DeNoL: A Few-Shot-Sample-Based Decoupling Noise Layer for Cross-channel Watermarking Robustness
MM '23: Proceedings of the 31st ACM International Conference on Multimedia(2023)
摘要
Cross-channel (e.g. Screen-to-Camera) robustness is an urgent requirement for modern watermarking systems. To realize such robustness, training a network that can precisely simulate the cross-channel distortion as the noise layer for deep watermarking training is an effective way. However, network training requires massive data, and generating the data is laborious. Meanwhile, directly using limited data to train may lead to an over-fitting issue. To address such limitation, we proposed DeNoL, a decoupling noise layer for cross-channel simulation which only needs few-shot samples. We believe the overfitting issue comes from the overlearning of the training image content rather than only simulating the distortion style. Consequently, we design a network that can decouple the image content and the distortion style into different components. Thus, by fixing the content representation component and fine-tuning a new style component accordingly, the network can efficiently learn and only learn the distortion style. Such learning can be done with only few-shot samples. Besides, in order to enhance adaptability, we also proposed a diversification operation to cooperate with DeNoL. Experimental results show that DeNoL can effectively simulate cross-channel distortion with only 20 image pairs and assist in training a general and robust watermarking network.
更多查看译文
AI 理解论文
溯源树
样例
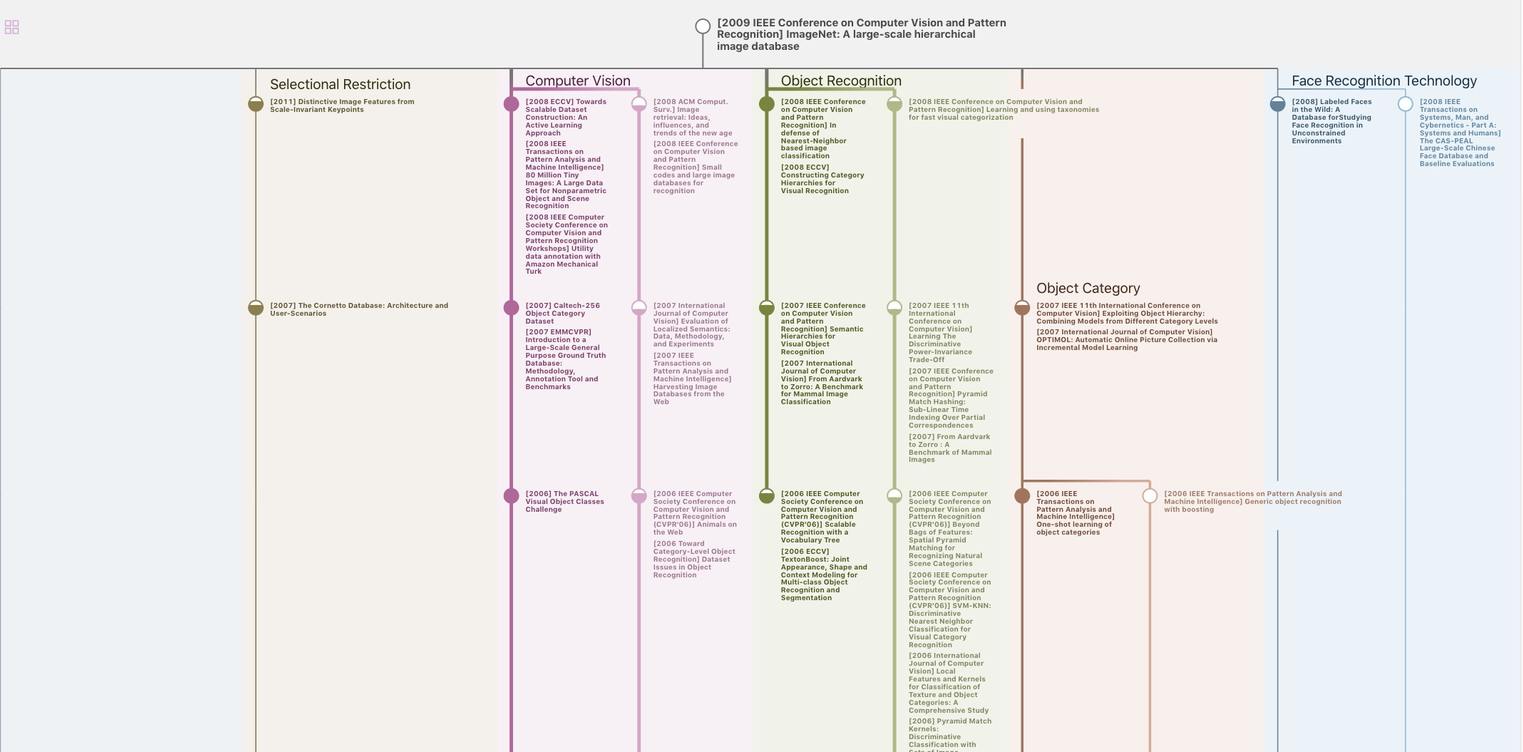
生成溯源树,研究论文发展脉络
Chat Paper
正在生成论文摘要