Data Imputation with an Autoencoder and MAGIC
2023 International Conference on Sampling Theory and Applications (SampTA)(2023)
摘要
Missing data is a common problem in many applications. Imputing missing values is a challenging task, as the imputations need to be accurate and robust to avoid introducing bias in downstream analysis. In this paper, we propose an ensemble method that combines the strengths of a manifold learning-based imputation method called MAGIC and an autoencoder deep learning model. We call our method Deep MAGIC. Deep MAGIC is trained on a linear combination of the mean squared error of the original data and the mean squared error of the MAGIC-imputed data. Experimental results on three benchmark datasets show that Deep MAGIC outperforms several state-of-the-art imputation methods, demonstrating its effectiveness and robustness in handling large amounts of missing data.
更多查看译文
AI 理解论文
溯源树
样例
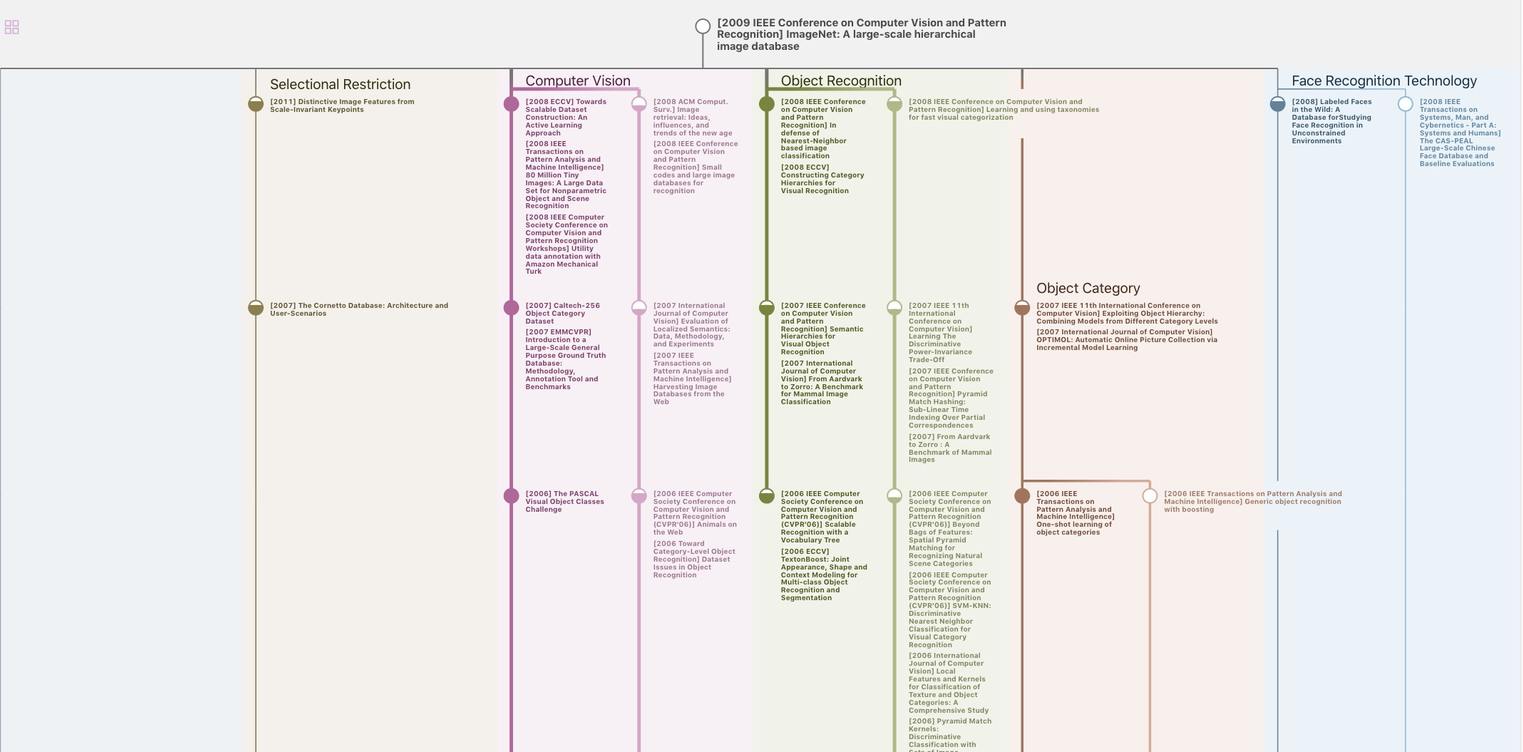
生成溯源树,研究论文发展脉络
Chat Paper
正在生成论文摘要