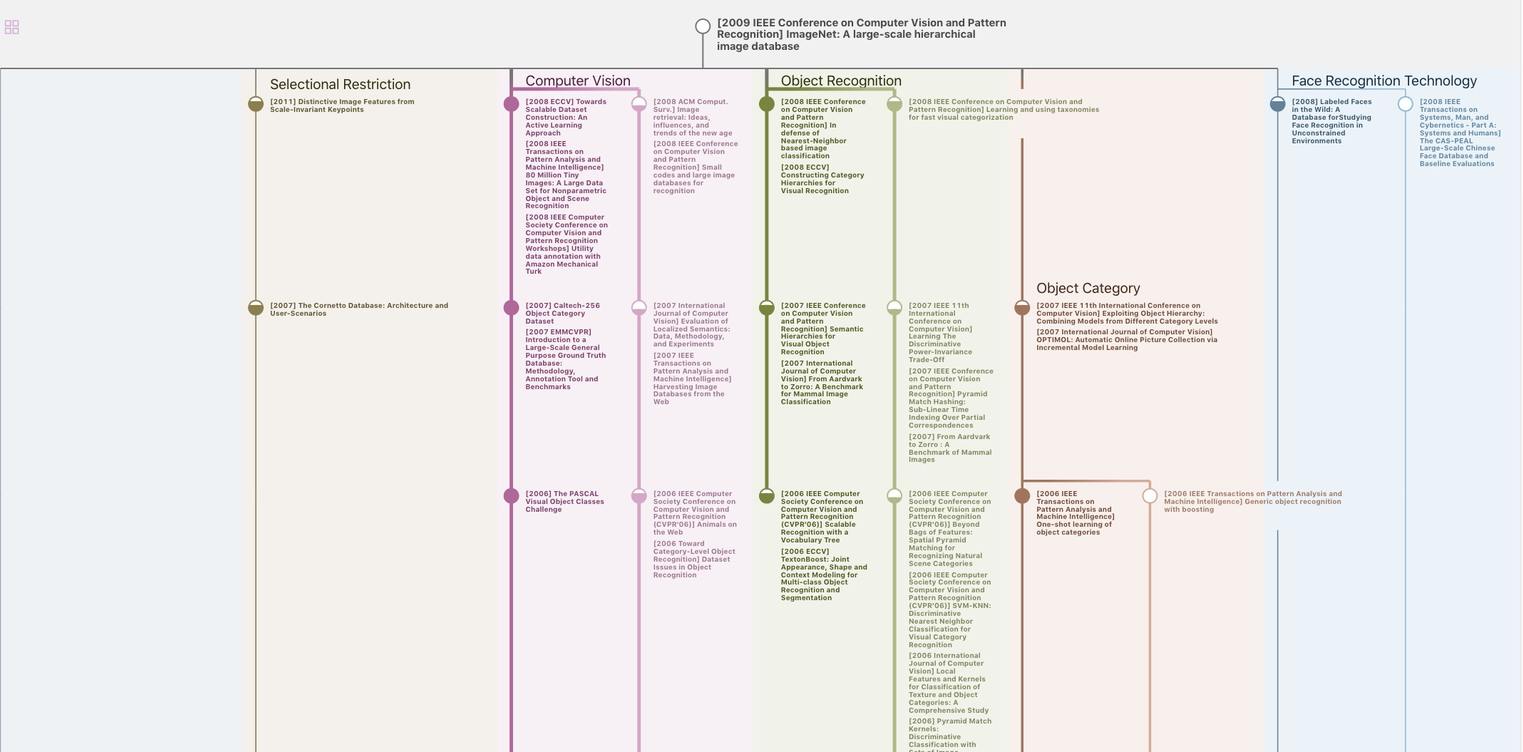
生成溯源树,研究论文发展脉络
Chat Paper
正在生成论文摘要