Handover Forecasting in Cellular Networks: A Spatio-temporal Graph Neural Network Approach
2023 14th International Conference on Network of the Future (NoF)(2023)
摘要
The proliferation of connected devices, as well as the continuous integration of data demanding applications, have brought significant challenges to mobile network operators. Indeed, mobile networks need to accommodate the resulting exponential growth of traffic, while still ensuring a decent Quality of Service (QoS) and Quality of Experience (QoE) to their users. To do so, adequate management solutions are needed. So far, a variety of problems have been tackled, including resource allocation and network planning. Yet, handovers (HO) management has not received much attention, despite its importance for users QoS and QoE. In this work, we study the problem of mobile devices HO forecasting, over time, among base stations. To solve the problem, we introduce a Handover Graph Convolutional Long Short-Term Memory (HGC-LSTM) neural network forecasting approach. Our approach allows capturing spatio-temporal correlations among neighboring pairs of base stations in the forecasting process. The evaluation on a real-world dataset shows that the proposed HGC-LSTM approach outperforms other methods in the state-of-art in terms of accuracy and execution time.
更多查看译文
关键词
Handovers Forecasting,5G and beyond,Time Series Forecasting,Machine Learning
AI 理解论文
溯源树
样例
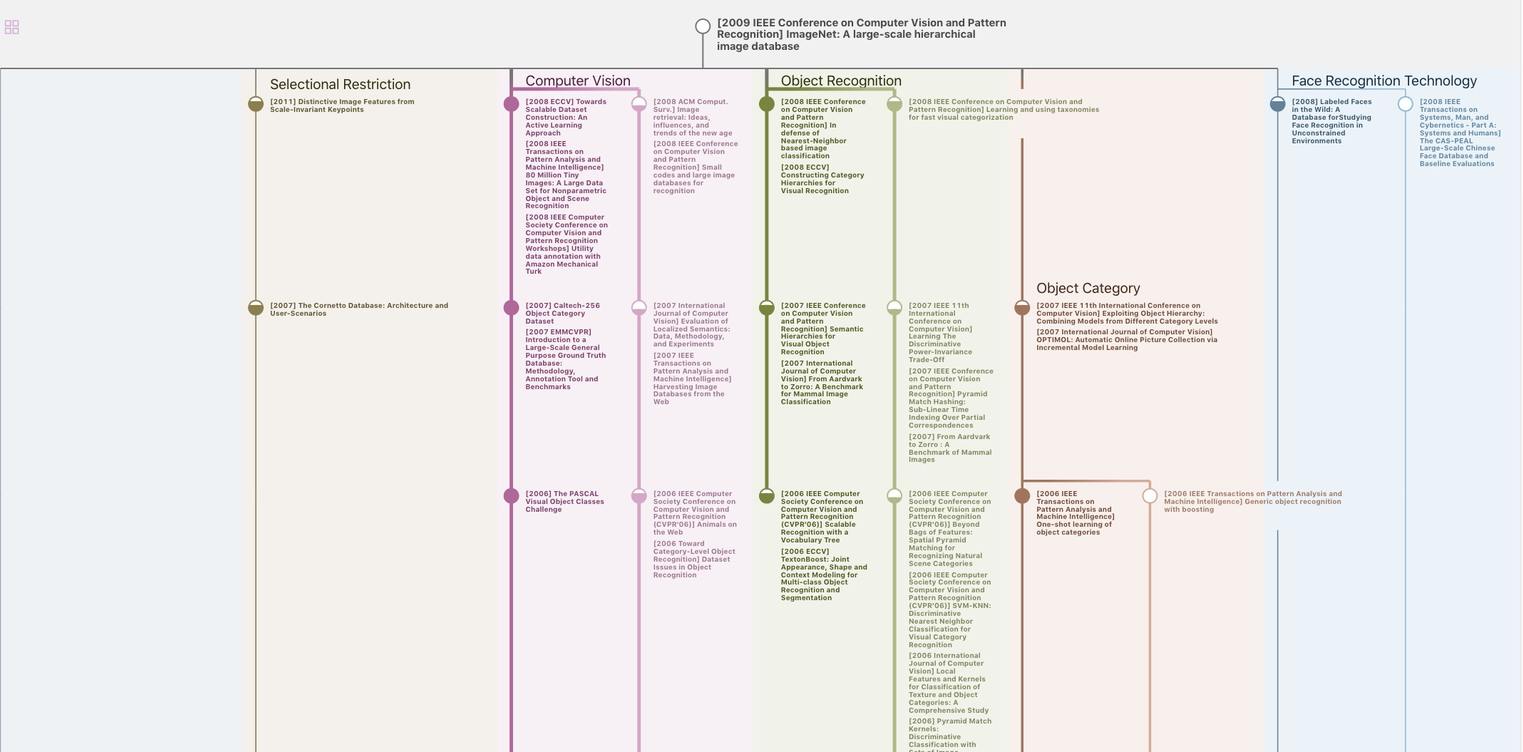
生成溯源树,研究论文发展脉络
Chat Paper
正在生成论文摘要